ISNAS-DIP: Image-Specific Neural Architecture Search for Deep Image Prior
IEEE Conference on Computer Vision and Pattern Recognition(2022)
摘要
Recent works show that convolutional neural network (CNN) architectures have a spectral bias towards lower frequencies, which has been leveraged for various image restoration tasks in the Deep Image Prior (DIP) framework. The benefit of the inductive bias the network imposes in the DIP framework depends on the architecture. Therefore, re-searchers have studied how to automate the search to de-termine the best-performing model. However, common neu-ral architecture search (NAS) techniques are resource and time-intensive. Moreover, best-performing models are de-termined for a whole dataset of images instead of for each image independently, which would be prohibitively expen-sive. In this work, we first show that optimal neural archi-tectures in the DIP framework are image-dependent. Lever-aging this insight, we then propose an image-specific NAS strategy for the DIP framework that requires substantially less training than typical NAS approaches, effectively en-abling image-specific NAS. We justify the proposed strat-egy's effectiveness by (1) demonstrating its performance on a NAS Dataset for DIP that includes 522 models from a particular search space (2) conducting extensive experi-ments on image denoising, inpainting, and super-resolution tasks. Our experiments show that image-specific metrics can reduce the search space to a small cohort of models, of which the best model outperforms current NAS approaches for image restoration. Codes and datasets are available at https://github.com/ozgurkara99/ISNAS-DIP.
更多查看译文
关键词
Low-level vision, Deep learning architectures and techniques, Image and video synthesis and generation, Self-& semi-& meta- & unsupervised learning
AI 理解论文
溯源树
样例
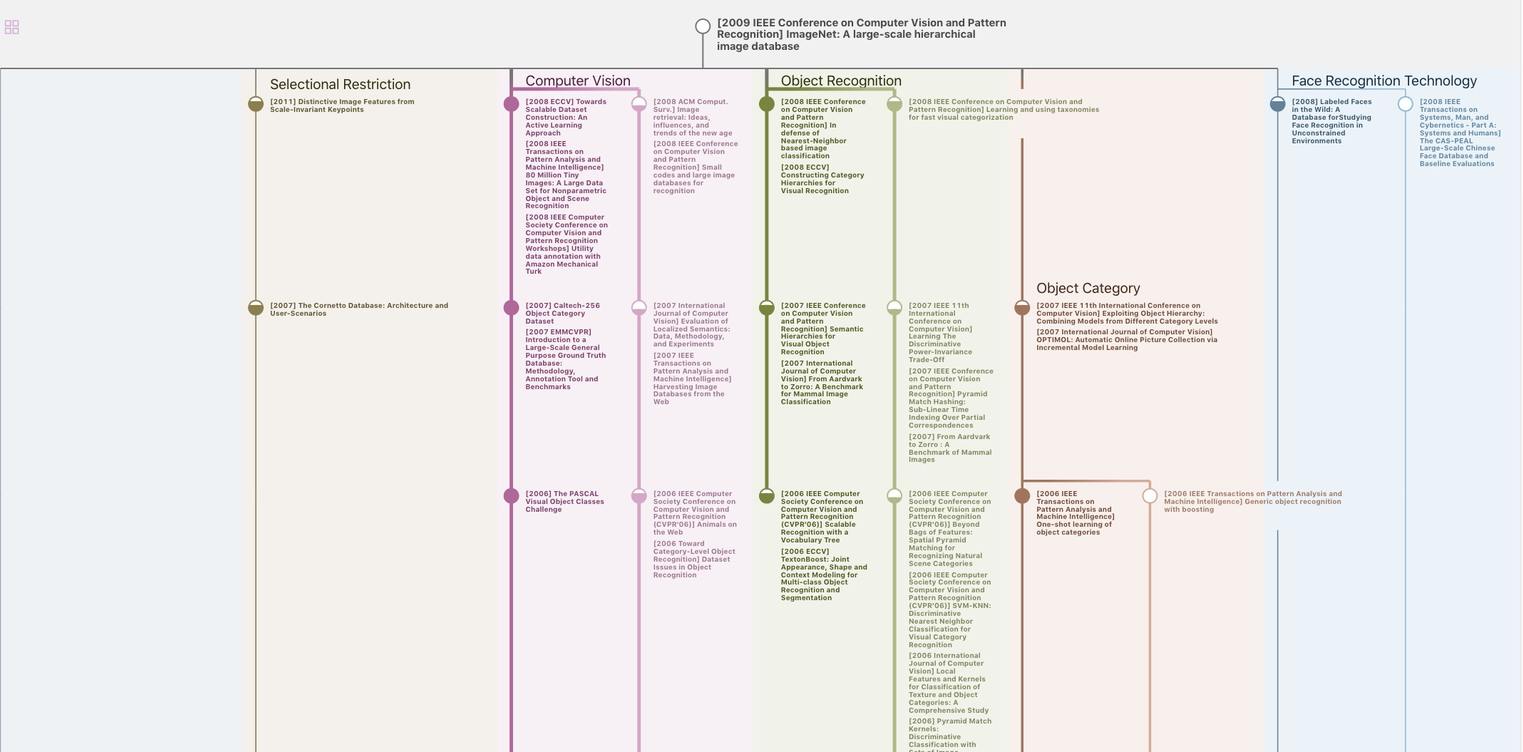
生成溯源树,研究论文发展脉络
Chat Paper
正在生成论文摘要