Longevity-Conscious Energy Management Strategy Of Fuel Cell Hybrid Electric Vehicle Based On Deep Reinforcement Learning
ENERGY(2022)
摘要
Deep reinforcement learning-based energy management strategy play an essential role in improving fuel economy and extending fuel cell lifetime for fuel cell hybrid electric vehicles. In this work, the traditional Deep Q-Network is compared with the Deep Q-Network with prioritized experience replay. Furthermore, the Deep Q-Network with prioritized experience replay is designed for energy management strategy to minimize hydrogen consumption and compared with the dynamic programming. Moreover, the fuel cell system degradation is incorporated into the objective function, and a balance between fuel economy and fuel cell system degradation is achieved by adjusting the degradation weight and the hydrogen consumption weight. Finally, the combined driving cycle is selected to further verify the effectiveness of the proposed strategy in unfamiliar driving environments and untrained situations. The training results under UDDS show that the fuel economy of the EMS decreases by 0.53 % when fuel cell system degradation is considered, reaching 88.73 % of the DP-based EMS in the UDDS, and the degradation of fuel cell system is effectively suppressed. At the same time, the computational efficiency is improved by more than 70 % compared to the DP-based strategy. (c) 2021 Elsevier Ltd. All rights reserved.
更多查看译文
关键词
Energy management strategy, Deep reinforcement learning, Fuel cell hybrid electric vehicles, DQN algorithm, Prioritized experience replay, Degradation
AI 理解论文
溯源树
样例
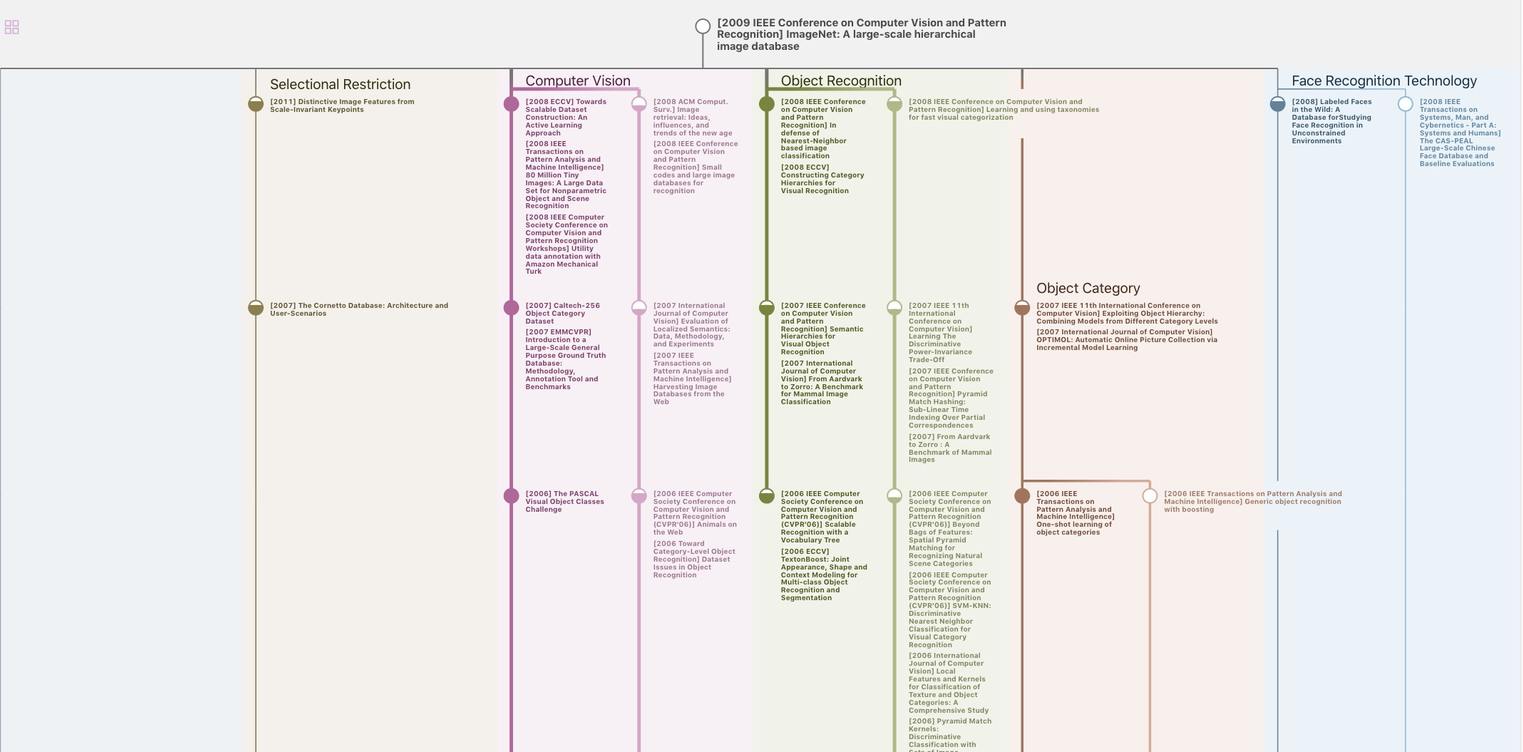
生成溯源树,研究论文发展脉络
Chat Paper
正在生成论文摘要