Automated Pixel-Level Pavement Distress Detection Based On Stereo Vision And Deep Learning
AUTOMATION IN CONSTRUCTION(2021)
摘要
Automated pavement distress detection based on 2D images is facing various challenges. To efficiently complete the crack and pothole segmentation in a practical environment, an automated pixel-level pavement distress detection framework integrating stereo vision and deep learning is developed in this study. Based on the multi-view stereo imaging system, multi-feature pavement image datasets containing color images, depth images and color-depth overlapped images are established, providing a new perspective for deep learning. To alleviate computational burden, a modified U-net deep learning architecture introducing depthwise separable convolution is proposed for crack and pothole segmentation. These methods are tested in asphalt roads with different cir-cumstances. The results show that the 3D pavement image achieves millimeter-level accuracy. The enhanced 3D crack segmentation model outperforms other models in terms of segmentation accuracy and inference speed. After obtaining the high-resolution pothole segmentation map, the automated pothole volume measurement is realized with high accuracy.
更多查看译文
关键词
Pavement distress detection, Stereo vision, Deep learning, U-net, Depthwise separable convolution, Crack and pothole segmentation
AI 理解论文
溯源树
样例
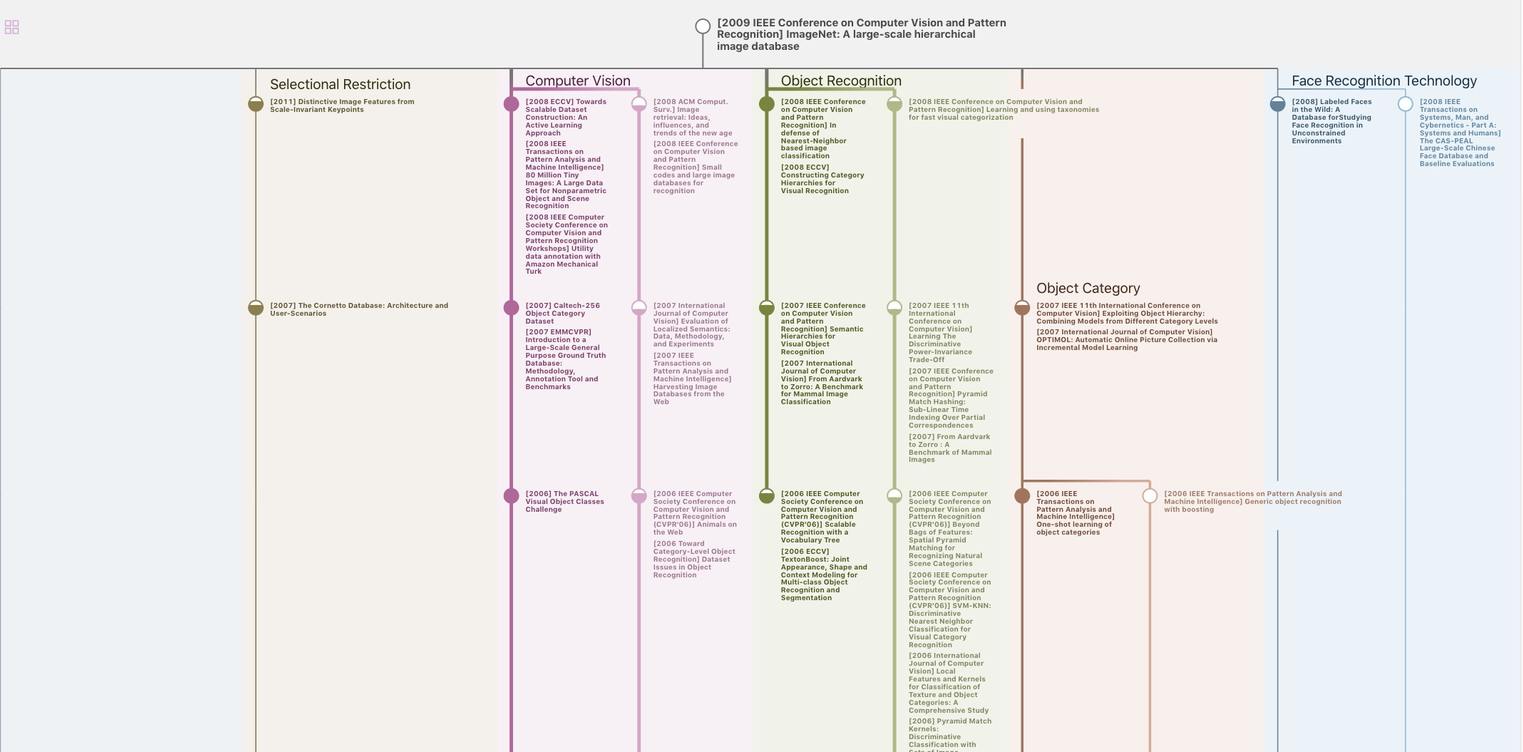
生成溯源树,研究论文发展脉络
Chat Paper
正在生成论文摘要