A Deep Learning Model to Extract Ship Size From Sentinel-1 SAR Images
IEEE TRANSACTIONS ON GEOSCIENCE AND REMOTE SENSING(2022)
摘要
This study develops a deep learning (DL) model to extract the ship size from Sentinel-1 synthetic aperture radar (SAR) images, named SSENet. We employ a single shot multibox detector (SSD)-based model to generate a rotatable bounding box (RBB) for the ship. We design a deep-neural-network (DNN)-based regression model to estimate the accurate ship size. The hybrid inputs to the DNN-based model include the initial ship size and orientation angle obtained from the RBB and the abstracted features extracted from the input SAR image. We design a custom loss function named mean scaled square error (MSSE) to optimize the DNN-based model. The DNN-based model is concatenated with the SSD-based model to form the integrated SSENet. We employ a subset of the OpenSARShip, a data set dedicated to Sentinel-1 ship interpretation, to train and test SSENet. The training/testing data set includes 1500/390 ship samples. Experiments show that SSENet is capable of extracting the ship size from SAR images end to end. The mean absolute errors (MAEs) are under 0.8 pixels, and their length and width are 7.88 and 2.23 m, respectively. The hybrid input significantly improves the model performance. The MSSE reduces the MAE of length by nearly 1 m and increases the MAE of width by 0.03m compared to the mean square error (MSE) loss function. Compared with the well-performed gradient boosting regression (GBR) model, SSENet reduces the MAE of length by nearly 2 m (18.68x0025;) and that of width by 0.06 m (2.51x0025;). SSENet shows robustness on different training/testing sets.
更多查看译文
关键词
Marine vehicles, Radar polarimetry, Feature extraction, Synthetic aperture radar, Data mining, Radar imaging, Oceans, Custom loss function, deep learning (DL), deep neural network (DNN) regression, ship size extraction, synthetic aperture radar (SAR) image
AI 理解论文
溯源树
样例
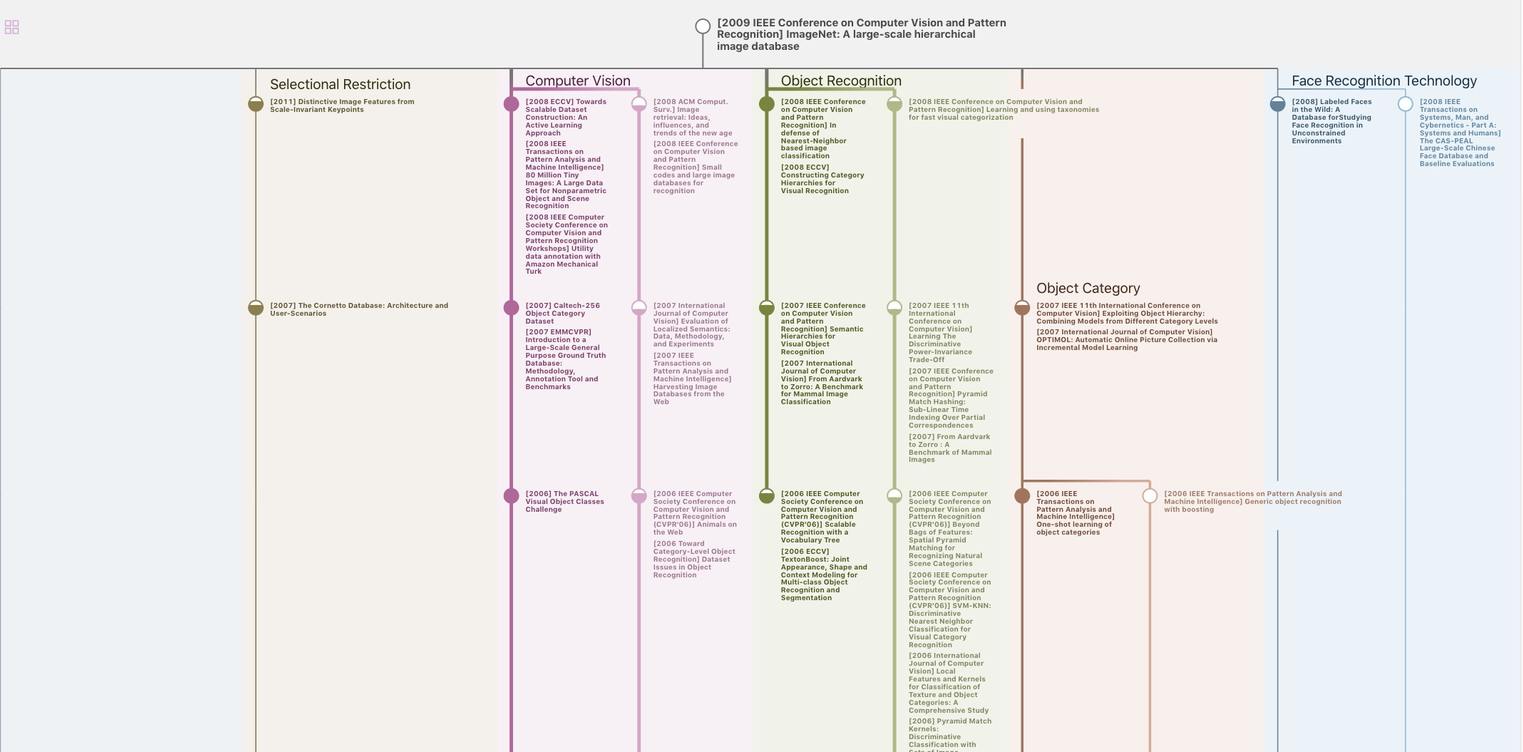
生成溯源树,研究论文发展脉络
Chat Paper
正在生成论文摘要