CMash: fast, multi-resolution estimation of k-mer-based Jaccard and containment indices
BIOINFORMATICS(2022)
摘要
Motivation: K-mer-based methods are used ubiquitously in the field of computational biology. However, determining the optimal value of k for a specific application often remains heuristic. Simply reconstructing a new k-mer set with another k-mer size is computationally expensive, especially in metagenomic analysis where datasets are large. Here, we introduce a hashing-based technique that leverages a kind of bottom-m sketch as well as a k-mer ternary search tree (KTST) to obtain k-mer-based similarity estimates for a range of k values. By truncating k-mers stored in a pre-built KTST with a large k = k(max) value, we can simultaneously obtain k-mer-based estimates for all k values up to k(max). This truncation approach circumvents the reconstruction of new k-mer sets when changing k values, making analysis more time and space-efficient. Results: We derived the theoretical expression of the bias factor due to truncation. And we showed that the biases are negligible in practice: when using a KTST to estimate the containment index between a RefSeq-based microbial reference database and simulated metagenome data for 10 values of k, the running time was close to 10x faster compared to a classic MinHash approach while using less than one-fifth the space to store the data structure.
更多查看译文
关键词
jaccard,estimation,multi-resolution,k-mer-based
AI 理解论文
溯源树
样例
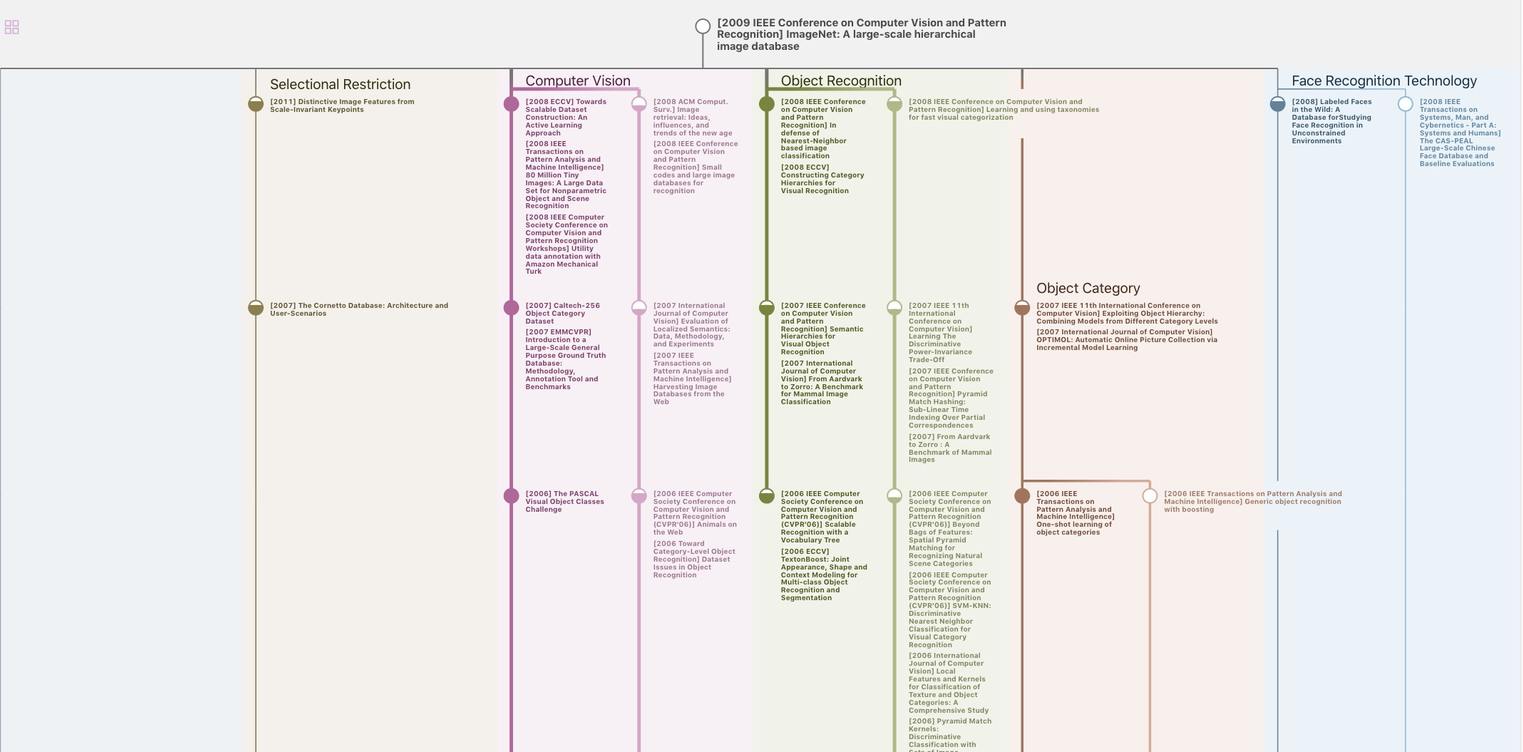
生成溯源树,研究论文发展脉络
Chat Paper
正在生成论文摘要