Deep Graph Convolutional Networks with Hybrid Normalization for Accurate and Diverse Recommendation
user-613ea93de55422cecdace10f(2021)
摘要
Collaborative filtering (CF) methods based on Graph Convolutional Networks (GCNs) have attracted great research interests and achieved the state-of-the-art performance in recent years. However, all the existing GCN-based CF models achieve their best performance with shallow layers, which fail to model higher-order collaborative signal. Besides, most GCN-based CF models utilize the same normalization rule for neighbor information aggregation, leading a popularity-related importance (symmetric normalization) or equal importance (left normalization) for neighbors. Due to the intrinsic differences over nodes, different normalization rules are suitable for them to aggregate neighbor information. In this work, we propose a novel Deep Graph Convolutional Network with Hybrid Normalization (DGCN-HN) to alleviate the above limitations. First, a deep graph convolutional network for recommendation is designed which consists of residual connection and holistic connection to alleviate the over-smoothing problem. It allows the effective training of GCN with deeper layers. Then, a hybrid normalization layer and a simplified attention network are proposed to flexibly model the importance of neighbors by adaptively incorporating information from different normalization rules. Comprehensive experiments on three real-world datasets show that DGCN-HN achieves the best accuracy against the state-of-the-art methods (12.12% and 12.77% relative improvement on average in terms of Recall@20 and NDCG@20). Further, we conduct a diversity study compared with the best baseline. It demonstrates that our proposed solution can achieve more diverse recommendation results. Besides, in our extensive case study, we show that our proposed method can benefit more for users having few interaction history, which can be used for alleviate the data sparsity problem.
更多查看译文
AI 理解论文
溯源树
样例
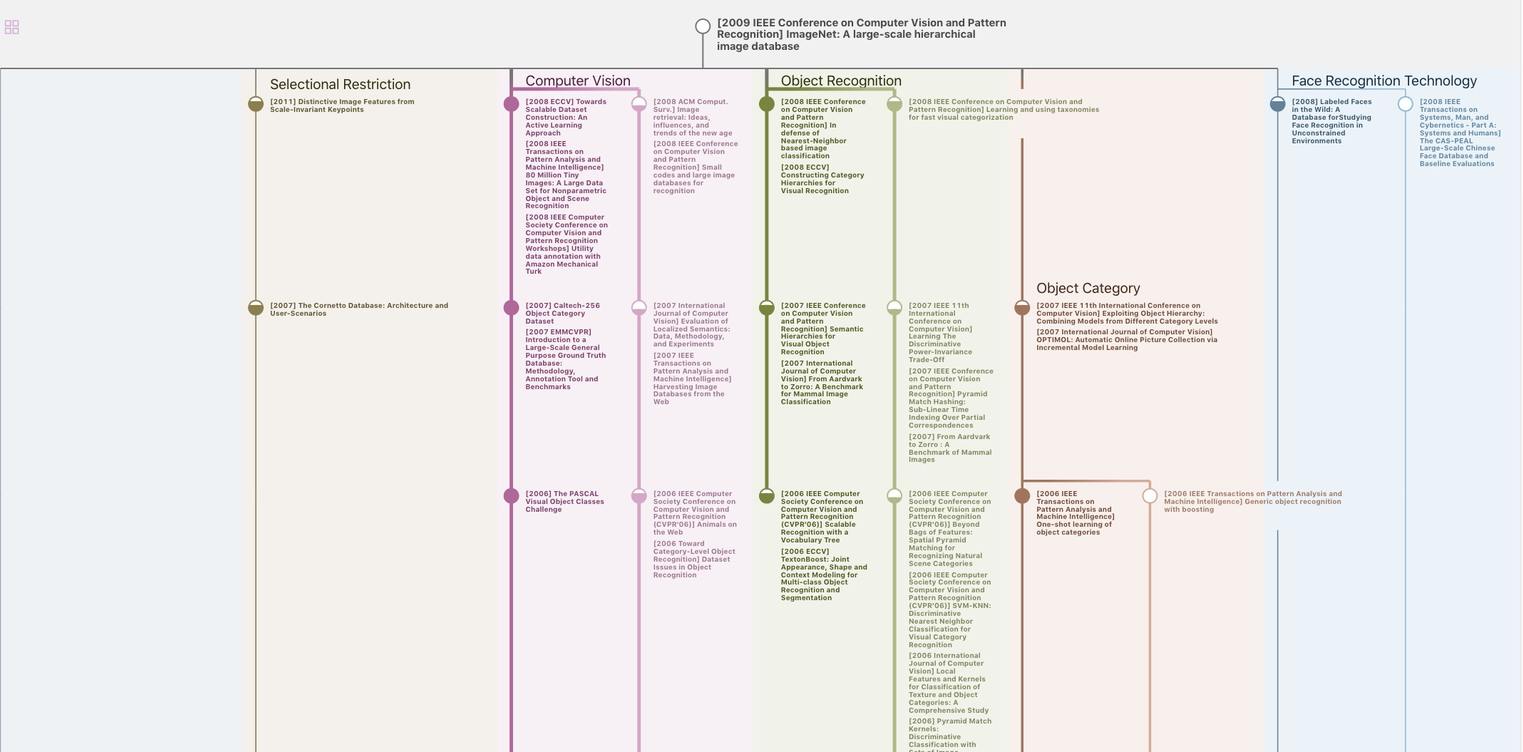
生成溯源树,研究论文发展脉络
Chat Paper
正在生成论文摘要