A Deep Learning Ensemble to Classify Anxiety, Depression, and their Comorbidity from Texts of Social Networks.
J. Inf. Data Manag.(2021)
摘要
The use of social networks to expose personal difficulties has enabled works on the automatic identification of specific mental conditions, particularly depression. Depression is the most incapacitating disease worldwide, and it has an alarming comorbidity rate with anxiety. In this paper, we explore deep learning techniques to develop a stacking ensemble to automatically identify depression, anxiety, and comorbidity, using data extracted from Reddit. The stacking is composed of specialized single-label binary classifiers that distinguish between specific disorders and control users. A meta-learner explores these base classifiers as a context for reaching a multi-label, multi-class decision. We developed extensive experiments using alternative architectures (LSTM, CNN, and their combination), word embeddings, and ensemble topologies. All base classifiers and ensembles outperformed the baselines. The CNN-based binary classifiers achieved the best performance, with f-measures of 0.79 for depression, 0.78 for anxiety, and 0.78 for comorbidity. The ensemble topology with best performance (Hamming Loss of 0.29 and Exact Match Ratio of 0.47) combines base classifiers according to three architectures, and do not include comorbidity classifiers. Using SHAP, we confirmed the influential features are related to symptoms of these disorders.
更多查看译文
AI 理解论文
溯源树
样例
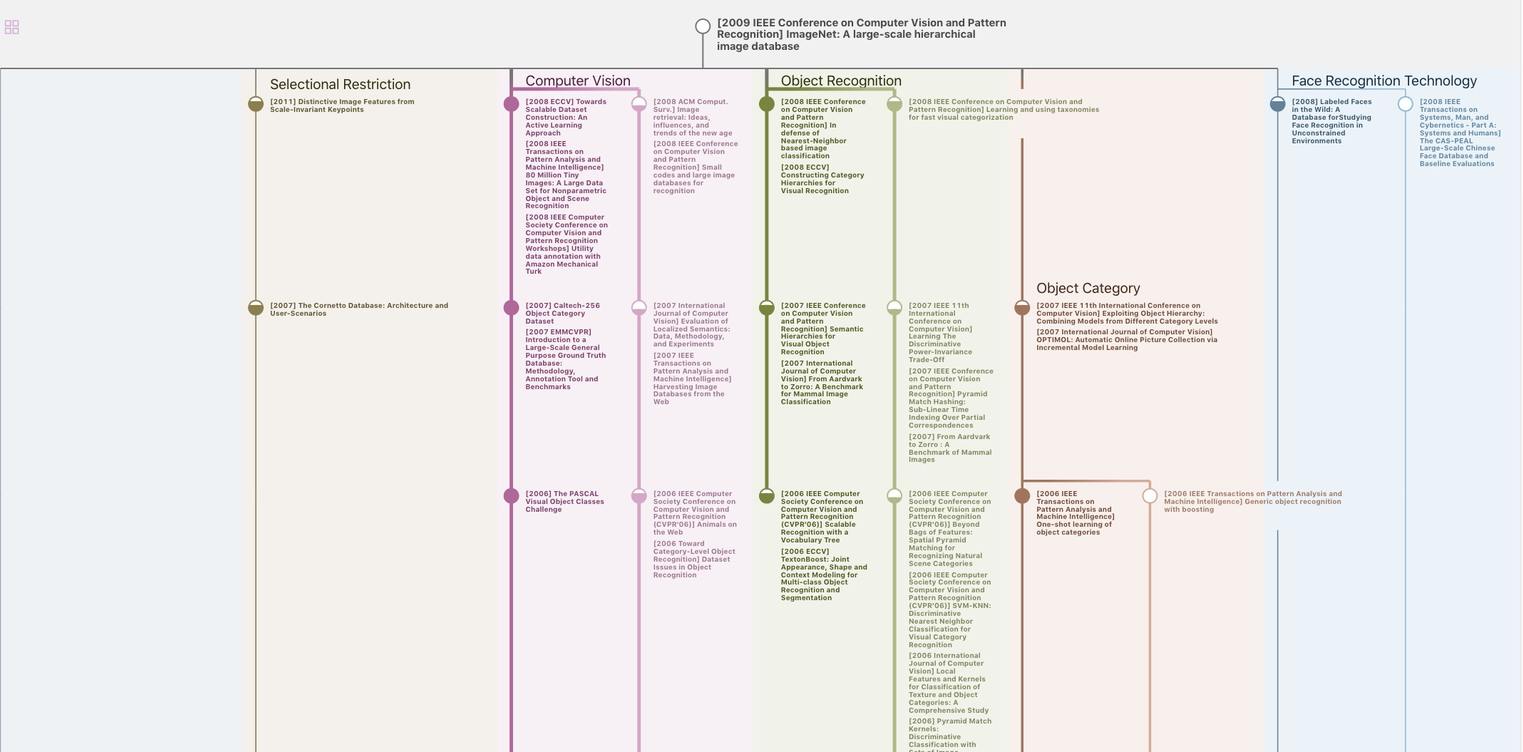
生成溯源树,研究论文发展脉络
Chat Paper
正在生成论文摘要