Two Wrongs Don't Make a Right: Combating Confirmation Bias in Learning with Label Noise
arxiv(2023)
摘要
Noisy labels damage the performance of deep networks. For robust learning, a prominent two-stage pipeline alternates between eliminating possible incorrect labels and semi-supervised training. However, discarding part of noisy labels could result in a loss of information, especially when the corruption has a dependency on data, e.g., class-dependent or instance-dependent. Moreover, from the training dynamics of a representative two-stage method DivideMix, we identify the domination of confirmation bias: pseudo-labels fail to correct a considerable amount of noisy labels, and consequently, the errors accumulate. To sufficiently exploit information from noisy labels and mitigate wrong corrections, we propose Robust Label Refurbishment (Robust LR) a new hybrid method that integrates pseudo-labeling and confidence estimation techniques to refurbish noisy labels. We show that our method successfully alleviates the damage of both label noise and confirmation bias. As a result, it achieves state-of-the-art performance across datasets and noise types, namely CIFAR under different levels of synthetic noise and Mini-WebVision and ANIMAL-10N with real-world noise.
更多查看译文
关键词
confirmation bias,learning
AI 理解论文
溯源树
样例
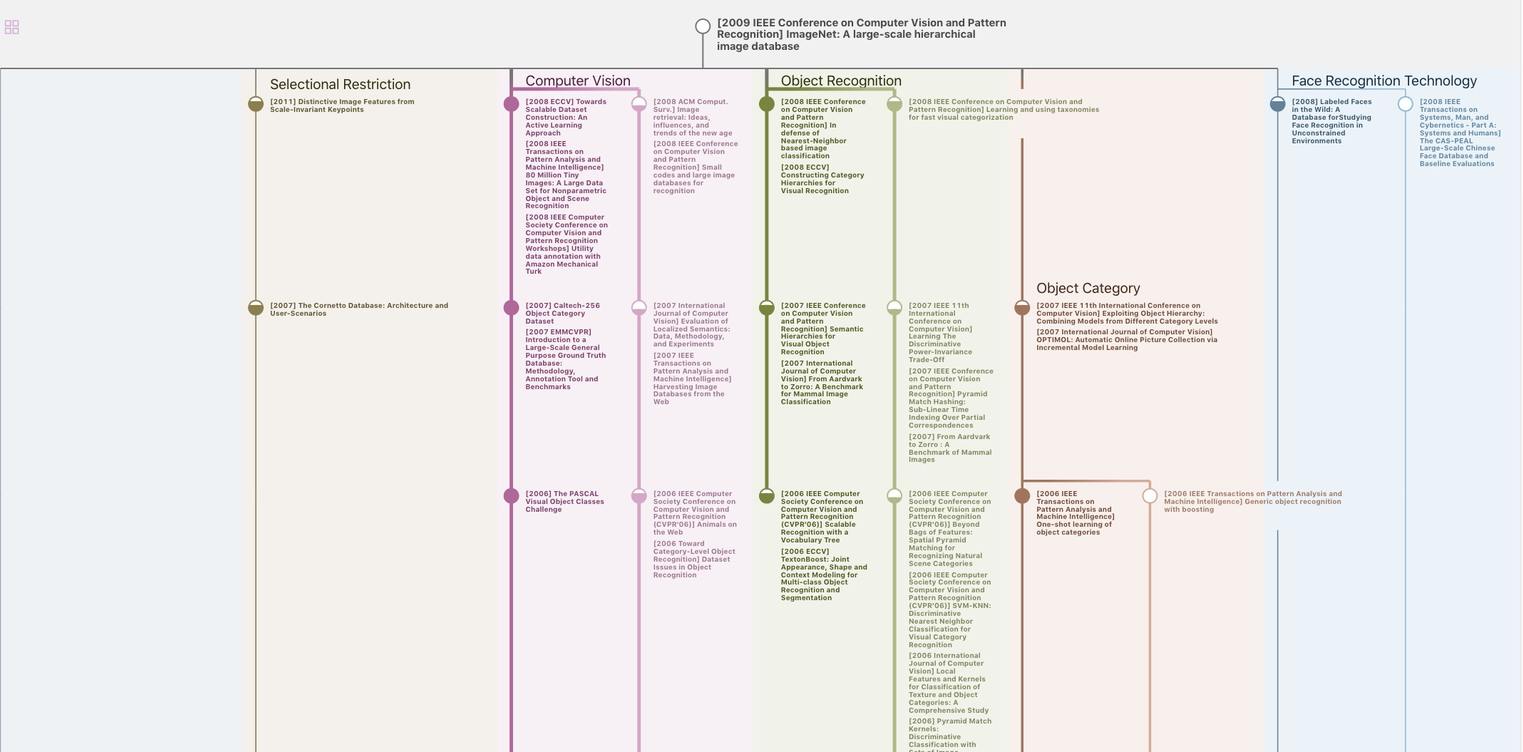
生成溯源树,研究论文发展脉络
Chat Paper
正在生成论文摘要