Dimensionality Reduction for Ordinal Classification
29TH EUROPEAN SIGNAL PROCESSING CONFERENCE (EUSIPCO 2021)(2021)
摘要
Many unsupervised and supervised dimension reduction techniques are available for visualization and interpretation of high-dimensional data for classification tasks. While the unsupervised techniques do not employ the class information at all, most supervised algorithms are blind to the order of classes in ordinal classification problems. In this paper, we propose a novel and intuitive dimension reduction technique specifically designed for visualization of high-dimensional features in ordinal classification tasks. The technique is an iterative process, where at each iteration a search is conducted in the high-dimensional space to find the viewpoint from which the centers of adjacent classes are seen most distant from each other. The data is then projected to the lower dimensional space defined by the optimum viewpoint. The iteration is terminated when the desired dimensionality is achieved. Experimental results on various ordinal datasets demonstrate that our technique can be used as a complementary tool to the classical dimensionality reduction methods.
更多查看译文
关键词
ordinal classification, dimensionality reduction, data visualization, interpretability
AI 理解论文
溯源树
样例
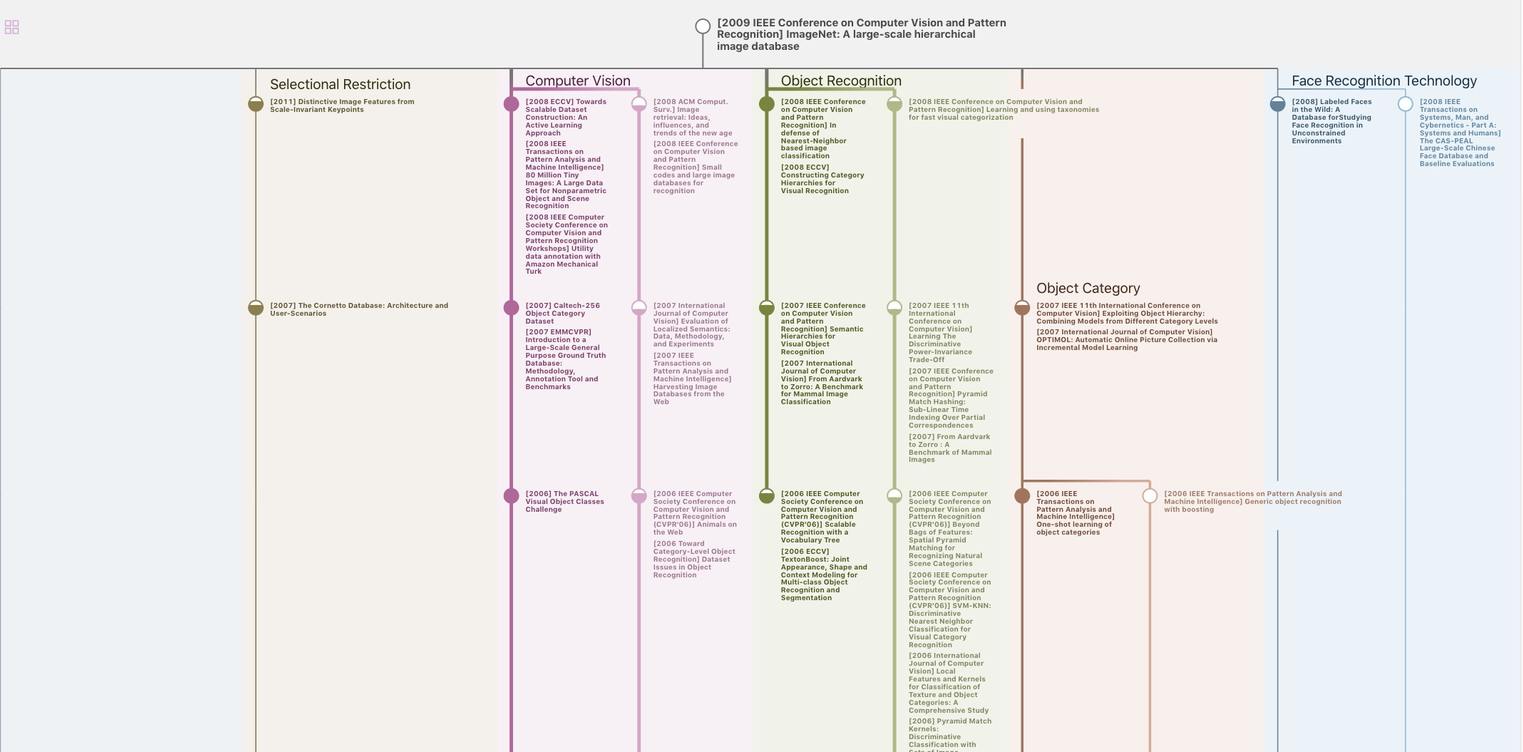
生成溯源树,研究论文发展脉络
Chat Paper
正在生成论文摘要