Probabilistic Approach for Road-Users Detection
arxiv(2023)
摘要
Object detection in autonomous driving applications implies that the detection and tracking of semantic objects are commonly native to urban driving environments, as pedestrians and vehicles. One of the major challenges in state-of-the-art deep-learning based object detection are false positives which occur with overconfident scores. This is highly undesirable in autonomous driving and other critical robotic-perception domains because of safety concerns. This paper proposes an approach to alleviate the problem of overconfident predictions by introducing a novel probabilistic layer to deep object detection networks in testing. The suggested approach avoids the traditional Sigmoid or Softmax prediction layer which often produces overconfident predictions. It is demonstrated that the proposed technique reduces overconfidence in the false positives without degrading the performance on the true positives. The approach is validated on the 2D-KITTI objection detection through the YOLOV4 and SECOND (Lidar-based detector). The proposed approach enables interpretable probabilistic predictions without the requirement of re-training the network and therefore is very practical.
更多查看译文
关键词
probabilistic approach,detection,road-users
AI 理解论文
溯源树
样例
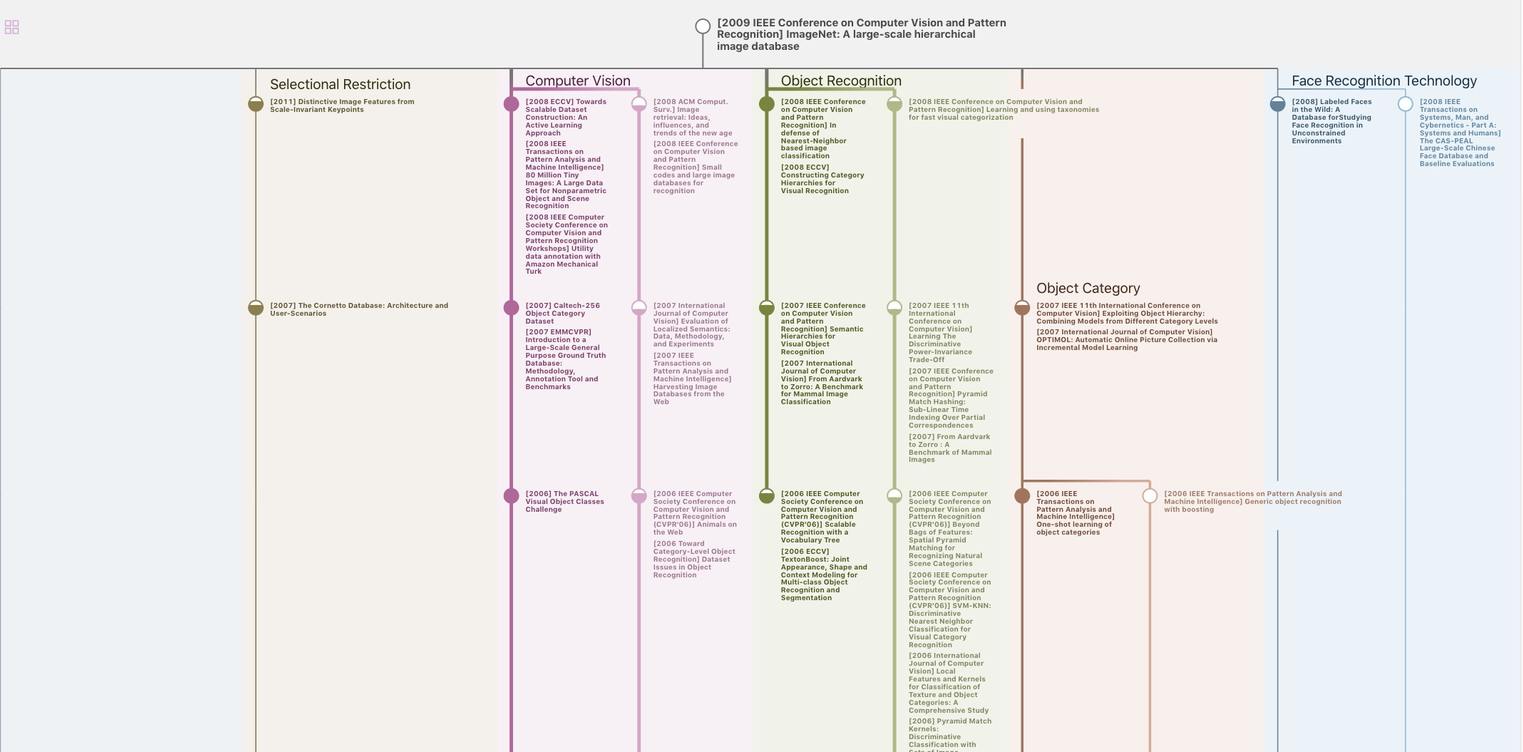
生成溯源树,研究论文发展脉络
Chat Paper
正在生成论文摘要