Building Healthy Recommendation Sequences for Everyone:A Safe Reinforcement Learning Approach
user-613ea93de55422cecdace10f(2021)
摘要
A key consideration in the design of recommender systems is the long term well-being of users. In this work, we formalize this challenge as a multi-objective safe reinforcement learning problem, balancing positive user feedback and the “healthiness” of user trajectories. We note that in some cases, naively balancing these objectives can lead to unhealthy experiences, even if unlikely, still occurring in a small subset of users. Therefore, we examine a distributional notion of recommendation safety. We propose a reinforcement learning approach that optimizes for positive feedback from users while simultaneously optimizing for the health of worst-case users to remain high. To empirically validate our method, we develop a research simulation environment motivated by a MovieLens recommendation setting that considers exposure to violence as a proxy for unhealthy recommendations. We demonstrate that our method reduces unhealthy recommendations to the most vulnerable users without sacrificing much user satisfaction.
更多查看译文
AI 理解论文
溯源树
样例
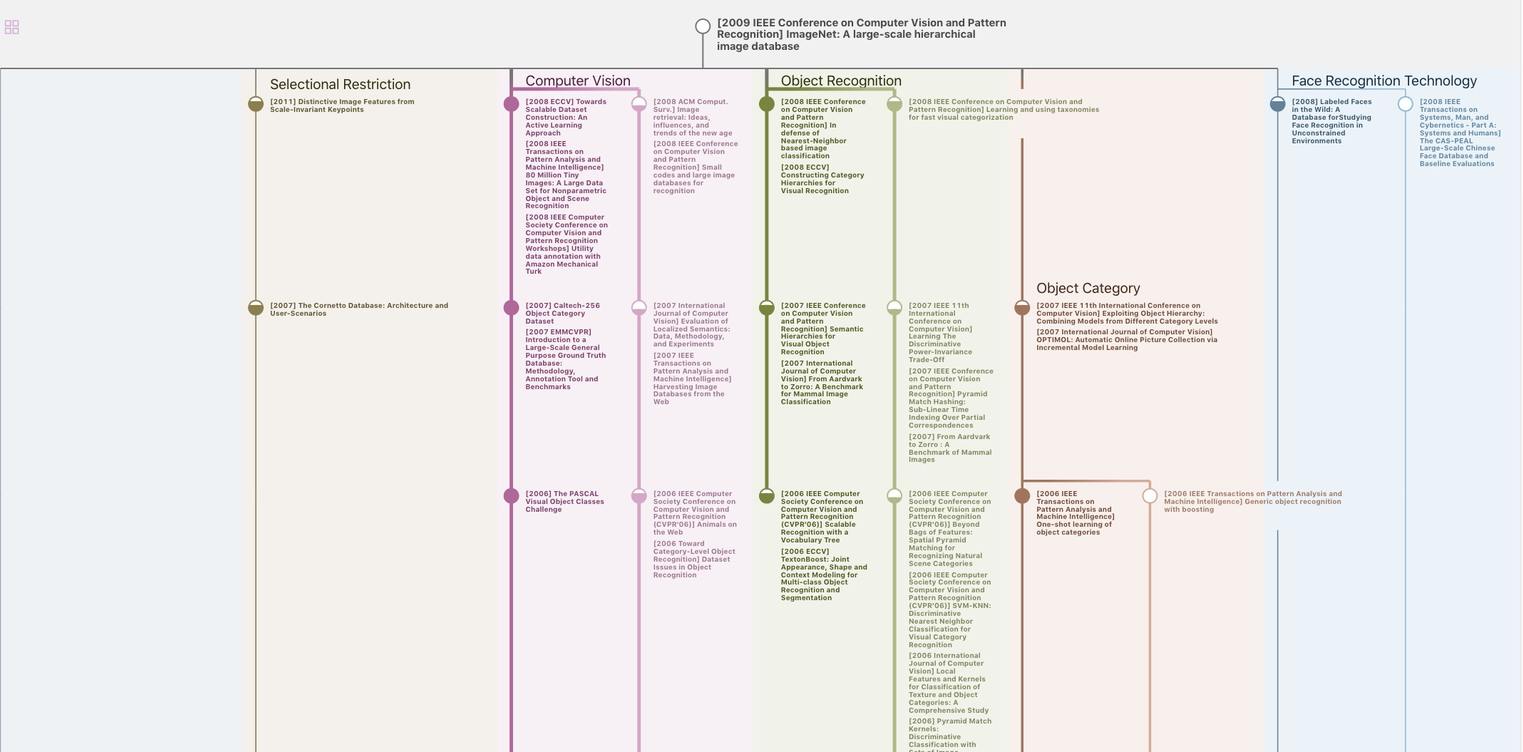
生成溯源树,研究论文发展脉络
Chat Paper
正在生成论文摘要