Visualizing Ensemble Predictions of Music Mood
IEEE Transactions on Visualization and Computer Graphics(2023)
摘要
Music mood classification has been a challenging problem in comparison with other music classification problems (e.g., genre, composer, or period). One solution for addressing this challenge is to use an ensemble of machine learning models. In this paper, we show that visualization techniques can effectively convey the popular prediction as well as uncertainty at different music sections along the temporal axis while enabling the analysis of individual ML models in conjunction with their application to different musical data. In addition to the traditional visual designs, such as stacked line graph, ThemeRiver, and pixel-based visualization, we introduce a new variant of ThemeRiver, called “dual-flux ThemeRiver”, which allows viewers to observe and measure the most popular prediction more easily than stacked line graph and ThemeRiver. Together with pixel-based visualization, dual-flux ThemeRiver plots can also assist in model-development workflows, in addition to annotating music using ensemble model predictions.
更多查看译文
关键词
time-series visualization,ensemble learning,music mood classification
AI 理解论文
溯源树
样例
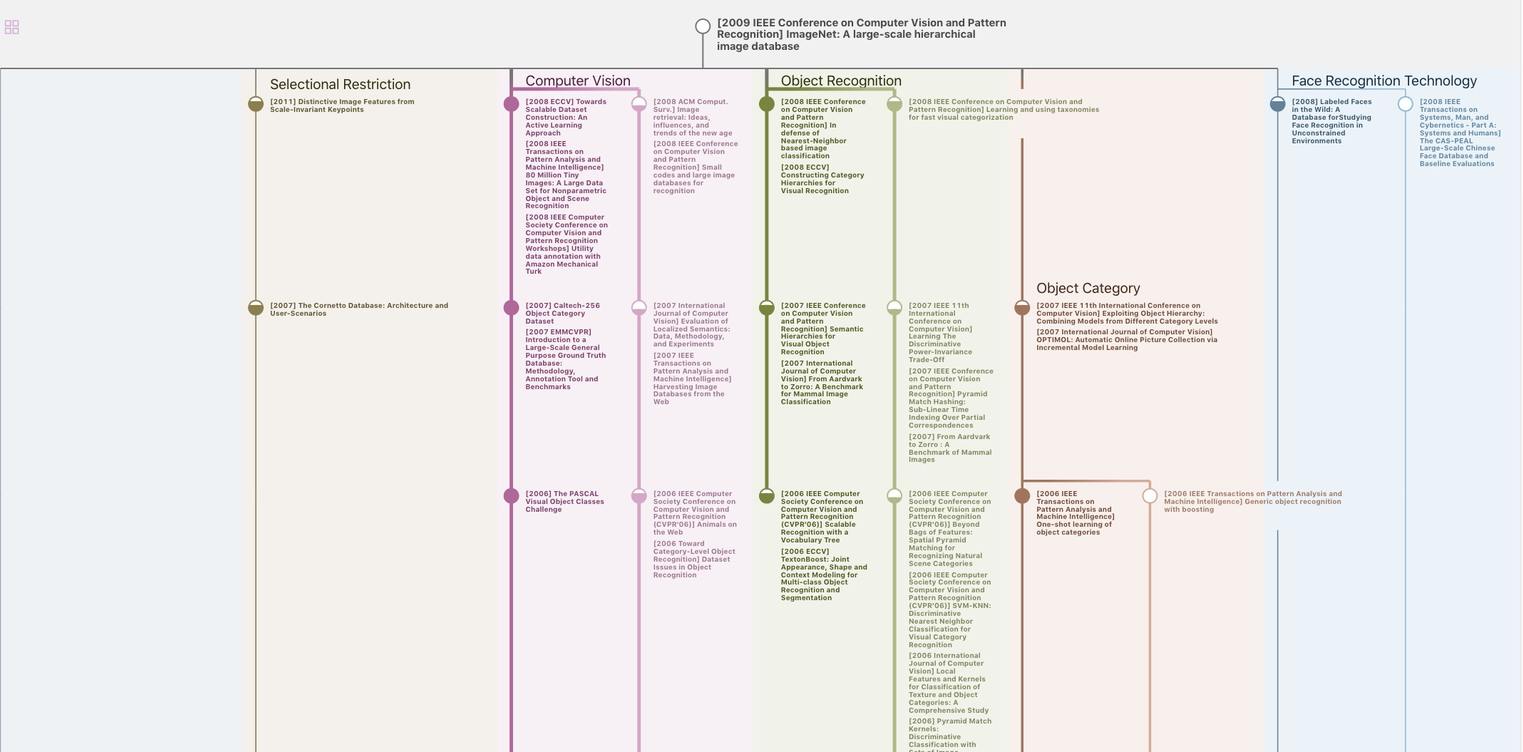
生成溯源树,研究论文发展脉络
Chat Paper
正在生成论文摘要