Explainability and Continuous Learning with Capsule Networks.
KDIR(2021)
摘要
Capsule networks are an emerging technique for image recognition and classification tasks with innovative approaches inspired by the human visual cortex. State of the art is that capsule networks achieve good accuracy for future image recognition tasks and are a promising approach for hierarchical data sets. In this work, it is shown that capsule networks can generate image descriptions representing detected objects in images. This visualisation in combination with reconstructed images delivers strong and easily understandable explainability regarding the decision-making process of capsule networks and leading towards trustworthy AI. Furthermore it is shown that capsule networks can be used for continuous learning utilising already learned basic geometric shapes to learn more complex objects. As shown by our experiments, our approach allows for distinct explainability making it possible to use capsule networks where explainability is required.
更多查看译文
关键词
Explainability,Continuous Learning,Capsule Networks,Data Privacy,Image Recognition
AI 理解论文
溯源树
样例
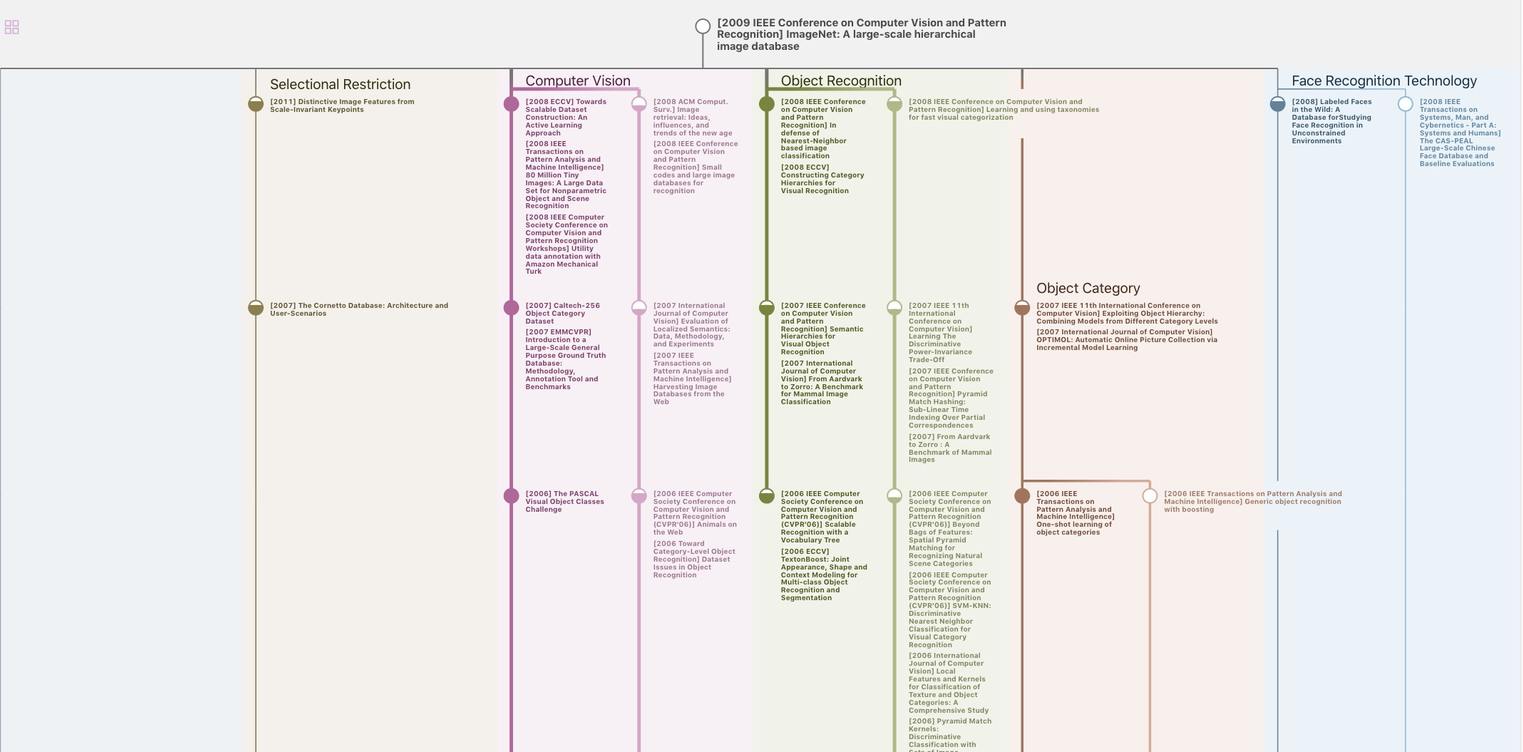
生成溯源树,研究论文发展脉络
Chat Paper
正在生成论文摘要