A Scalable Approach for Distributed Reasoning over Large-scale OWL Datasets.
KEOD(2021)
摘要
With the tremendous increase in the volume of semantic data on the Web, reasoning over such an amount of data has become a challenging task. On the other hand, the traditional centralized approaches are no longer feasible for large-scale data due to the limitations of software and hardware resources. Therefore, horizontal scalability is desirable. We develop a scalable distributed approach for RDFS and OWL Horst Reasoning over large-scale OWL datasets. The eminent feature of our approach is that it combines an optimized execution strategy, pre-shuffling method, and duplication elimination strategy. thus achieving an efficient distributed reasoning mechanism. We implemented our approach as open-source in Apache Spark using Resilient Distributed Datasets (RDD) as a parallel programming model. As a use case, our approach is used by the SANSA framework for large-scale semantic reasoning over OWL datasets. The evaluation results have shown the strength of the proposed approach for both data and node scalability.
更多查看译文
关键词
Big Data,Distributed Computing,In-Memory Computation,Parallel Reasoning,OWL Horst Rules,OWL Axioms
AI 理解论文
溯源树
样例
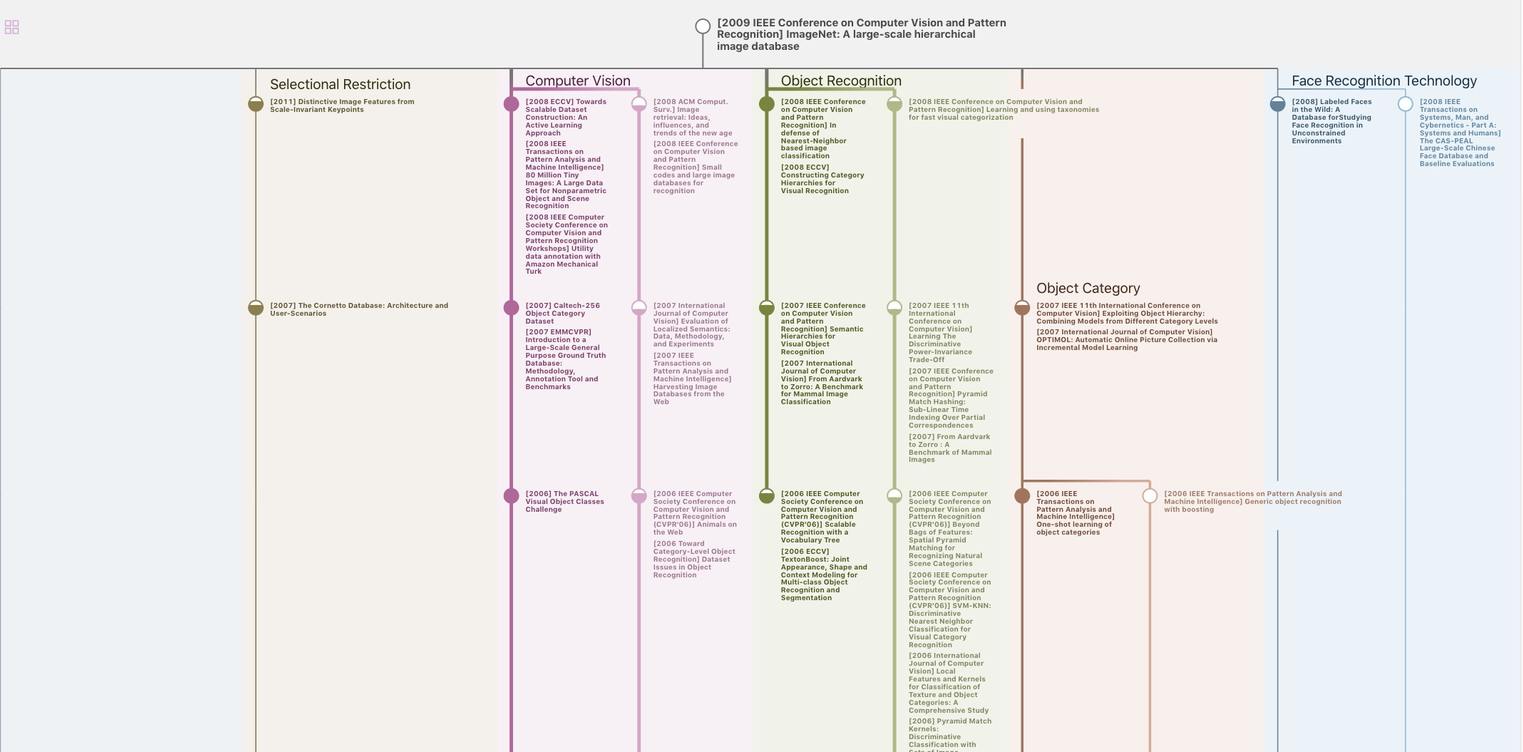
生成溯源树,研究论文发展脉络
Chat Paper
正在生成论文摘要