Learning from Temporal Gradient for Semi-supervised Action Recognition
IEEE Conference on Computer Vision and Pattern Recognition(2022)
摘要
Semi-supervised video action recognition tends to enable deep neural networks to achieve remarkable performance even with very limited labeled data. However, existing methods are mainly transferred from current image-based methods (e.g., FixMatch). Without specifically utilizing the temporal dynamics and inherent multimodal attributes, their results could be suboptimal. To better leverage the encoded temporal information in videos, we introduce temporal gradient as an additional modality for more attentive feature extraction in this paper. To be specific, our method explicitly distills the fine-grained motion representations from temporal gradient (TG) and imposes consistency across different modalities (i.e., RGB and TG). The performance of semi-supervised action recognition is significantly improved without additional computation or parameters during inference. Our method achieves the state-of-the-art performance on three video action recognition benchmarks (i.e., Kinetics-400, UCF-101, and HMDB-51) under several typical semi-supervised settings (i.e., different ratios of labeled data). Code is made available at https://github.com/lambert-x/video-semisup.
更多查看译文
关键词
Video analysis and understanding
AI 理解论文
溯源树
样例
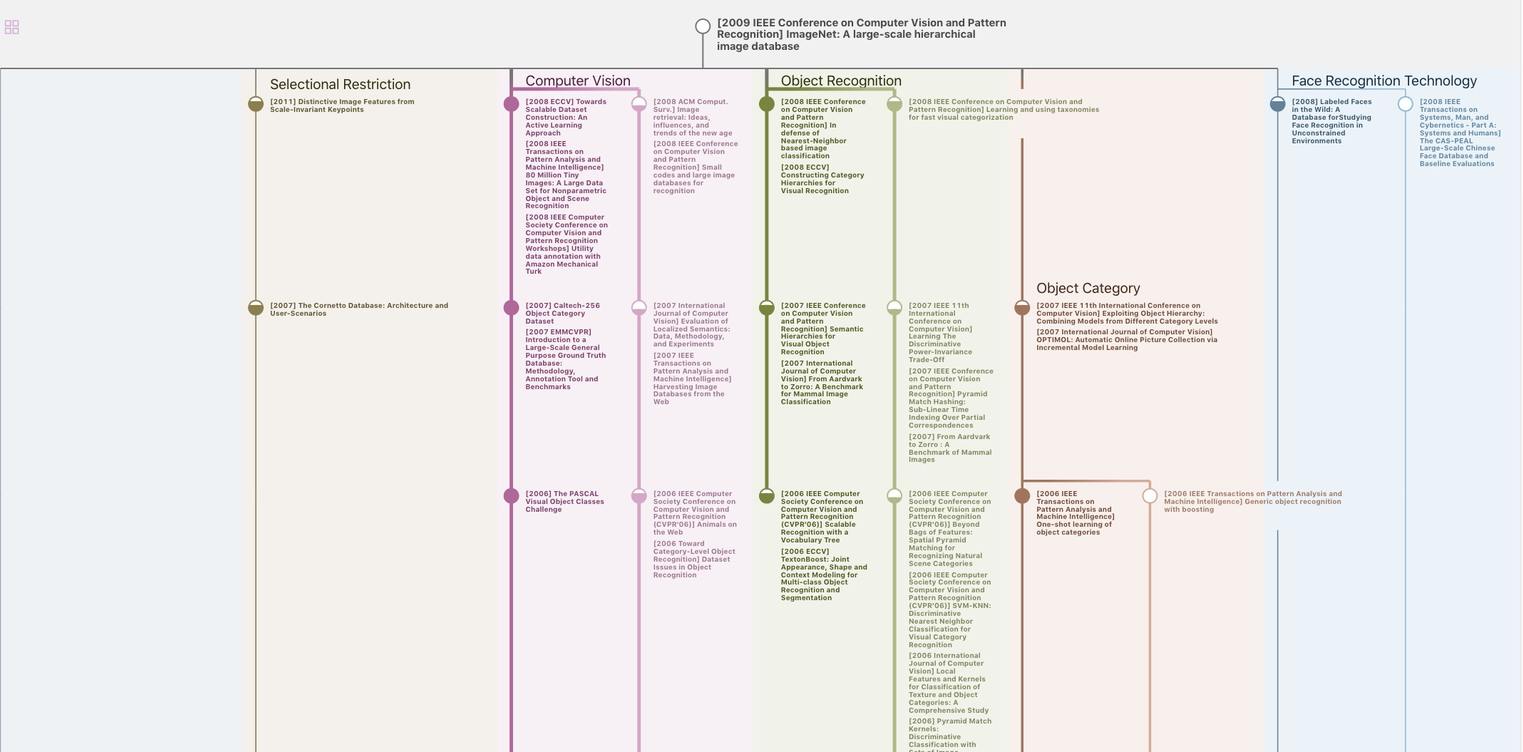
生成溯源树,研究论文发展脉络
Chat Paper
正在生成论文摘要