Transfer learning for automatic joint segmentation of thyroid and breast lesions from ultrasound images
INTERNATIONAL JOURNAL OF COMPUTER ASSISTED RADIOLOGY AND SURGERY(2021)
摘要
P urpose It plays a significant role to accurately and automatically segment lesions from ultrasound (US) images in clinical application. Nevertheless, it is extremely challenging because distinct components of heterogeneous lesions are similar to background in US images. In our study, a transfer learning-based method is developed for full-automatic joint segmentation of nodular lesions. M ethods Transfer learning is a widely used method to build high performing computer vision models. Our transfer learning model is a novel type of densely connected convolutional network (SDenseNet). Specifically, we pre-train SDenseNet based on ImageNet dataset. Then our SDenseNet is designed as a multi-channel model (denoted Mul-DenseNet) for automatically jointly segmenting lesions. As comparison, our SDenseNet using different transfer learning is applied to segmenting nodules, respectively. In our study, we find that more datasets for pre-training and multiple pre-training do not always work in segmentation of nodules, and the performance of transfer learning depends on a judicious choice of dataset and characteristics of targets. R esults Experimental results illustrate a significant performance of the Mul-DenseNet compared to that of other methods in the study. Specially, for thyroid nodule segmentation, overlap metric (OM), dice ratio (DR), true-positive rate (TPR), false-positive rate (FPR) and modified Hausdorff distance (MHD) are 0.9257± 0.0027 , 0.9596± 0.0009 , 0.9869± 0.0008 , 0.0183± 0.0066 and 0.3897± 0.3488 mm, respectively; for breast nodule segmentation, OM, DR, TPR, FPR and MHD are 0.8912± 0.0072 , 0.9513± 0.0038 , 0.9835± 0.0015 , 0.2381± 0.1301 and 0.2017± 0.0302 mm, respectively. C onclusions The experimental results illustrate our transfer learning models are very effective in segmentation of lesions, which also demonstrate that it is potential of our proposed Mul-DenseNet model in clinical applications. This model can reduce heavy workload of the physicians so that it can avoid misdiagnosis cases due to excessive fatigue. Moreover, it is easy and reproducible to detect lesions without medical expertise.
更多查看译文
关键词
Transfer learning, Densely connected convolutional network, Lesions, Ultrasound image, Segmentation
AI 理解论文
溯源树
样例
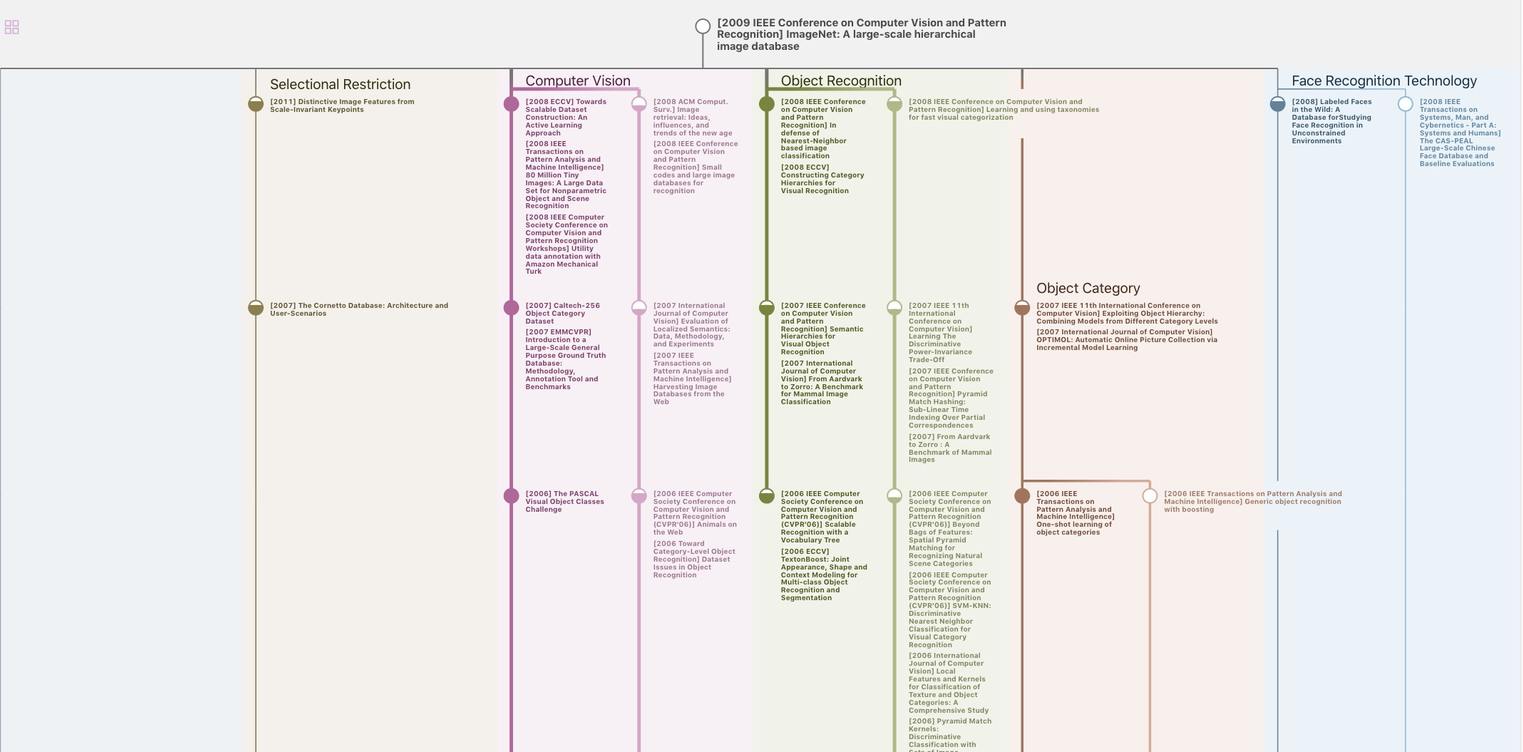
生成溯源树,研究论文发展脉络
Chat Paper
正在生成论文摘要