Deep Bayesian Sparse Subspace Clustering
IEEE SIGNAL PROCESSING LETTERS(2021)
摘要
Sparse subspace clustering, as one of the most effective subspace clustering method, is widely studied in the data processing realm. However, conventional sparse subspace clustering methods are organized with two separated steps, feature learning and indicator learning. This makes the algorithm suffer the difficulties that: (1) representation and cluster indicator learning cannot affect each other; (2) cluster number and sparse penalty coefficient should be specified a priori; (3) sparse subspace clustering method is designed for the linear subspace data and cannot be exploited in the general clustering task. In this letter, a novel sparse clustering method is proposed, in which we extend the conventional algebraic sparse subspace clustering approach to a Bayesian framework. Then, cluster number estimation and low rank constraint are coupled via a Dirichlet process parameter generation process, in which the rank are no more required to be low but can be generated with a suitable value. Finally, Generative Adversarial Network (GAN) is incorporated into the Bayesian sparse model, which extends the subspace clustering method to a normal clustering model. Experiments on different real world datasets validate our theory analysis and demonstrate the effective of the proposed algorithm.
更多查看译文
关键词
Bayes methods, Clustering methods, Clustering algorithms, Task analysis, Laplace equations, Kernel, Inference algorithms, Sparse subspace clustering, Dirichlet process, variational inference, Bayesian learning
AI 理解论文
溯源树
样例
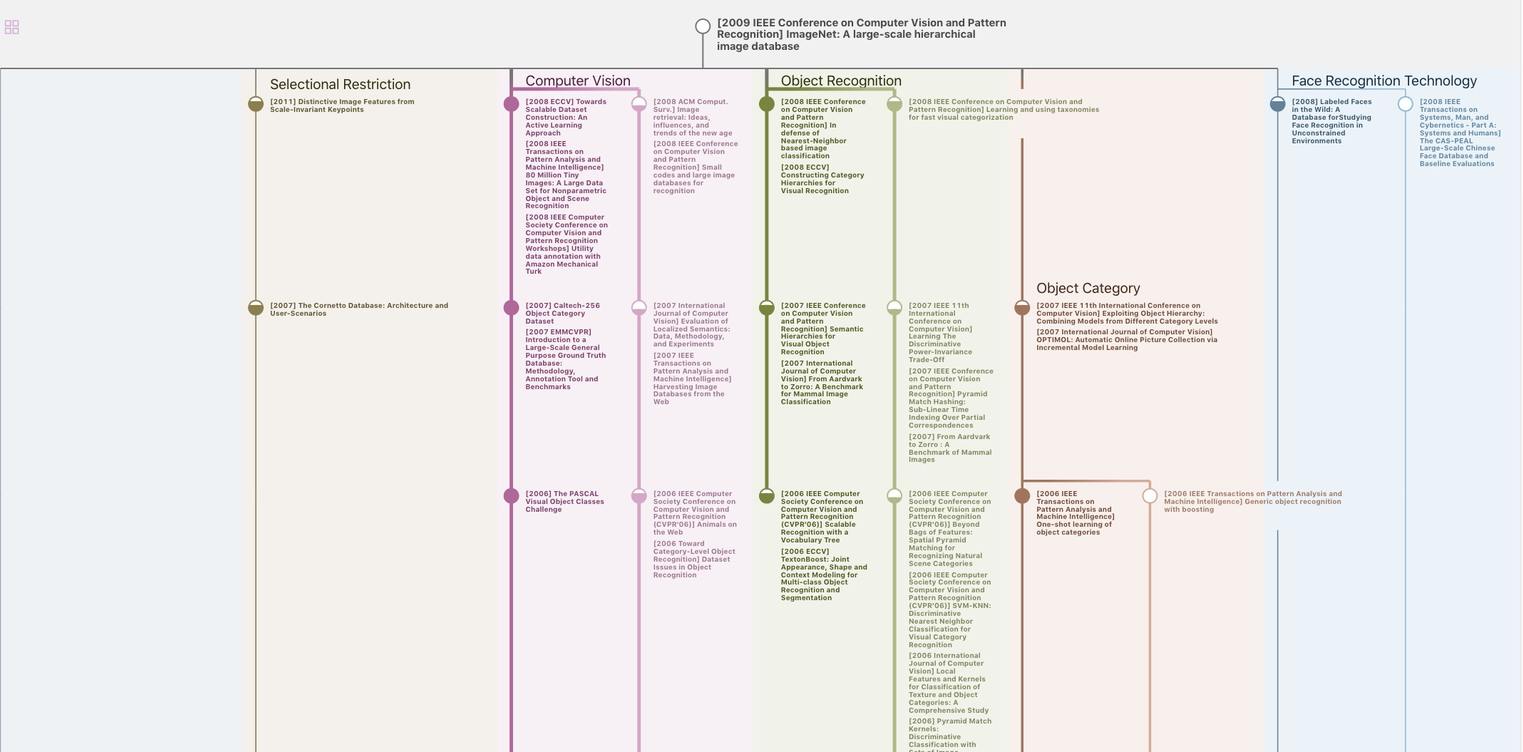
生成溯源树,研究论文发展脉络
Chat Paper
正在生成论文摘要