Log Sequence Anomaly Detection Based on Local Information Extraction and Globally Sparse Transformer Model
IEEE Transactions on Network and Service Management(2021)
摘要
Anomaly detection for log sequences is a necessary task for system intelligent operation and fault diagnosis. In a log sequence, adjacent logs have the property of local correlation, while long-distance logs have remote dependencies. It is helpful to fully mine these information during modeling for improving the performance of anomaly detection. Meanwhile, there are some redundant information or noise in the log sequence, which has no contribution to the detection, and may even bring negative impact. The existing methods for log sequence anomaly detection do not take the above problems into account when constructing models. In this paper, we propose LSADNET, an unsupervised log sequence anomaly detection network based on local information extraction and globally sparse Transformer model. LSADNET applies multi-layer convolution to capture the local correlation between adjacent logs, and utilizes Transformer to learn the global dependency among long-distance logs. Meanwhile, we propose a globally sparse Transformer model to improve the self-attention mechanism, which can help to retain important information adaptively and eliminate the irrelevant information in the log sequence. In addition, according to the co-occurrence mode of log templates, we put forward the calculation formula of log template transfer value, and apply it to log vectorization. Through sufficient experiments on two public datasets, it is confirmed that LSADNET has better performance than the state-of-art methods.
更多查看译文
关键词
Log parsing,anomaly detection,local information extraction,globally sparse transformer
AI 理解论文
溯源树
样例
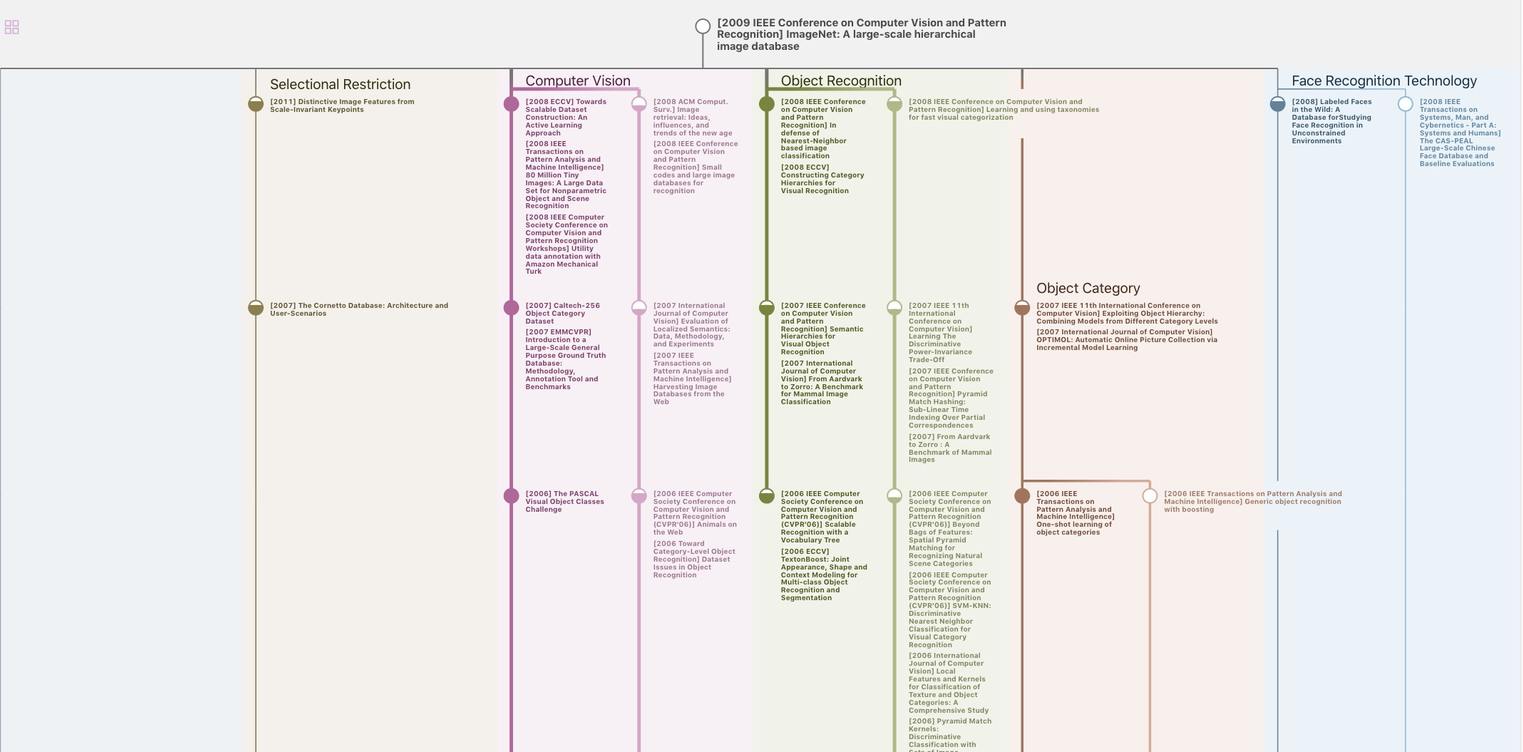
生成溯源树,研究论文发展脉络
Chat Paper
正在生成论文摘要