A Method Based On Dual-Network Information Fusion to Predict MiRNA-Disease Associations.
IEEE/ACM Transactions on Computational Biology and Bioinformatics(2023)
摘要
MicroRNAs (miRNAs) are single-stranded small RNAs. An increasing number of studies have shown that miRNAs play a vital role in many important biological processes. However, some experimental methods to predict unknown miRNA-disease associations (MDAs) are time-consuming and costly. Only a small percentage of MDAs are verified by researchers. Therefore, there is a great need for high-speed and efficient methods to predict novel MDAs. In this paper, a new computational method based on Dual-Network Information Fusion (DNIF) is developed to predict potential MDAs. Specifically, on the one hand, two enhanced sub-models are integrated to reconstruct an effective prediction framework; on the other hand, the prediction performance of the algorithm is improved by fully fusing multiple omics data information, including validated miRNA-disease associations network, miRNA functional similarity, disease semantic similarity and Gaussian interaction profile (GIP) kernel network associations. As a result, DNIF achieves the excellent performance under situation of 5-fold cross validation (average AUC of 0.9571). In the cases study of three important human diseases, our model has achieved satisfactory performance in predicting potential miRNAs for certain diseases. The reliable experimental results demonstrate that DNIF could serve as an effective calculation method to accelerate the identification of MDAs.
更多查看译文
关键词
MiRNA-disease associations,Gaussian interaction kernel,Kronecker regularized least squares,Information Fusion Strategy
AI 理解论文
溯源树
样例
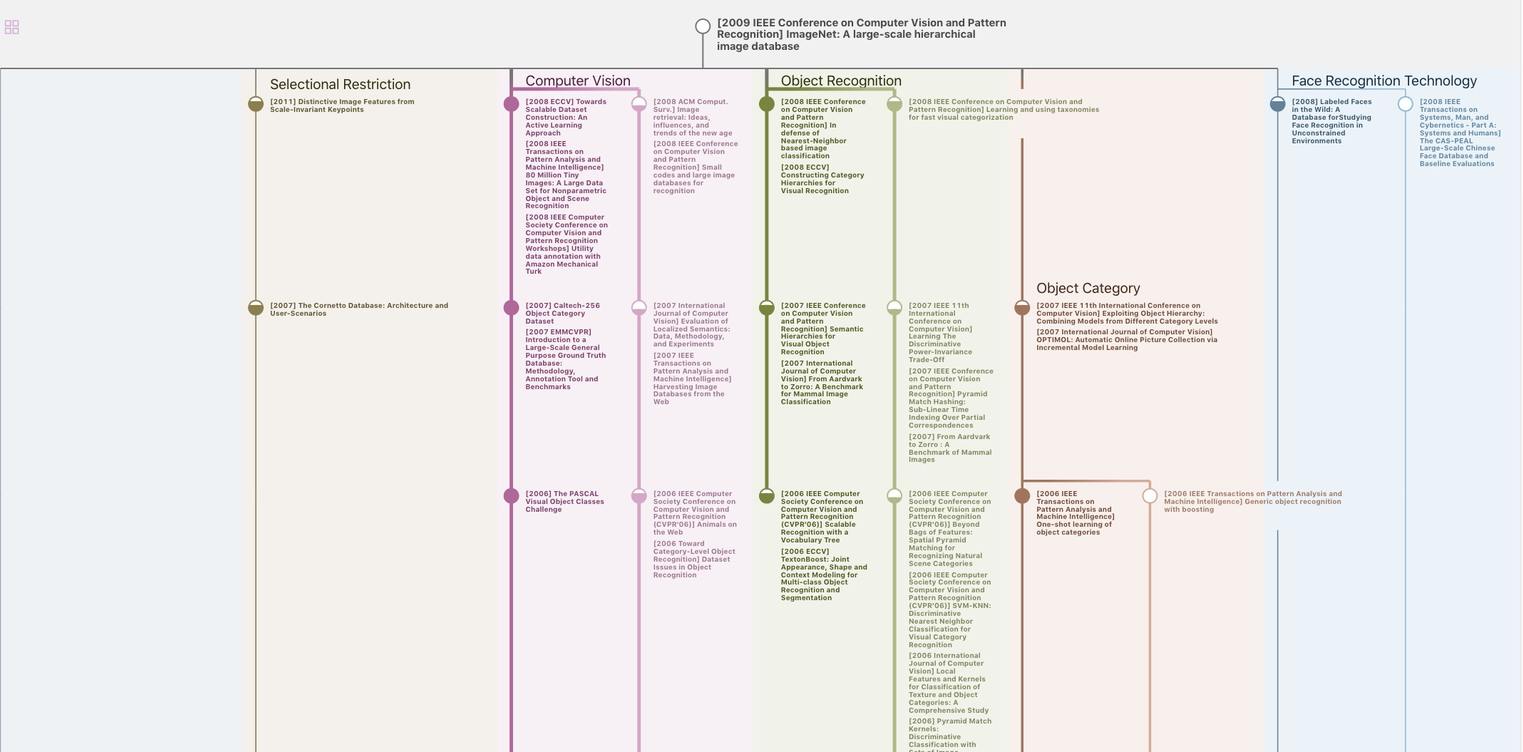
生成溯源树,研究论文发展脉络
Chat Paper
正在生成论文摘要