Status-quo policy gradient in Multi-Agent Reinforcement Learning
CoRR(2021)
摘要
Individual rationality, which involves maximizing expected individual returns, does not always lead to high-utility individual or group outcomes in multi-agent problems. For instance, in multi-agent social dilemmas, Reinforcement Learning (RL) agents trained to maximize individual rewards converge to a low-utility mutually harmful equilibrium. In contrast, humans evolve useful strategies in such social dilemmas. Inspired by ideas from human psychology that attribute this behavior to the status-quo bias, we present a status-quo loss (SQLoss) and the corresponding policy gradient algorithm that incorporates this bias in an RL agent. We demonstrate that agents trained with SQLoss learn high-utility policies in several social dilemma matrix games (Prisoner's Dilemma, Stag Hunt matrix variant, Chicken Game). We show how SQLoss outperforms existing state-of-the-art methods to obtain high-utility policies in visual input non-matrix games (Coin Game and Stag Hunt visual input variant) using pre-trained cooperation and defection oracles. Finally, we show that SQLoss extends to a 4-agent setting by demonstrating the emergence of cooperative behavior in the popular Braess' paradox.
更多查看译文
关键词
Reinforcement learning,Game theory,Social dilemma,Rationality,Status quo,Artificial intelligence,Computer science,Contrast (statistics),Sequence,Group outcomes
AI 理解论文
溯源树
样例
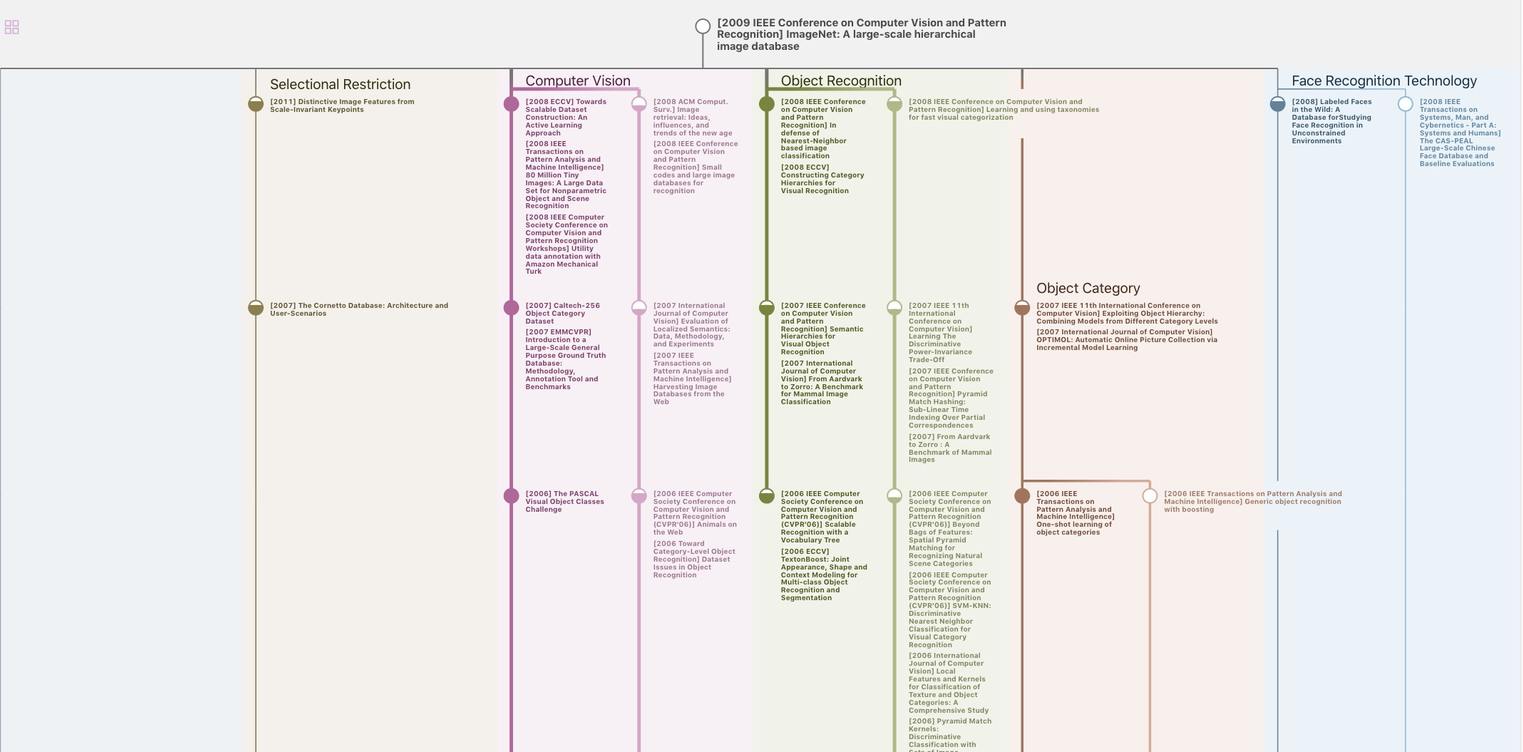
生成溯源树,研究论文发展脉络
Chat Paper
正在生成论文摘要