Time-series data dynamic density clustering
INTELLIGENT DATA ANALYSIS(2021)
摘要
In many clustering problems, the whole data is not always static. Over time, part of it is likely to be changed, such as updated, erased, etc. Suffer this effect, the timeline can be divided into multiple time segments. And, the data at each time slice is static. Then, the data along the timeline shows a series of dynamic intermediate states. The union set of data from all time slices is called the time-series data. Obviously, the traditional clustering process does not apply directly to the time-series data. Meanwhile, repeating the clustering process at every time slices costs tremendous. In this paper, we analyze the transition rules of the data set and cluster structure when the time slice shifts to the next. We find there is a distinct correlation of data set and succession of cluster structure between two adjacent ones, which means we can use it to reduce the cost of the whole clustering process. Inspired by it, we propose a dynamic density clustering method (DDC) for time-series data. In the simulations, we choose 6 representative problems to construct the time-series data for testing DDC. The results show DDC can get high accuracy results for all 6 problems while reducing the overall cost markedly.
更多查看译文
关键词
Time-series data, dynamic clustering, density clustering
AI 理解论文
溯源树
样例
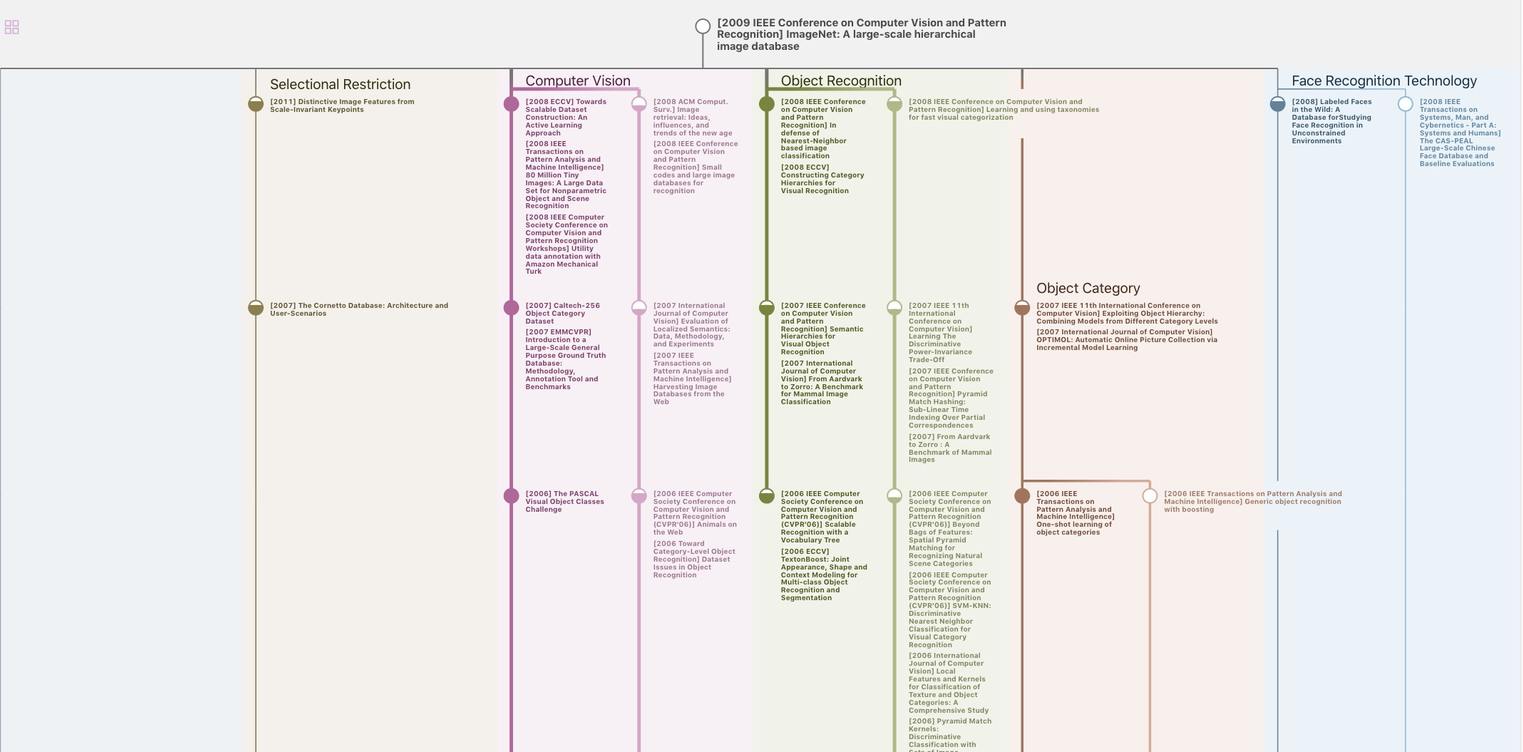
生成溯源树,研究论文发展脉络
Chat Paper
正在生成论文摘要