A Hybrid Seasonal Autoregressive Integrated Moving Average and Denoising Autoencoder Model for Atmospheric Temperature Profile Prediction.
Big Data(2022)
摘要
Atmospheric parameter profile plays a vital role in the studies on meteorology and quantitative remote sensing. Besides measurement of the radiosonde and satellite remote sensing, the atmospheric temperature profile can be predicted by the time series model. However, the performance of the time series model is limited by the weak correlation of temperature data in the stratosphere. In this study, to predict monthly mean atmospheric temperature profile, a hybrid model named seasonal autoregressive integrated moving average and denoising autoencoder (SARIMA-DAE), which combines the traditional time series model with an artificial neural network (ANN), is proposed. The SARIMA is first used to predict the temperature at each altitude level independently; then, the DAE is employed to denoise the predicted temperature profile by SARIMA. The data set used is the L3 monthly mean gridded product of atmospheric infrared sounder, an instrument aboard NASA's Aqua satellite. Taking Maoming, a city in China, as an example, the absolute errors are within 1 K. The mean squared error and mean absolute percentage error on the test set are 0.12 and 0.0012, respectively. Compared with SARIMA, support vector machine regression, and ANN models, experimental results show that the SARIMA-DAE model improves the prediction accuracy at almost all altitude levels. Especially in the stratosphere, the advantage of the hybrid model is more pronounced. The model we proposed should, therefore, be of value to estimate missing data and predict atmospheric parameter profiles.
更多查看译文
关键词
DAE,SARIMA,atmospheric profile prediction,time series
AI 理解论文
溯源树
样例
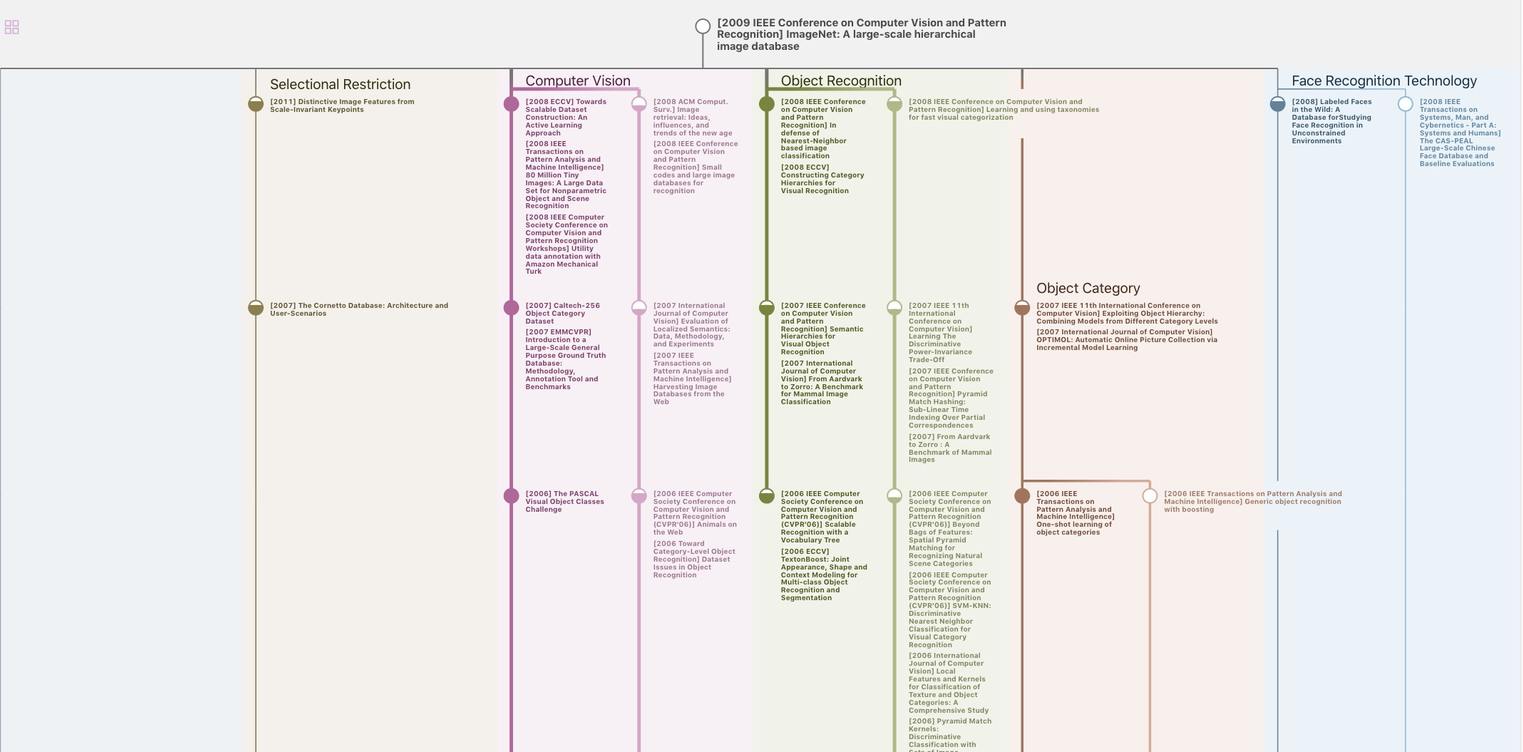
生成溯源树,研究论文发展脉络
Chat Paper
正在生成论文摘要