Privacy-Preserving Randomized Controlled Trials: A Protocol for Industry Scale Deployment
CCS(2021)
摘要
ABSTRACTRandomized Controlled Trials, when feasible, give the strongest and most trustworthy empirical measures of causal effects. They are the gold standard in many clinical, social, and behavioral fields of study. However, the most important settings often involve the most sensitive data, therefore cause privacy concerns. In this paper, we outline a way to deploy an end-to-end privacy-preserving protocol for learning causal effects from Randomized Controlled Trials (RCTs). We are particularly focused on the difficult and important case where one party determines which treatment an individual receives, and another party measures outcomes on individuals, and these parties do not want to leak any of their information to each other, but still want to collectively learn a true causal effect in the world. Moreover, we show how such a protocol can be scaled to 500 million rows of data and more than a billion gates. We also offer an open source deployment of this protocol. We accomplish this by a three-stage solution, interconnecting and blending three privacy technologies--private set intersection, multiparty computation, and differential privacy--to address core points of privacy leakage, at the join, at the point of computation, and at the release, respectively. The first stage uses the Private-ID protocol[8] to create a private encrypted join of the users. The second stage utilizes the encrypted join to run multiple instances of a general purpose MPC over a sharded database to aggregate statistics about each experimental group while discarding individuals who took an action before they received treatment. The third stage adds distributed and calibrated Differential Privacy (DP) noise within the final MPC computations to the released aggregate statistical estimates of causal effects and their uncertainty measures, providing formal two-sided privacy guarantees. We also evaluate the performance of multiple open source general purpose MPC libraries for this task. We additionally demonstrate how we have used this to create a working ads effectiveness measurement product capable of measuring hundreds of millions of individuals per experiment.
更多查看译文
关键词
industry scale deployment,privacy-preserving
AI 理解论文
溯源树
样例
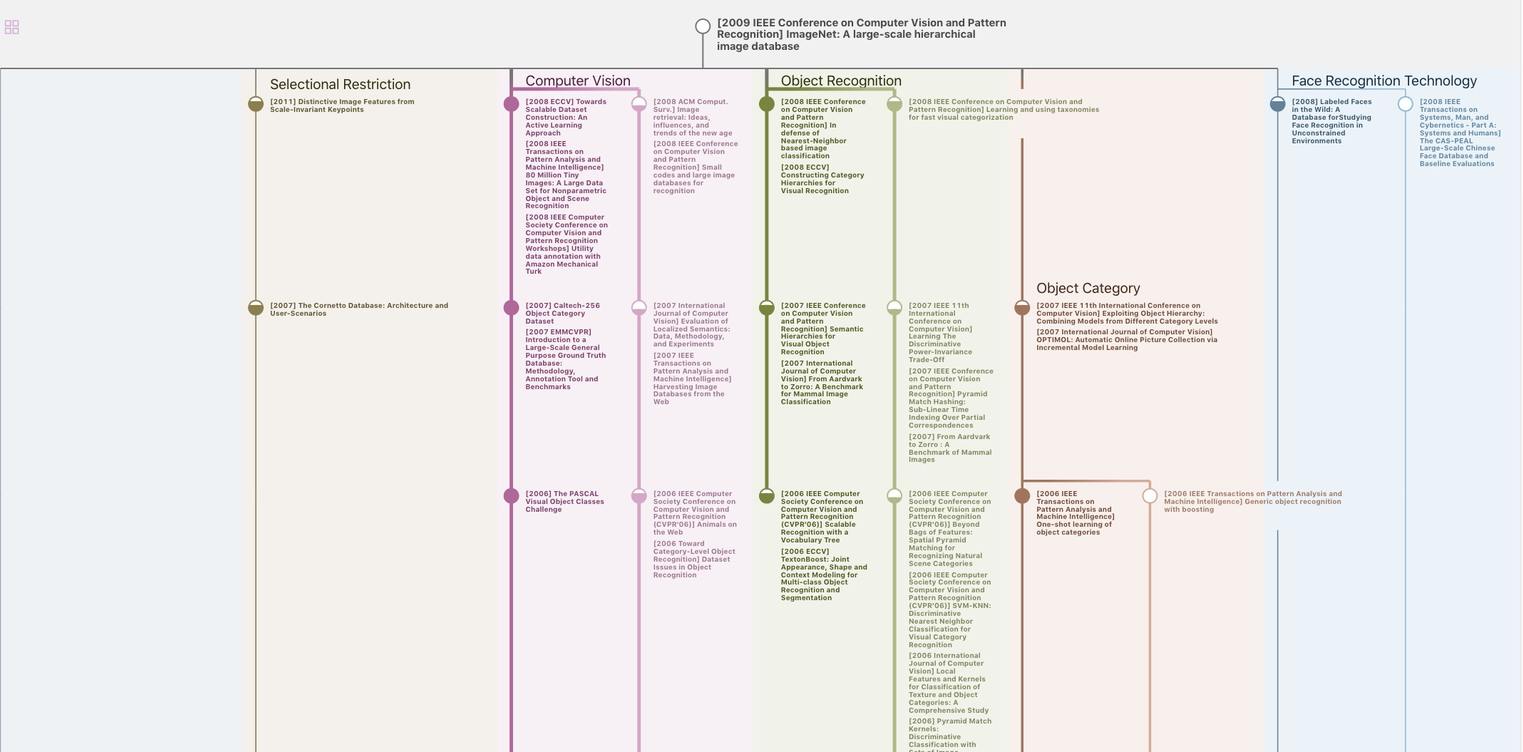
生成溯源树,研究论文发展脉络
Chat Paper
正在生成论文摘要