Traversability Mapping In Off-Road Environment Using Semantic Segmentation
AUTONOMOUS SYSTEMS: SENSORS, PROCESSING, AND SECURITY FOR VEHICLES AND INFRASTRUCTURE 2021(2021)
摘要
Autonomous driving in off-road environments is challenging as it does not have a definite terrain structure. Assessment of terrain traversability is the main factor in deciding the autonomous driving capability of the ground vehicle. Traversability in off-road environments can be defined as the drivable locations which differs based on the type of vehicle. It is very crucial for the autonomous ground vehicle (AGV) to avoid obstacles such as trees, boulders etc. while traversing through the trails. A key requirement in developing autonomous navigation algorithms is the availability of labeled data to train and test the machine learning algorithms. The labeled two-dimensional camera and threedimensional LiDAR datasets which are available at present are almost exclusively for structured on-road environments. There are few labeled off-road datasets available and there is a need for producing these off-road labeled data for different applications. The goal of this research has three main objectives: a) collection of 2D camera data in the off-road and unstructured environment, b) annotation of 2D camera data depending on the vehicles' ability to drive through the trails, and c) application of semantic segmentation algorithm on the labeled dataset to predict the trajectory based on the type of ground vehicle. The ground vehicles considered in this work are a sedan, a pickup truck, and a military vehicle (for example HMMWV). The data are collected at the Center for Advanced Vehicular Systems (CAVS) proving grounds during different seasons of the year. Our models and labeled datasets will be publicly available.
更多查看译文
关键词
autonomous ground vehicle, traversability, off-road environment, deep learning, semantic segmentation
AI 理解论文
溯源树
样例
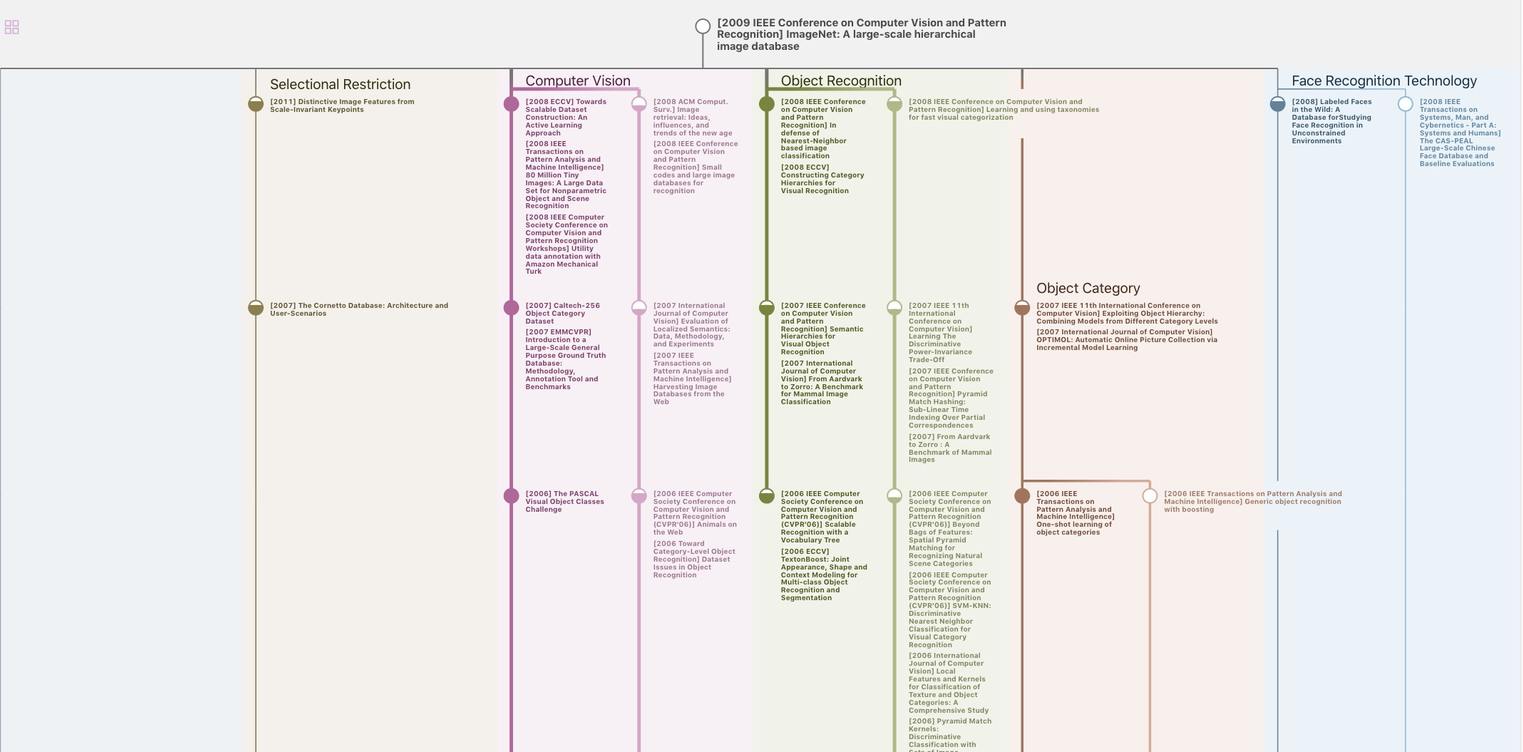
生成溯源树,研究论文发展脉络
Chat Paper
正在生成论文摘要