Hierarchical Models for Detecting Mobility Clusters during COVID-19
MOBICOM(2021)
摘要
ABSTRACTAnalyzing and developing mobility models that accurately capture human mobility is critical for combating the COVID-19 pandemic and minimizing the spread of the disease. In this paper, we design a two-layer hierarchical mobility model to model user mobility in the city of Rio de Janeiro and its suburbs by analyzing cellular network connectivity data during the COVID-19 pandemic. To this end, we collaborate with one of the main network providers in Brazil, TIM Brazil, to collect user connectivity logs from April 5th, 2020 to July 2nd, 2020, which we use to generate mobility graphs. We adopt the Louvain community detection algorithm in the first layer of our hierarchical model to detect the main communities in the city from the mobility graphs. Our model then uses the KMeans++ and Agglomerative clustering methods in the second layer to identify high, medium, and low mobility clusters within each community. Via extensive experiments, we show that the Louvain, and the Kmeans++ and Agglomerative algorithms outperform traditional clustering approaches in the first and second layers, respectively. Our results also demonstrate that our hierarchical model is able to pinpoint main mobility locations within each community and can be used by authorities to implement partial lockdown measures in place of widely unpopular complete lockdowns.
更多查看译文
AI 理解论文
溯源树
样例
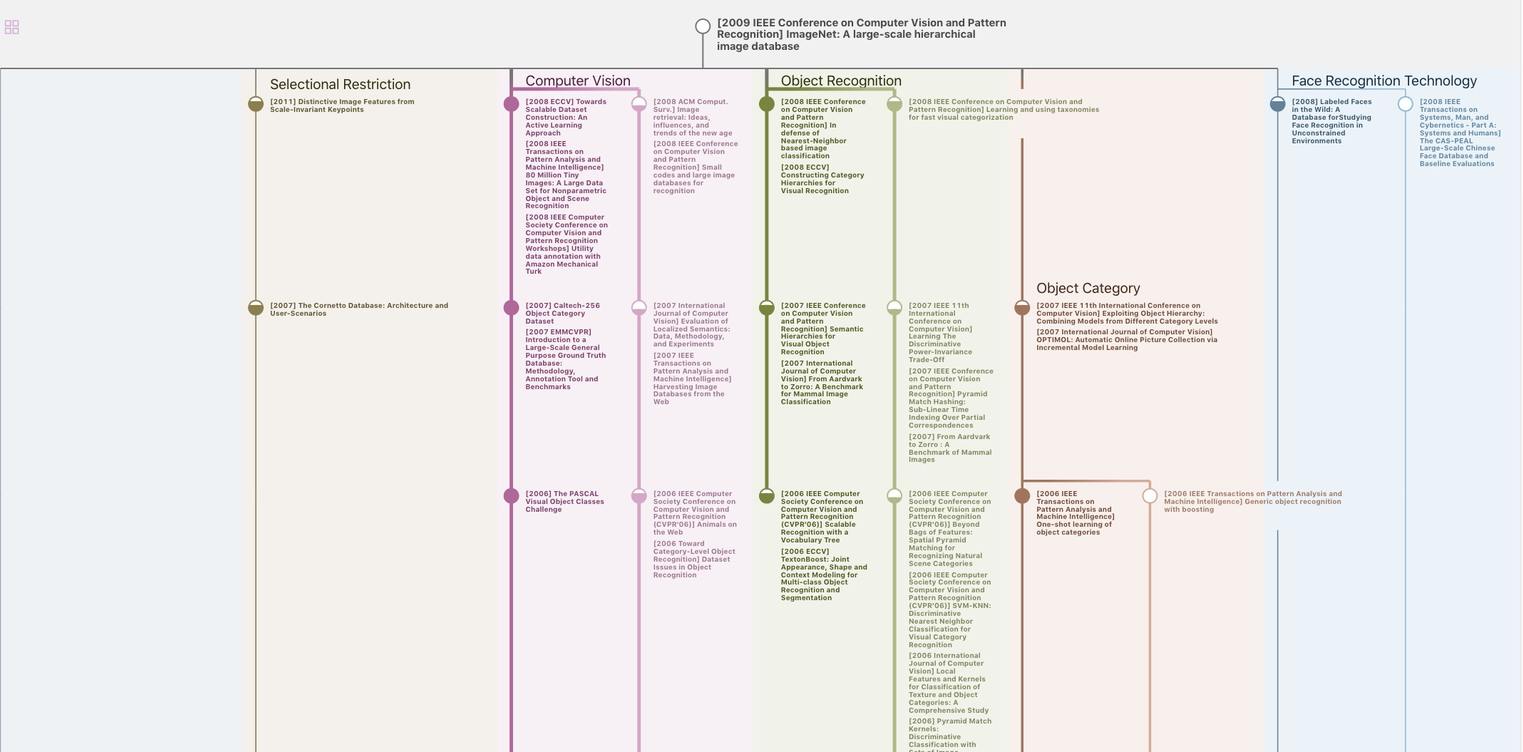
生成溯源树,研究论文发展脉络
Chat Paper
正在生成论文摘要