Synthetic Data Generation using Imitation Training.
IEEE International Conference on Computer Vision(2021)
摘要
We propose a strategic approach to generate synthetic data in order to improve machine learning algorithms such as Deep Neural Networks (DNN). Utilization of synthetic data has shown promising results yet there are no specific rules or recipes on how to generate and cook synthetic data. We propose imitation training as a guideline of synthetic data generation to add more underrepresented entities and balance the data distribution for DNN to handle corner cases and resolve long tail problems. The proposed imitation training has a circular process with three main steps: First, the existing system is evaluated and failure cases such as false positive and false negative detections are sorted out; Secondly, synthetic data imitating such failure cases is created with domain randomization; Thirdly, we train a network with the existing data and the newly added synthetic data; We repeat these three steps until the evaluation metric converges. We validated the approach by experimenting on object detection in autonomous driving.
更多查看译文
关键词
synthetic data generation,imitation training,data distribution,deep neural networks,DNN,false positive detection,false negative detection,domain randomization,object detection,autonomous driving
AI 理解论文
溯源树
样例
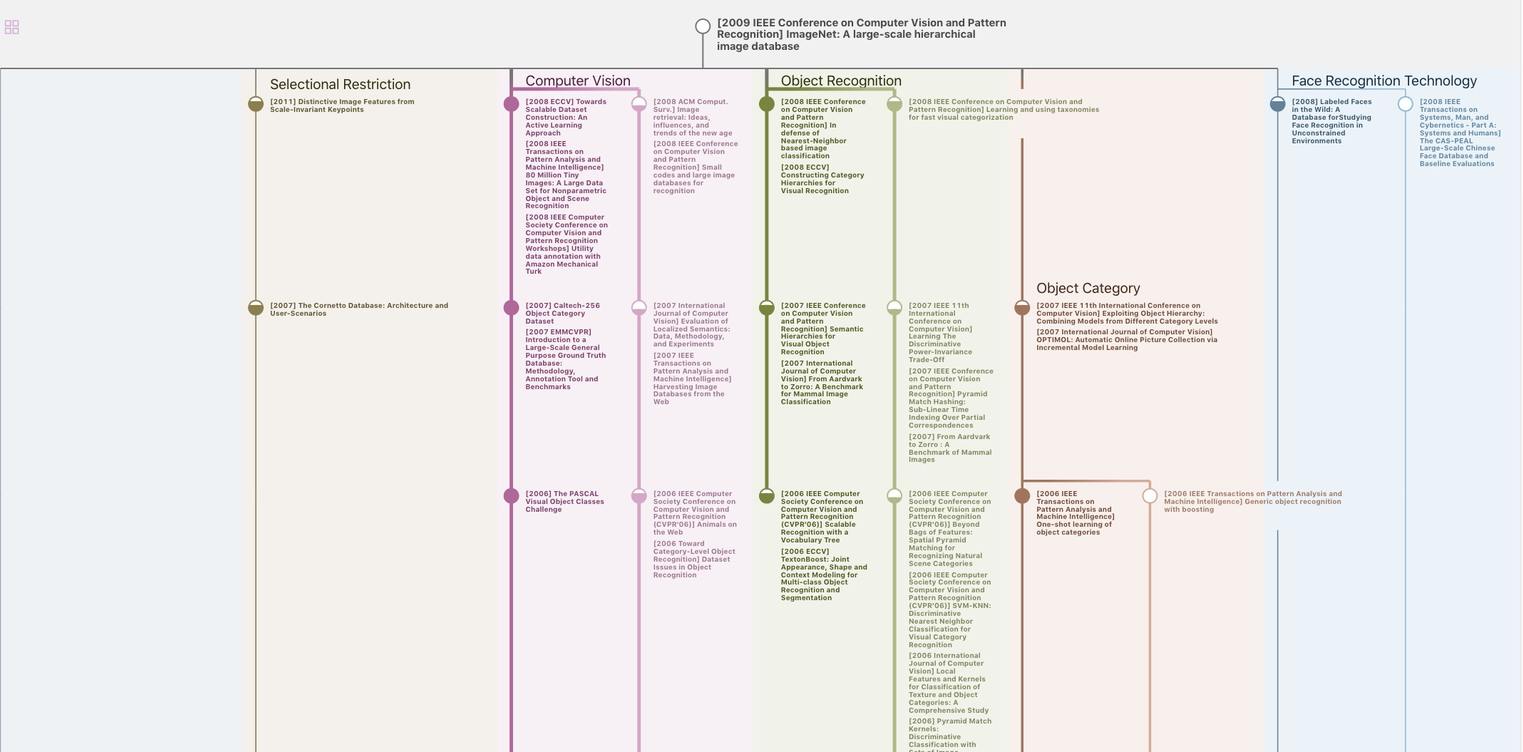
生成溯源树,研究论文发展脉络
Chat Paper
正在生成论文摘要