Temporal convolutional networks for fault diagnosis of photovoltaic systems using satellite and inverter measurements
Embedded Network Sensor Systems(2021)
摘要
ABSTRACTOver time, photovoltaic (PV) systems become increasingly susceptible to faults. Early fault detection and identification not only limits power losses and increases the systems lifetime, but also prevents more serious consequences, such as risk of fire or electrical shock. Although several accurate fault diagnosis methods have been proposed in literature, most PV systems remain unmonitored as the installations are not equipped with the required sensors. In this work, we propose a fault diagnosis technique that does not require on-site sensors. Rather, weather satellite and inverter measurements are used as inputs for the proposed machine learning model. As no dedicated sensors are needed, our method is widely applicable and cost-effective. A temporal convolutional neural network is developed to accurately identify 6 common types of faults, based on the past 24 h of measurements. The proposed approach is tested extensively on a simulated PV system, taking into account multiple severities of each fault type, and reaches an accuracy of over 86%.
更多查看译文
关键词
Fault diagnosis, Photovoltaics, Temporal convolutional network
AI 理解论文
溯源树
样例
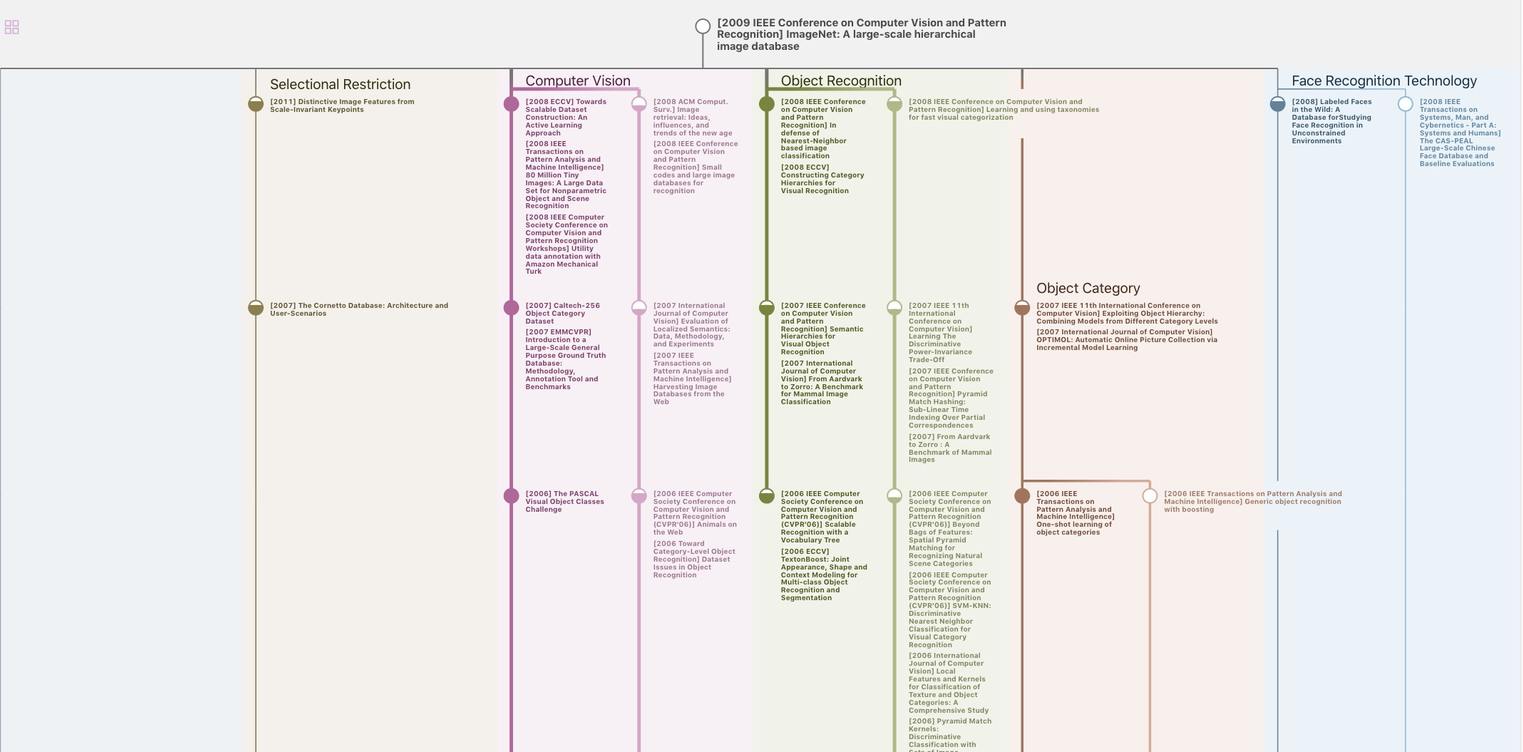
生成溯源树,研究论文发展脉络
Chat Paper
正在生成论文摘要