Multiscale Visual-Attribute Co-Attention for Zero-Shot Image Recognition
IEEE transactions on neural networks and learning systems(2023)
摘要
Zero-shot image recognition aims to classify data from unseen classes, by exploring the association between visual features and the semantic representations of each class. Most existing approaches focus on learning a shared single-scale embedding space (often at the output layer of the network) for both visual and semantic features, ignoring a fact that different-scale visual features exhibit different semantics. In this article, we propose a multi-scale visual-attribute co-attention (mVACA) model, considering both visual-semantic alignment and visual discrimination at multiple scales. At each scale, a hybrid visual attention is realized by attribute-related attention and visual self-attention. The attribute-related attention is guided by a pseudo attribute vector inferred via a mutual information regularization (MIR). The visual self-attentive features further influence the attribute attention to emphasize visual-associated attributes. Leveraging multiscale visual discrimination, mVACA unifies standard zero-shot learning (ZSL) and generalized ZSL tasks in one framework, achieving state-of-the-art or competitive performance on several commonly used benchmarks of both setups. To better understand the interaction between images and attributes in mVACA, we also provide visualized analysis.
更多查看译文
关键词
Image classification,multiscale attention,mutual information regularization (MIR),visual-attribute co-attention,zero-shot learning (ZSL)
AI 理解论文
溯源树
样例
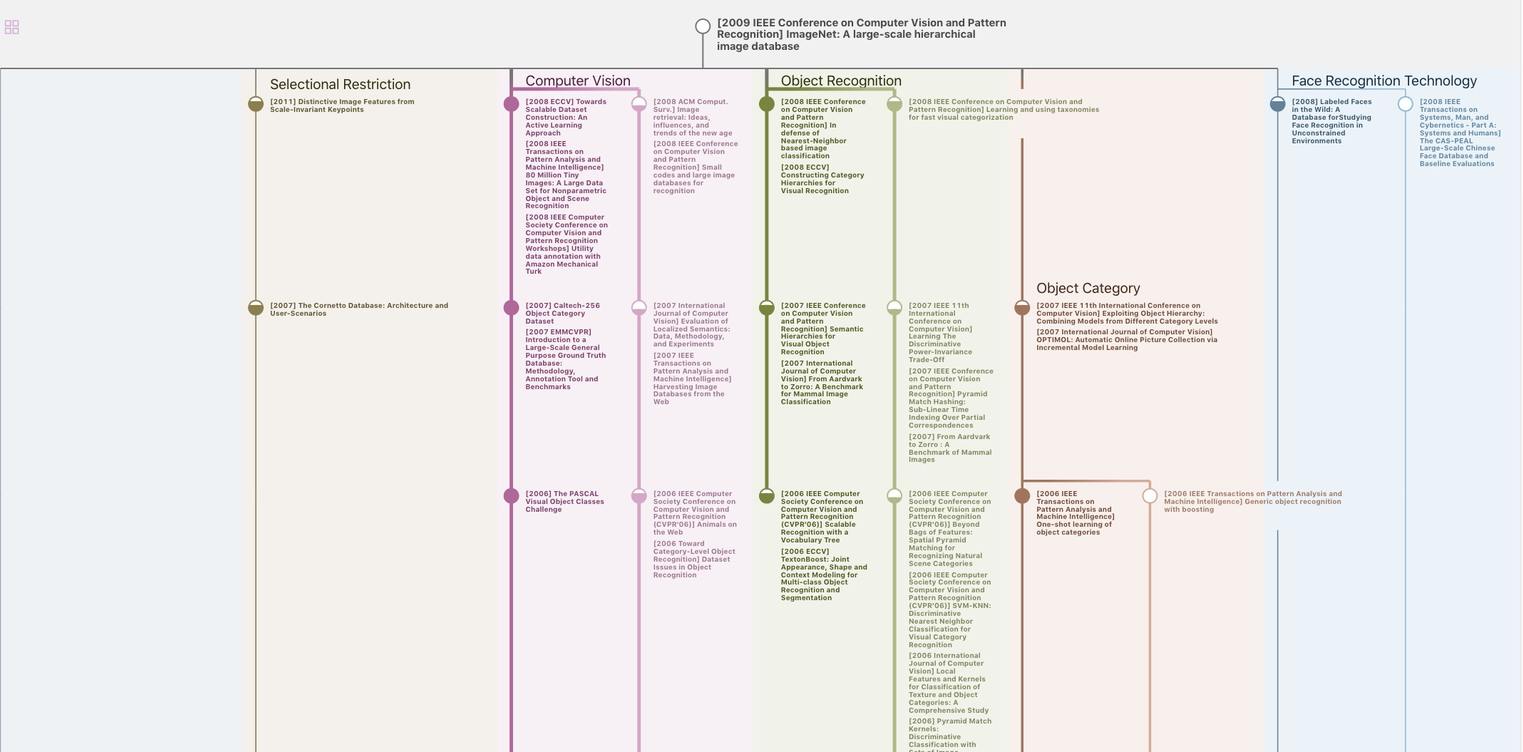
生成溯源树,研究论文发展脉络
Chat Paper
正在生成论文摘要