Personalizing emotion recognition using incremental random forests
29TH EUROPEAN SIGNAL PROCESSING CONFERENCE (EUSIPCO 2021)(2021)
摘要
Affective learning analytics is based on video exchanges within students and between students and teachers. Their aim is to manage both cognitive and affective loads. Affective state detection from videos is subject to identity bias, particularly morphological and behavioral. Although machine learning can fairly detect basic expressions (as defined by Paul Ekman), it hardly deals with these biases and perform better after personalization to a given user (or set of users). But this adaptation needs to train incrementally algorithms with new data. We propose here to use incremental random forests that show abilities to deal with covariate shift in data. We initially train our model on CFEE dataset. Then we train incrementally and evaluate on benchmark CK dataset. Experimental results show that, after progressive training, precision increases. These results suggest that the use of incremental training can improve the accuracy of emotion recognition by specializing the model on a given subject.
更多查看译文
关键词
Incremental Learning, Semi-supervised Learning, Emotion Recognition, Random Forest
AI 理解论文
溯源树
样例
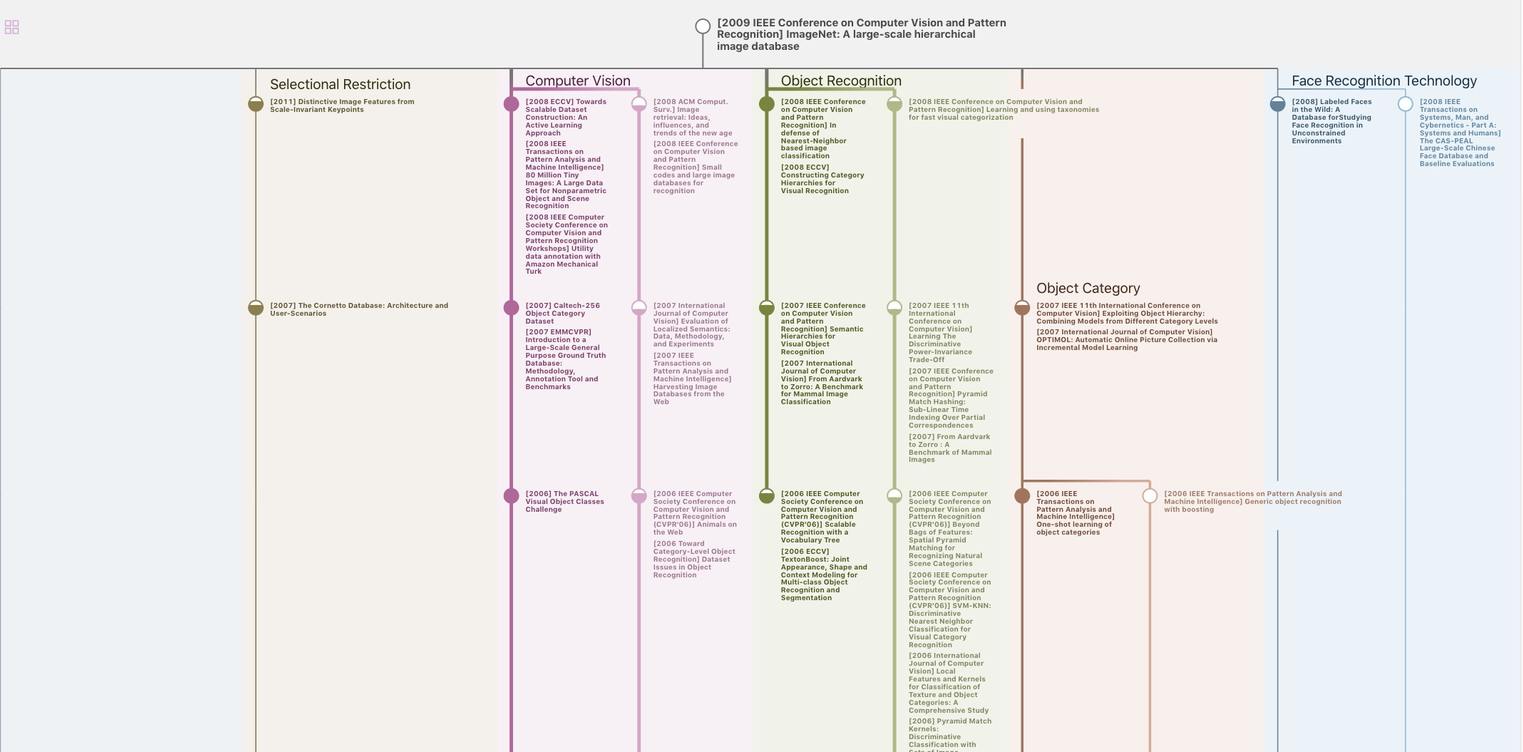
生成溯源树,研究论文发展脉络
Chat Paper
正在生成论文摘要