Recurrent Sparse MIMO Detection Network Based on Modified Projected Gradient Descent Method
2021 IEEE 94TH VEHICULAR TECHNOLOGY CONFERENCE (VTC2021-FALL)(2021)
摘要
Deep learning (DL) has emerged as a powerful tool for signal detection in large-scale multiple-input multiple-output (MIMO) systems. In this paper, the recurrent sparse detection network (RS-Net) is proposed for performance improvement and complexity reduction. First of all, in order to reduce complexity, RS-Net unfolds the projected gradient descent (PGD) method in a modified way, which consists of a projection and a gradient descent (GD) part. Meanwhile, an RNN with the parameter-sharing structure is adopted to its projection, which significantly eases the training burden. Then, to improve the detection performance, we regularize RS-Net by introducing sparse representation. Besides, the step size of iterations in GD part is also investigated for better convergence efficiency. Finally, simulations demonstrate a better decoding trade-off between performance and complexity in RS-Net.
更多查看译文
关键词
large-scale MIMO detection, projected gradient descent, recurrent neural network, sparse representation
AI 理解论文
溯源树
样例
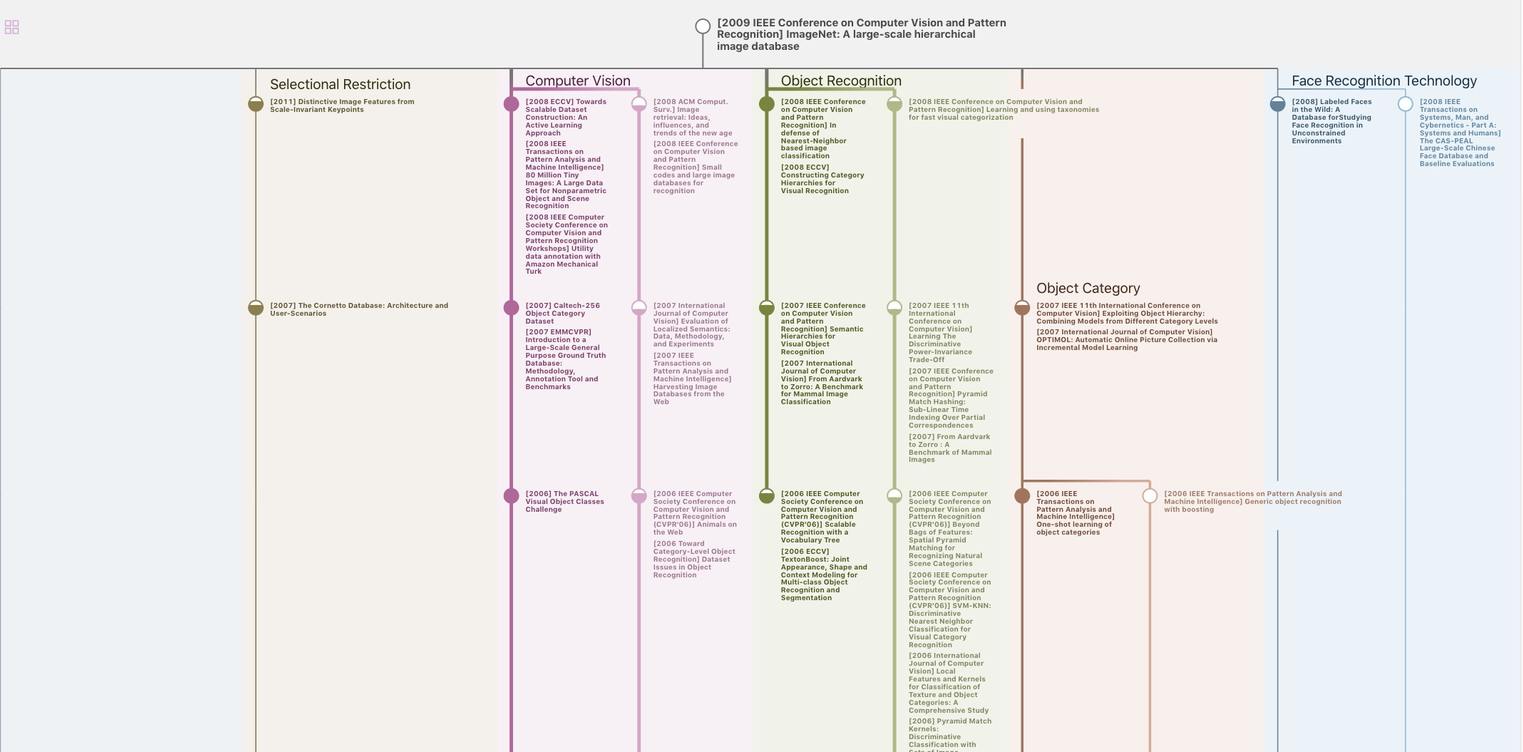
生成溯源树,研究论文发展脉络
Chat Paper
正在生成论文摘要