DeepUME: Learning the Universal Manifold Embedding for Robust Point Cloud Registration.
British Machine Vision Conference(2021)
摘要
Registration of point clouds related by rigid transformations is one of the fundamental problems in computer vision. However, a solution to the practical scenario of aligning sparsely and differently sampled observations in the presence of noise is still lacking. We approach registration in this scenario with a fusion of the closed-form Universal Mani-fold Embedding (UME) method and a deep neural network. The two are combined into a single unified framework, named DeepUME, trained end-to-end and in an unsupervised manner. To successfully provide a global solution in the presence of large transformations, we employ an SO(3)-invariant coordinate system to learn both a joint-resampling strategy of the point clouds and SO(3)-invariant features. These features are then utilized by the geometric UME method for transformation estimation. The parameters of DeepUME are optimized using a metric designed to overcome an ambiguity problem emerging in the registration of symmetric shapes, when noisy scenarios are considered. We show that our hybrid method outperforms state-of-the-art registration methods in various scenarios, and generalizes well to unseen data sets. Our code is publicly available.
更多查看译文
关键词
robust point
AI 理解论文
溯源树
样例
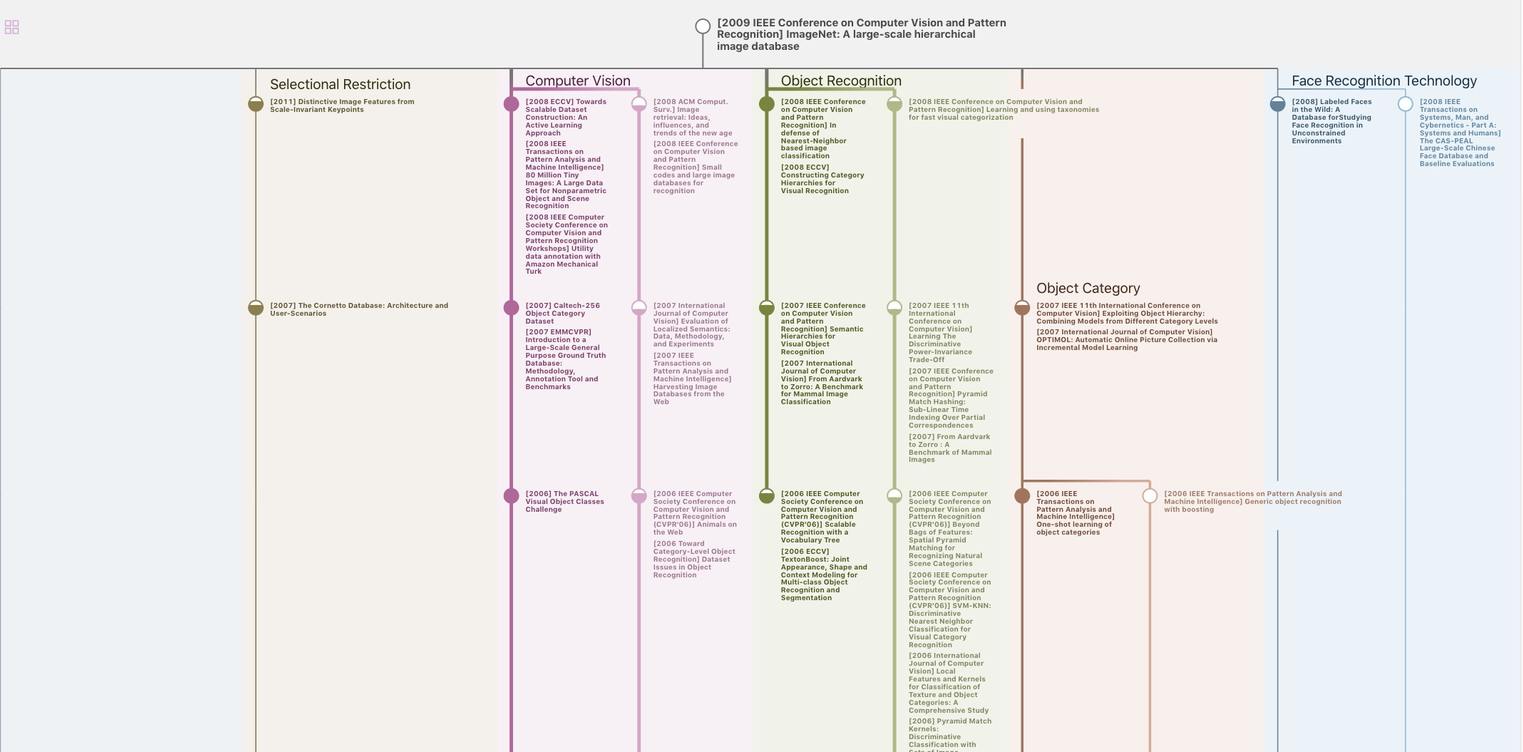
生成溯源树,研究论文发展脉络
Chat Paper
正在生成论文摘要