What Stops Learning-based 3D Registration from Working in the Real World?
arxiv(2021)
摘要
Much progress has been made on the task of learning-based 3D point cloud registration, with existing methods yielding outstanding results on standard benchmarks, such as ModelNet40, even in the partial-to-partial matching scenario. Unfortunately, these methods still struggle in the presence of real data. In this work, we identify the sources of these failures, analyze the reasons behind them, and propose solutions to tackle them. We summarise our findings into a set of guidelines and demonstrate their effectiveness by applying them to different baseline methods, DCP and IDAM. In short, our guidelines improve both their training convergence and testing accuracy. Ultimately, this translates to a best-practice 3D registration network (BPNet), constituting the first learning-based method able to handle previously-unseen objects in real-world data. Despite being trained only on synthetic data, our model generalizes to real data without any fine-tuning, reaching an accuracy of up to 67% on point clouds of unseen objects obtained with a commercial sensor.
更多查看译文
关键词
3d registration,learning-based
AI 理解论文
溯源树
样例
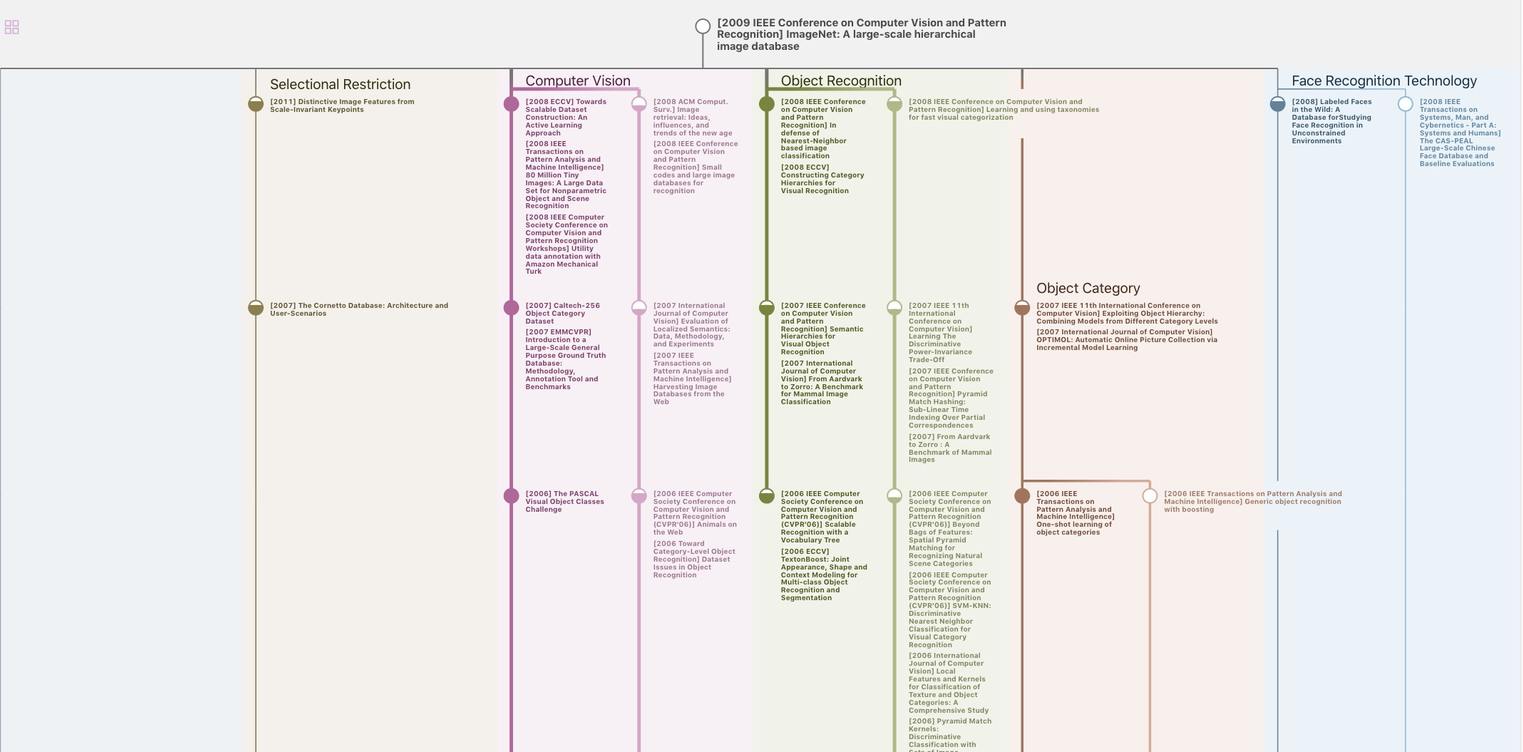
生成溯源树,研究论文发展脉络
Chat Paper
正在生成论文摘要