Targeted Universal Adversarial Perturbations for Automatic Speech Recognition
INFORMATION SECURITY (ISC 2021)(2021)
摘要
Automatic speech recognition (ASR) is an essential technology used in commercial products nowadays. However, the underlying deep learning models used in ASR systems are vulnerable to adversarial examples (AEs), which are generated by applying small or imperceptible perturbations to audio to fool these models. Recently, universal adversarial perturbations (UAPs) have attracted much research interest. UAPs used to generate audio AEs are not limited to a specific input audio signal. Instead, given a generic audio signal, audio AEs can be generated by directly applying UAPs. This paper presents a method of generating UAPs based on a targeted phrase. To the best of our knowledge, our proposed method of generating UAPs is the first to successfully attack ASR models with connectionist temporal classification (CTC) loss. In addition to generating UAPs, we empirically show that the UAPs can be considered as signals that are transcribed as the target phrase. We also show that the UAPs themselves preserve temporal dependency, such that the audio AEs generated using these UAPs also preserved temporal dependency.
更多查看译文
关键词
Audio adversarial example, Universal adversarial perturbations, Automatic speech recognition, Deep learning, Machine learning
AI 理解论文
溯源树
样例
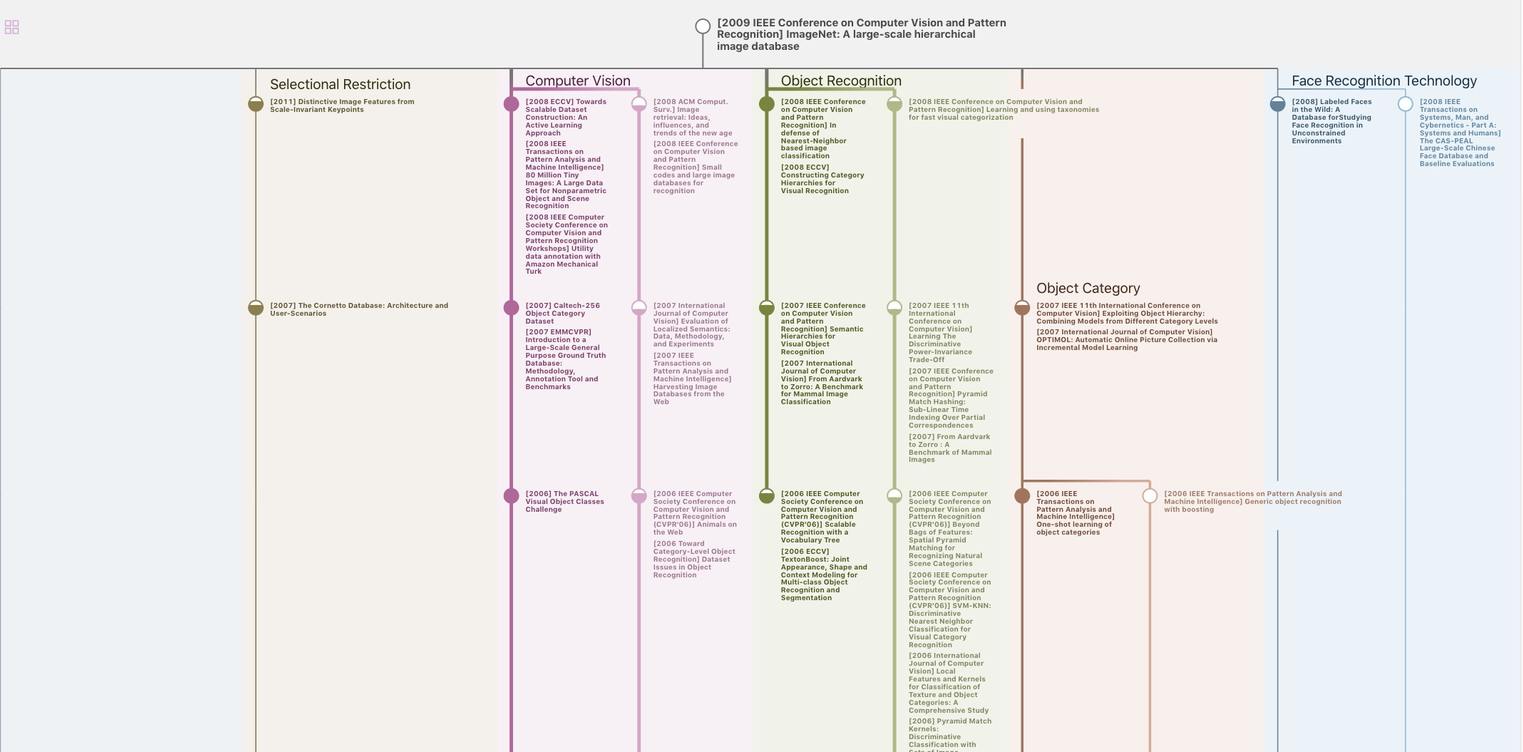
生成溯源树,研究论文发展脉络
Chat Paper
正在生成论文摘要