On Learning Domain-Invariant Representations for Transfer Learning with Multiple Sources.
Annual Conference on Neural Information Processing Systems(2021)
摘要
Domain adaptation (DA) benefits from the rigorous theoretical works that study its insightful characteristics and various aspects, e.g., learning domain-invariant representations and its trade-off. However, it seems not the case for the multiple source DA and domain generalization (DG) settings which are remarkably more complicated and sophisticated due to the involvement of multiple source domains and potential unavailability of target domain during training. In this paper, we develop novel upper-bounds for the target general loss which appeal us to define two kinds of domain-invariant representations. We further study the pros and cons as well as the trade-offs of enforcing learning each domain-invariant representation. Finally, we conduct experiments to inspect the trade-off of these representations for offering practical hints regarding how to use them in practice and explore other interesting properties of our developed theory.
更多查看译文
关键词
transfer learning,multiple sources,domain-invariant
AI 理解论文
溯源树
样例
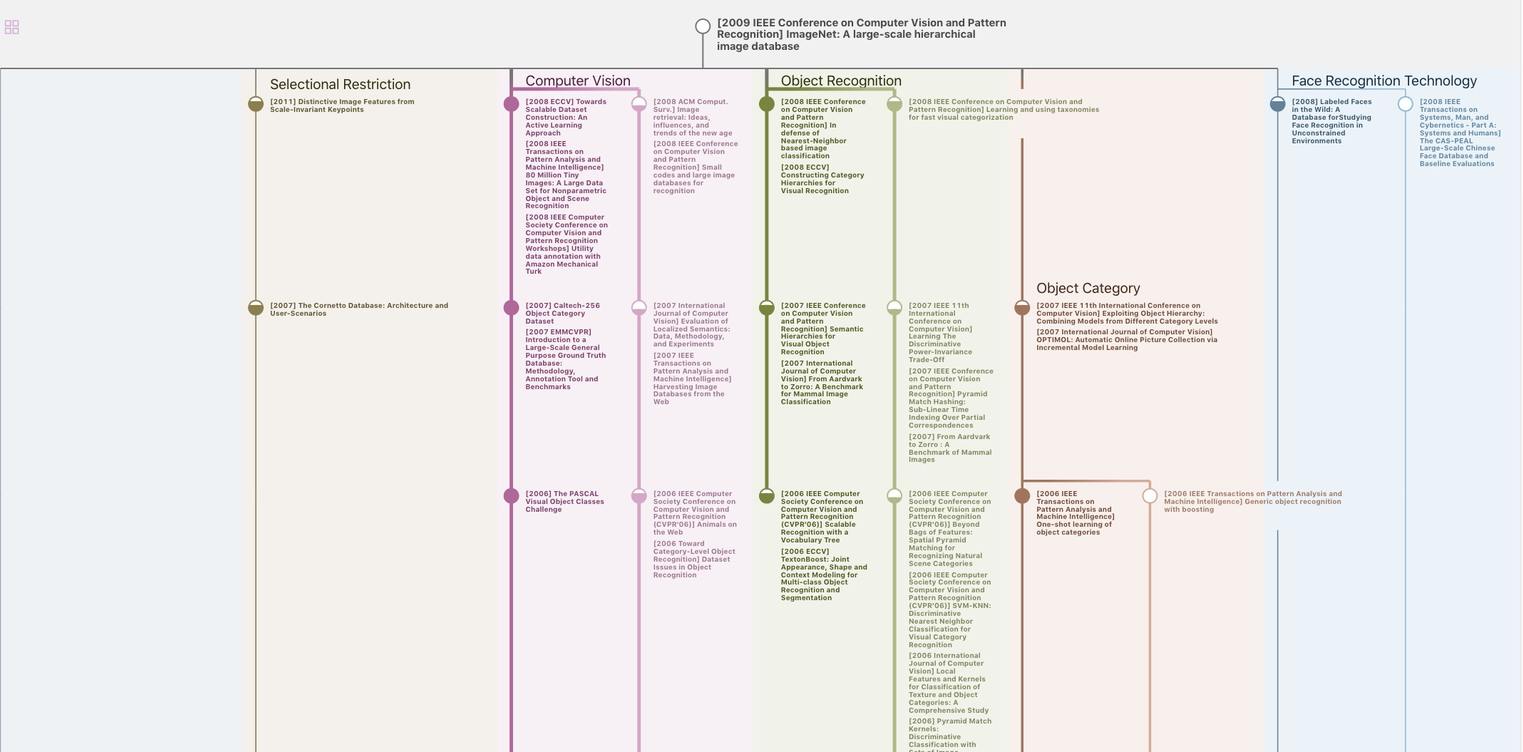
生成溯源树,研究论文发展脉络
Chat Paper
正在生成论文摘要