FedNI: Federated Graph Learning With Network Inpainting for Population-Based Disease Prediction
IEEE transactions on medical imaging(2023)
摘要
Graph Convolutional Neural Networks (GCNs) are widely used for graph analysis. Specifically, in medical applications, GCNs can be used for disease prediction on a population graph, where graph nodes represent individuals and edges represent individual similarities. However, GCNs rely on a vast amount of data, which is challenging to collect for a single medical institution. In addition, a critical challenge that most medical institutions continue to face is addressing disease prediction in isolation with incomplete data information. To address these issues, Federated Learning (FL) allows isolated local institutions to collaboratively train a global model without data sharing. In this work, we propose a framework, FedNI, to leverage network inpainting and inter-institutional data via FL. Specifically, we first federatively train missing node and edge predictor using a graph generative adversarial network (GAN) to complete the missing information of local networks. Then we train a global GCN node classifier across institutions using a federated graph learning platform. The novel design enables us to build more accurate machine learning models by leveraging federated learning and also graph learning approaches. We demonstrate that our federated model outperforms local and baseline FL methods with significant margins on two public neuroimaging datasets.
更多查看译文
关键词
Federated learning,graph convolutional networks,population network,disease prediction
AI 理解论文
溯源树
样例
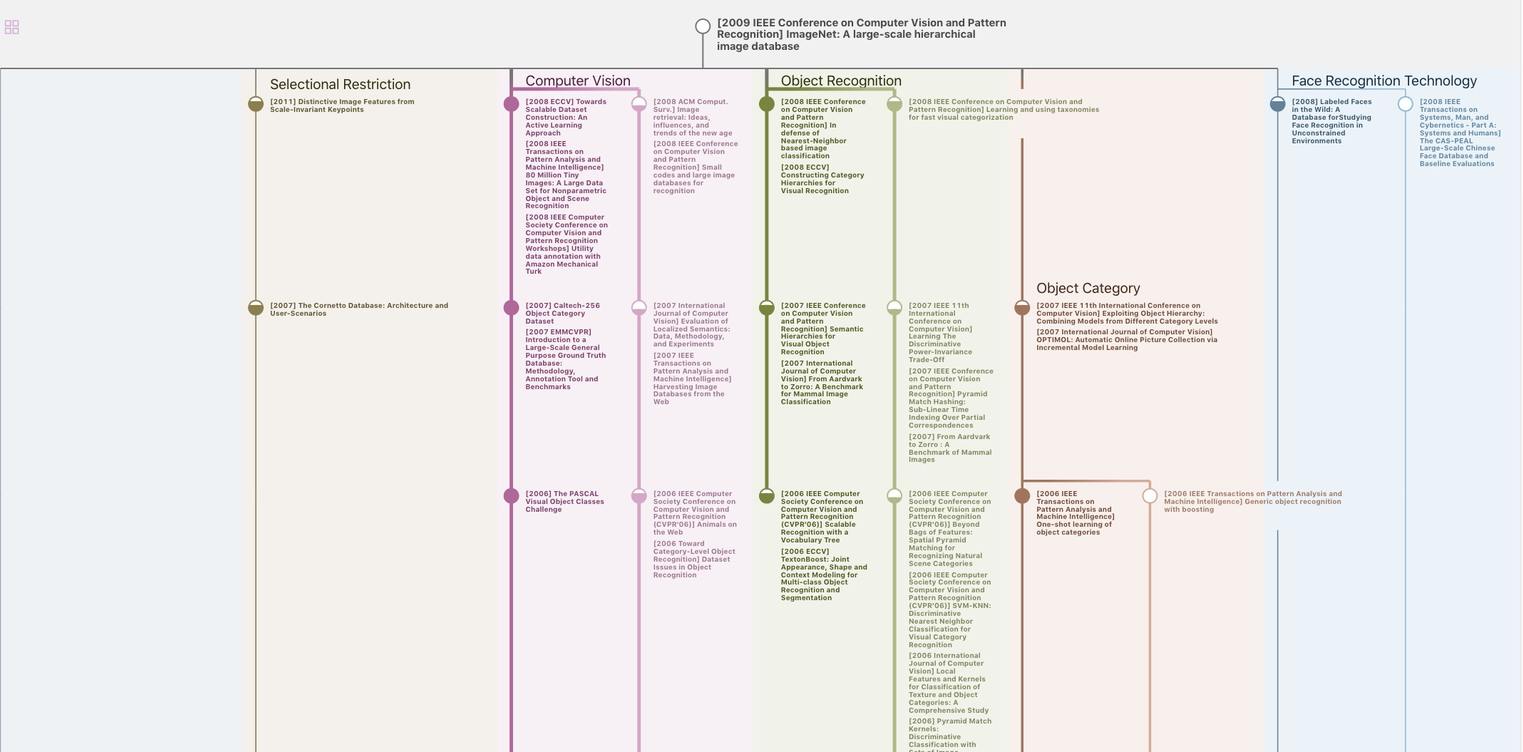
生成溯源树,研究论文发展脉络
Chat Paper
正在生成论文摘要