3D Compositional Zero-Shot Learning with DeCompositional Consensus.
European Conference on Computer Vision(2022)
摘要
Parts represent a basic unit of geometric and semantic similarity across different objects. We argue that part knowledge should be composable beyond the observed object classes. Towards this, we present 3D Compositional Zero-shot Learning as a problem of part generalization from seen to unseen object classes for semantic segmentation. We provide a structured study through benchmarking the task with the proposed Compositional-PartNet dataset. This dataset is created by processing the original PartNet to maximize part overlap across different objects. The existing point cloud part segmentation methods fail to generalize to unseen object classes in this setting. As a solution, we propose DeCompositional Consensus, which combines a part segmentation network with a part scoring network. The key intuition to our approach is that a segmentation mask over some parts should have a consensus with its part scores when each part is taken apart. The two networks reason over different part combinations defined in a per-object part prior to generate the most suitable segmentation mask. We demonstrate that our method allows compositional zero-shot segmentation and generalized zero-shot classification, and establishes the state of the art on both tasks.
更多查看译文
关键词
3D compositional zero-shot learning,Compositionality
AI 理解论文
溯源树
样例
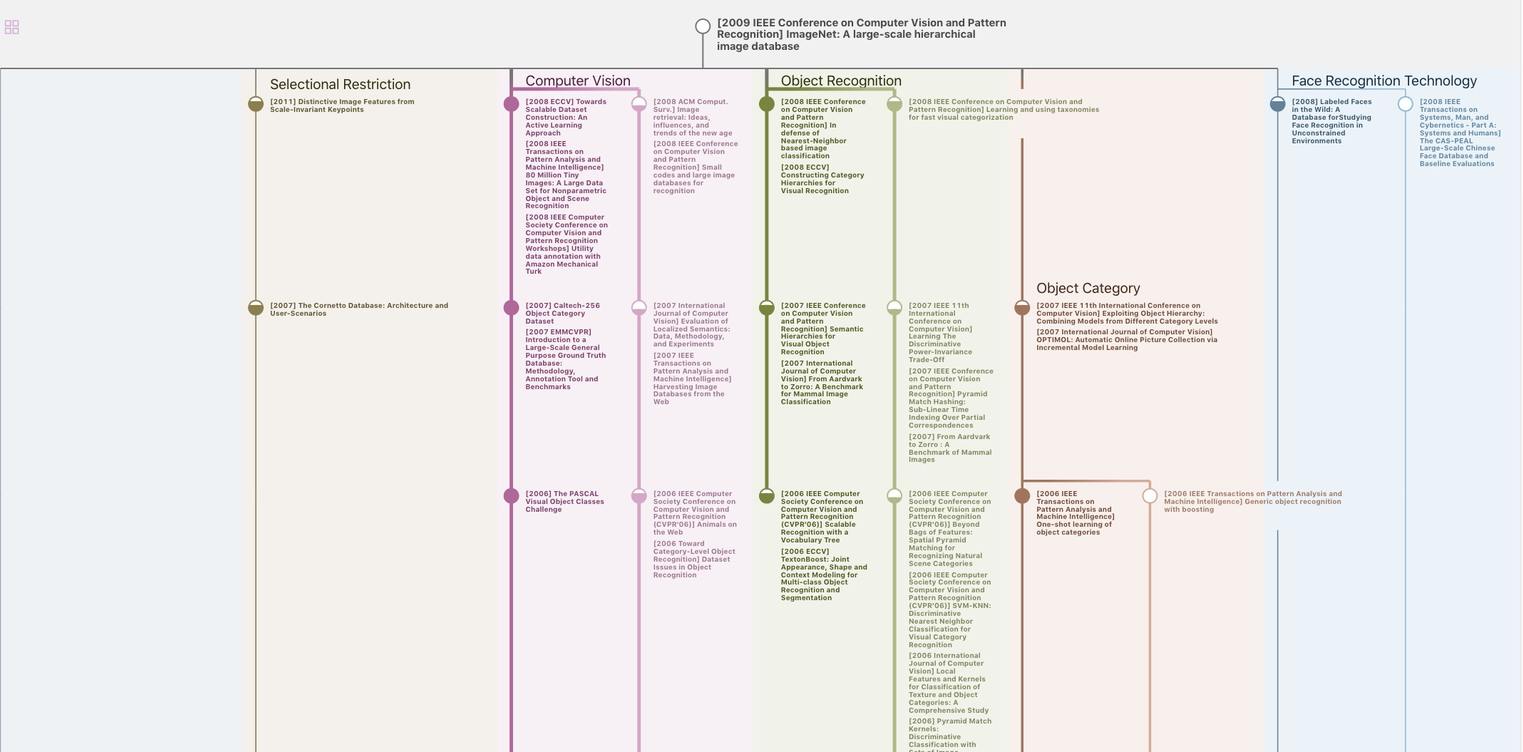
生成溯源树,研究论文发展脉络
Chat Paper
正在生成论文摘要