Predicting Spatiotemporal Demand of Dockless E-Scooter Sharing Services with a Masked Fully Convolutional Network
ISPRS INTERNATIONAL JOURNAL OF GEO-INFORMATION(2021)
摘要
Dockless electric scooters (e-scooter) have emerged as a green alternative to automobiles and a solution to the first- and last-mile problems. Demand anticipation, or being able to accurately predict spatiotemporal demand of e-scooter usage, is one supply-demand balancing strategy. In this paper, we present a dockless e-scooter demand prediction model based on a fully convolutional network (FCN) coupled with a masking process and a weighted loss function, namely, masked FCN (or MFCN). The MFCN model handles the sparse e-scooter usage data with its masking process and weighted loss function. The model is trained with highly correlated features through our feature selection process. Next-hour and next 24-h prediction schemes have been tested for both pick-up and drop-off demands. Overall, the proposed MFCN outperforms other baseline models including a naive forecasting, linear regression, and convolutional long short-term memory networks with mean absolute errors of 0.0434 and 0.0464 for the next-hour pick-up and drop-off demand prediction, respectively, and the errors of 0.0491 and 0.0501 for the next 24-h pick-up and drop-off demand prediction, respectively. The developed MFCN expands the collection of deep learning techniques that can be applied in the transportation domain, especially spatiotemporal demand prediction.
更多查看译文
关键词
e-scooter, micromobility, free-floating systems, urban mobility, mobility-as-a-service, spatiotemporal demand, demand prediction, fully convolutional network
AI 理解论文
溯源树
样例
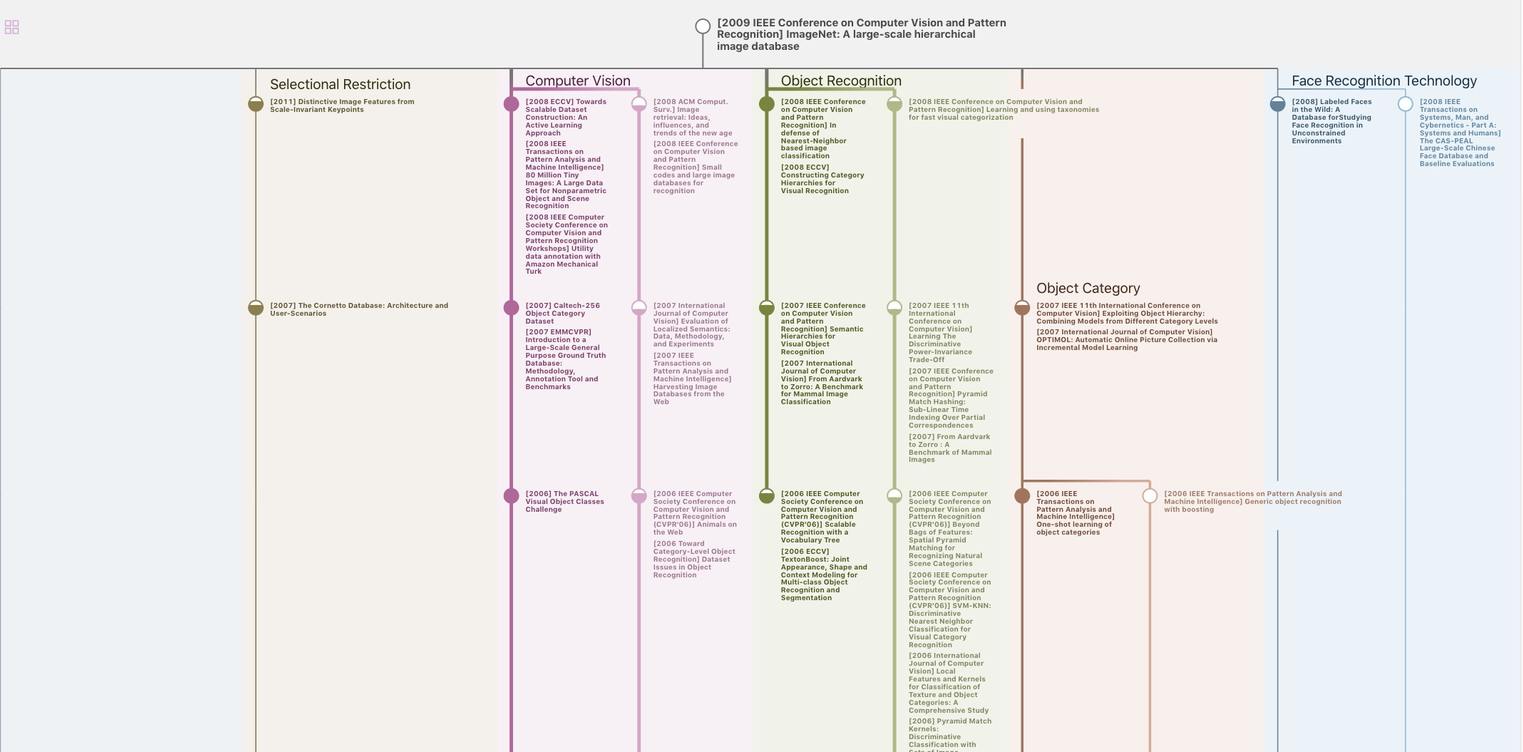
生成溯源树,研究论文发展脉络
Chat Paper
正在生成论文摘要