Pew: Prediction-Based Early Dark Cores Wake-Up Using Online Ridge Regression For Many-Core Systems
IEEE ACCESS(2021)
摘要
Future many-core systems need to address the dark silicon problem, where some cores would be turned off to control the chip's thermal and power density, which effectively limits the performance gain from having a large number of processing cores. Task migration technique has been previously proposed to improve many-core system performance by moving tasks between active and dark cores. As task migration imposes system performance overhead due to the large wake-up latency of the dark cores, this paper proposes a prediction-based early wake-up (PEW) to reduce the dark cores' wake-up latency during task migration. A window-based online ridge regression (RR) is used as the prediction model. The prediction model uses the past window's thermal, power, and core status (i.e., active or dark) to predict the future core temperatures at run-time. If task migration is predicted in the next control period, the proposed PEW puts the dark cores in a power state with low wake-up latency. Thus, the proposed PEW reduces the time for the dark cores to start executing the tasks. The comparison results show that our proposed PEW reduces the completion time by up to 7.9% and 4.1% compared to non-early wake-up (NoEW) and a fixed threshold wake-up (FEW), respectively. It also shows that the proposed PEW increases the MIPS/Watt by up to 5.5% and 2.3% over NoEW and FEW, respectively. These results show that the proposed PEW improves the many-core system's overall performance in terms of reducing dark cores' wake-up latency and increasing the number of executed instructions per Watt.
更多查看译文
关键词
Task analysis, Silicon, System performance, Predictive models, Optimization, Transient analysis, Thermal management, Dark silicon, many-core systems, task migration, dynamic voltage frequency scaling (DVFS), ridge regression, early wake-up, dark core wake-up latency
AI 理解论文
溯源树
样例
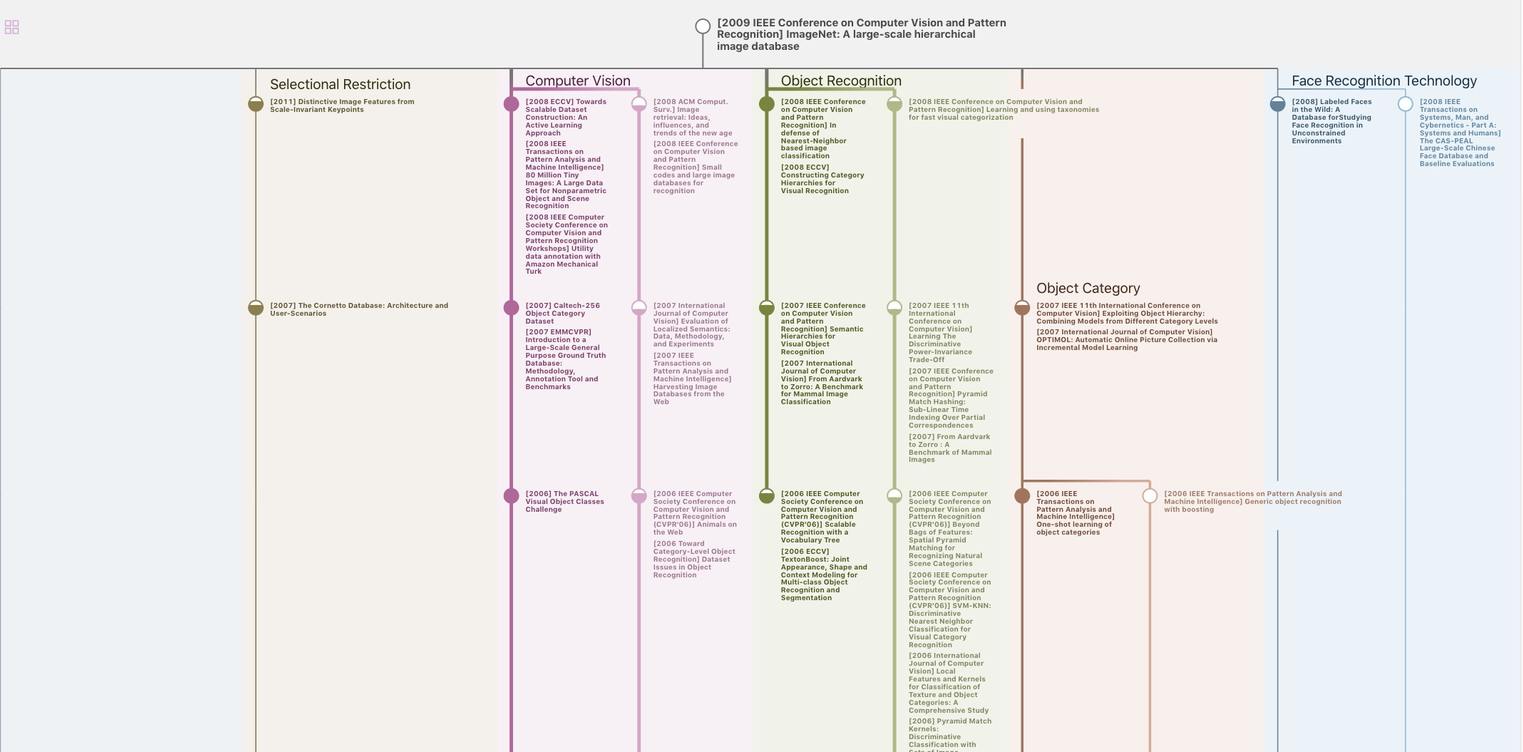
生成溯源树,研究论文发展脉络
Chat Paper
正在生成论文摘要