Self-Supervised Autoregressive Domain Adaptation for Time Series Data
IEEE TRANSACTIONS ON NEURAL NETWORKS AND LEARNING SYSTEMS(2024)
摘要
Unsupervised domain adaptation (UDA) has successfully addressed the domain shift problem for visual applications. Yet, these approaches may have limited performance for time series data due to the following reasons. First, they mainly rely on the large-scale dataset (i.e., ImageNet) for source pretraining, which is not applicable for time series data. Second, they ignore the temporal dimension on the feature space of the source and target domains during the domain alignment step. Finally, most of the prior UDA methods can only align the global features without considering the fine-grained class distribution of the target domain. To address these limitations, we propose a SeLf-supervised AutoRegressive Domain Adaptation (SLARDA) framework. In particular, we first design a self-supervised (SL) learning module that uses forecasting as an auxiliary task to improve the transferability of source features. Second, we propose a novel autoregressive domain adaptation technique that incorporates temporal dependence of both source and target features during domain alignment. Finally, we develop an ensemble teacher model to align class-wise distribution in the target domain via a confident pseudo labeling approach. Extensive experiments have been conducted on three real-world time series applications with 30 cross-domain scenarios. The results demonstrate that our proposed SLARDA method significantly outperforms the state-of-the-art approaches for time series domain adaptation. Our source code is available at: https://github.com/mohamedr002/SLARDA.
更多查看译文
关键词
Time series analysis,Feature extraction,Task analysis,Training,Stars,Visualization,Representation learning,Autoregressive domain adaptation,ensemble teacher learning,self-supervised (SL) learning,time series data
AI 理解论文
溯源树
样例
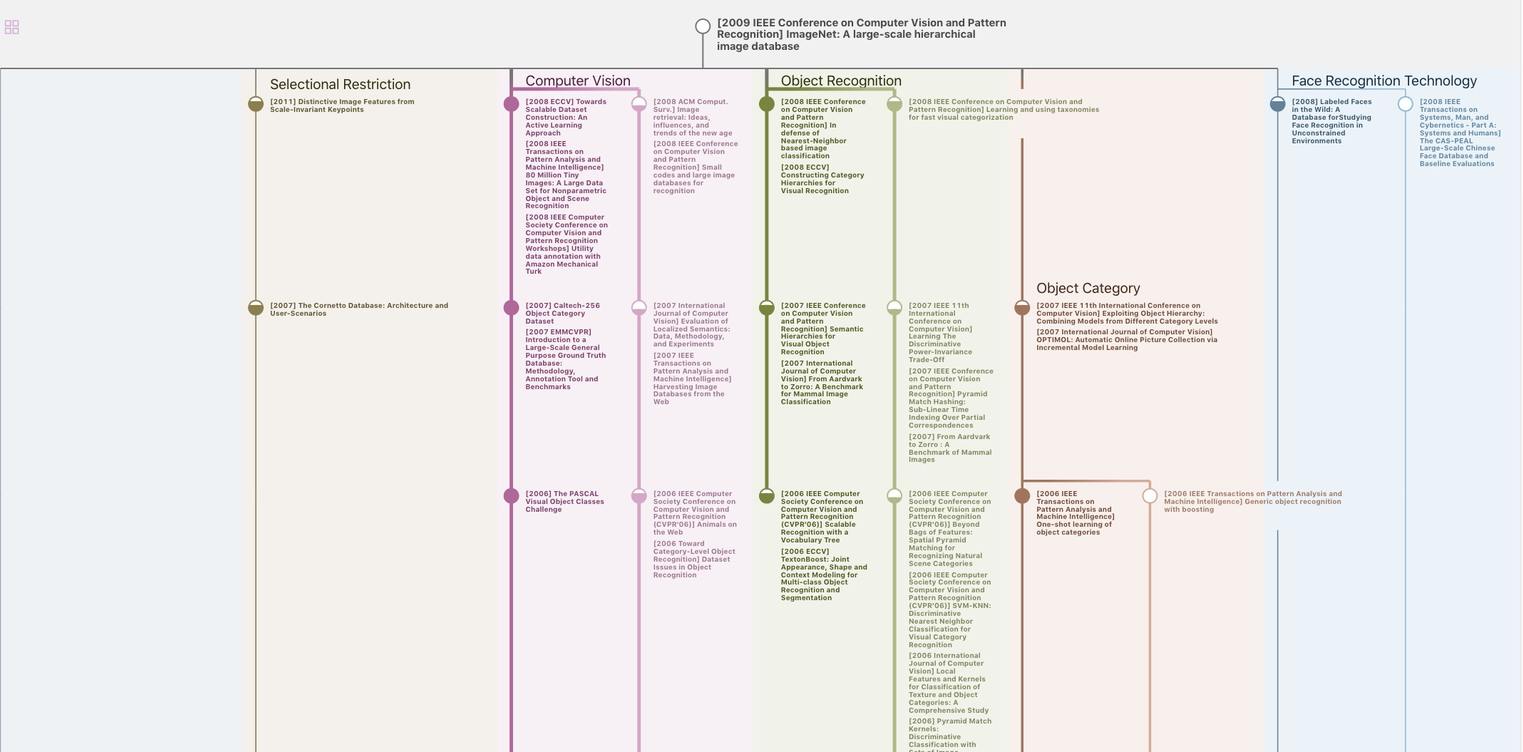
生成溯源树,研究论文发展脉络
Chat Paper
正在生成论文摘要