CamLiFlow: Bidirectional Camera-LiDAR Fusion for Joint Optical Flow and Scene Flow Estimation
IEEE Conference on Computer Vision and Pattern Recognition(2022)
摘要
In this paper, we study the problem of jointly estimating the optical flow and scene flow from synchronized 2D and 3D data. Previous methods either employ a complex pipeline that splits the joint task into independent stages, or fuse 2D and 3D information in an “early-fusion“ or “late-fusion“ manner. Such one-size-fits-all approaches suffer from a dilemma of failing to fully utilize the characteristic of each modality or to maximize the inter-modality complementarity. To address the problem, we propose a novel end-to-end framework, called CamLiFlow. It consists of 2D and 3D branches with multiple bidirectional connections between them in specific layers. Different from previous work, we apply a point-based 3D branch to better extract the geometric features and design a symmetric learnable operator to fuse dense image features and sparse point features. Experiments show that CamLiFlow achieves better performance with fewer parameters. Our method ranks 1st on the KITTI Scene Flow benchmark, outperforming the previous art with 1/7 parameters. Code is available at https://github.com/MCG-NJU/CamLiFlow.
更多查看译文
关键词
Low-level vision, 3D from multi-view and sensors, Motion and tracking, Navigation and autonomous driving, Scene analysis and understanding
AI 理解论文
溯源树
样例
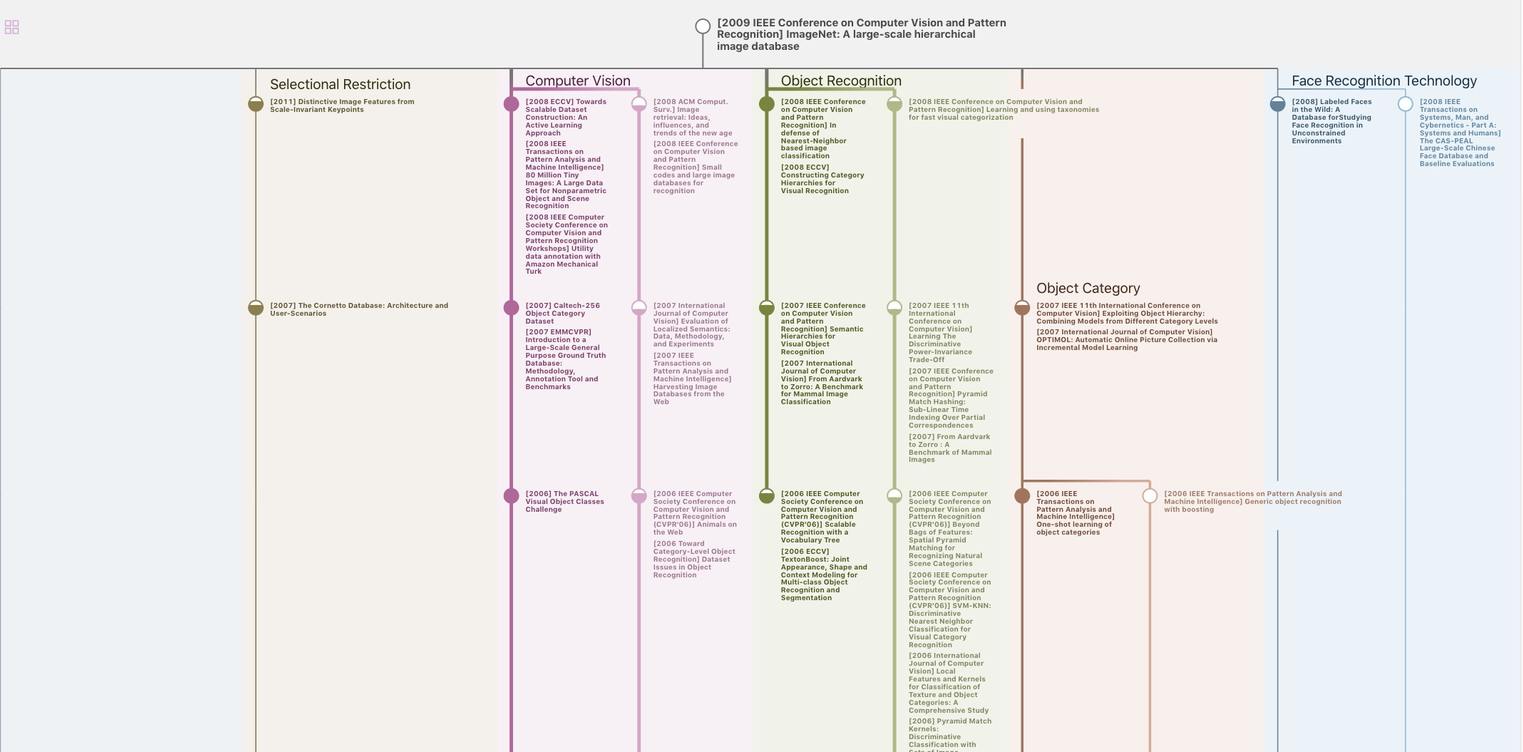
生成溯源树,研究论文发展脉络
Chat Paper
正在生成论文摘要