Practical Quantum K-Means Clustering: Performance Analysis and Applications in Energy Grid Classification
IEEE Transactions on Quantum Engineering(2022)
摘要
In this work, we aim to solve a practical use-case of unsupervised clustering that has applications in predictive maintenance in the energy operations sector using quantum computers. Using only cloud access to quantum computers, we complete thorough performance analysis of what some current quantum computing systems are capable of for practical applications involving nontrivial mid-to-high-dimensional datasets. We first benchmark how well distance estimation can be performed using two different metrics based on the swap-test, using angle and amplitude data embedding. Next, for the clustering performance analysis, we generate sets of synthetic data with varying cluster variance and compare simulation to physical hardware results using the two metrics. From the results of this performance analysis, we propose a general, competitive, and parallelized version of quantum
$k$
-means clustering to avoid some pitfalls discovered due to noisy hardware and apply the approach to a real energy grid clustering scenario. Using real-world German electricity grid data, we show that the new approach improves the balanced accuracy of the standard quantum
$k$
-means clustering by 67.8% with respect to the labeling of the classical algorithm.
更多查看译文
关键词
Cloud quantum computing,quantum clustering,quantum computing,quantum distance estimation
AI 理解论文
溯源树
样例
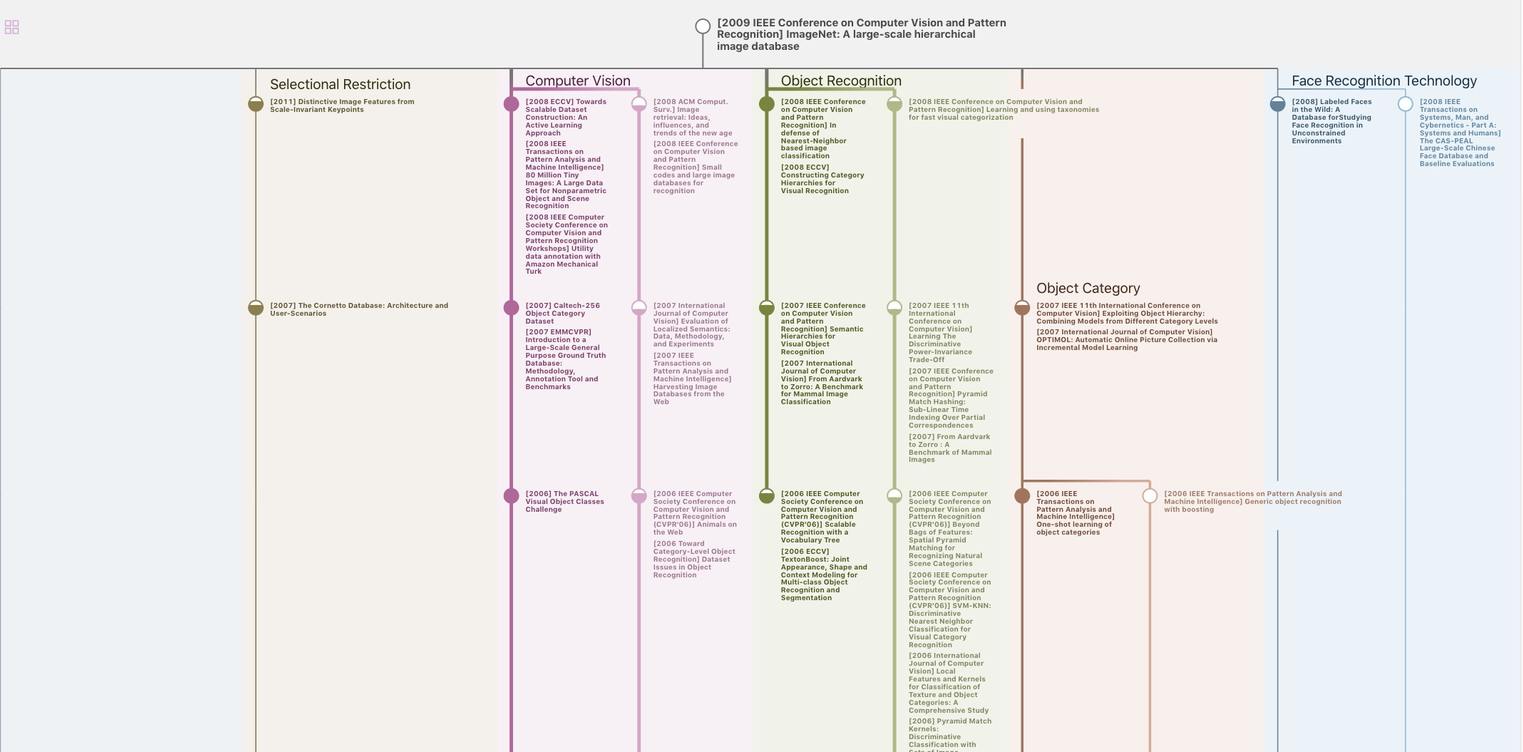
生成溯源树,研究论文发展脉络
Chat Paper
正在生成论文摘要