Know Thy Strengths: Comprehensive Dialogue State Tracking Diagnostics
arxiv(2022)
摘要
Recent works that revealed the vulnerability of dialogue state tracking (DST) models to distributional shifts have made holistic comparisons on robustness and qualitative analyses increasingly important for understanding their relative performance. We present our findings from standardized and comprehensive DST diagnoses, which have previously been sparse and uncoordinated, using our toolkit, CheckDST, a collection of robustness tests and failure mode analytics. We discover that different classes of DST models have clear strengths and weaknesses, where generation models are more promising for handling language variety while span-based classification models are more robust to unseen entities. Prompted by this discovery, we also compare checkpoints from the same model and find that the standard practice of selecting checkpoints using validation loss/accuracy is prone to overfitting and each model class has distinct patterns of failure. Lastly, we demonstrate how our diagnoses motivate a pre-finetuning procedure with non-dialogue data that offers comprehensive improvements to generation models by alleviating the impact of distributional shifts through transfer learning.
更多查看译文
关键词
dialogue,diagnostics,tracking,state
AI 理解论文
溯源树
样例
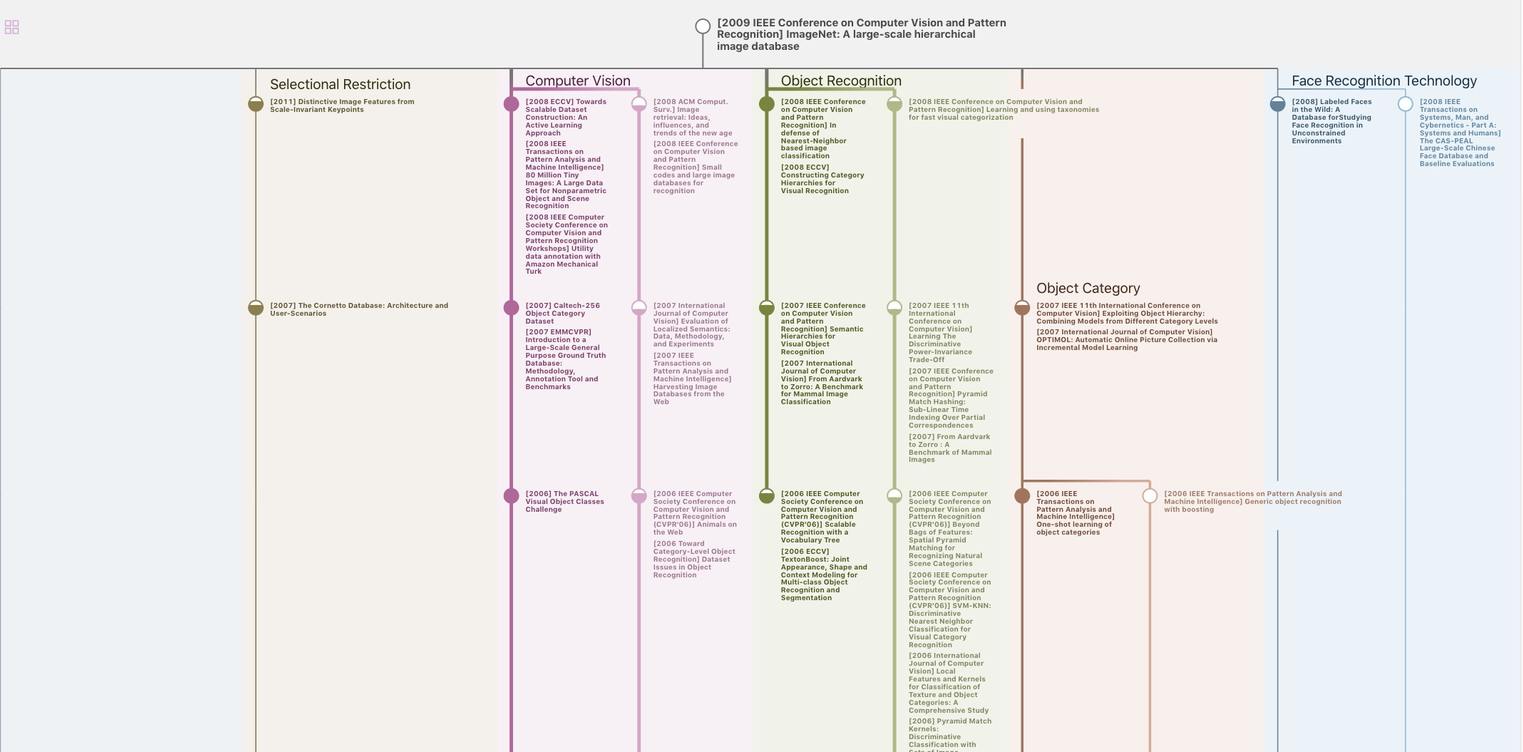
生成溯源树,研究论文发展脉络
Chat Paper
正在生成论文摘要