Towards Better Concordance Among Contextualized Evaluations In Fast-Gdm Problems
MATHEMATICS(2021)
摘要
A flexible attribute-set group decision-making (FAST-GDM) problem consists in finding the most suitable option(s) out of the options under consideration, with a general agreement among a heterogeneous group of experts who can focus on different attributes to evaluate those options. An open challenge in FAST-GDM problems is to design consensus reaching processes (CRPs) by which the participants can perform evaluations with a high level of consensus. To address this challenge, a novel algorithm for reaching consensus is proposed in this paper. By means of the algorithm, called FAST-CR-XMIS, a participant can reconsider his/her evaluations after studying the most influential samples that have been shared by others through contextualized evaluations. Since exchanging those samples may make participants' understandings more like each other, an increase of the level of consensus is expected. A simulation of a CRP where contextualized evaluations of newswire stories are characterized as augmented intuitionistic fuzzy sets (AIFS) shows how FAST-CR-XMIS can increase the level of consensus among the participants during the CRP.
更多查看译文
关键词
augmented intuitionistic fuzzy sets, contextualized evaluations, group decision-making, recurrent evaluations, consensus reaching process, computational intelligence, explainable artificial intelligence, explainable support vector machine classification
AI 理解论文
溯源树
样例
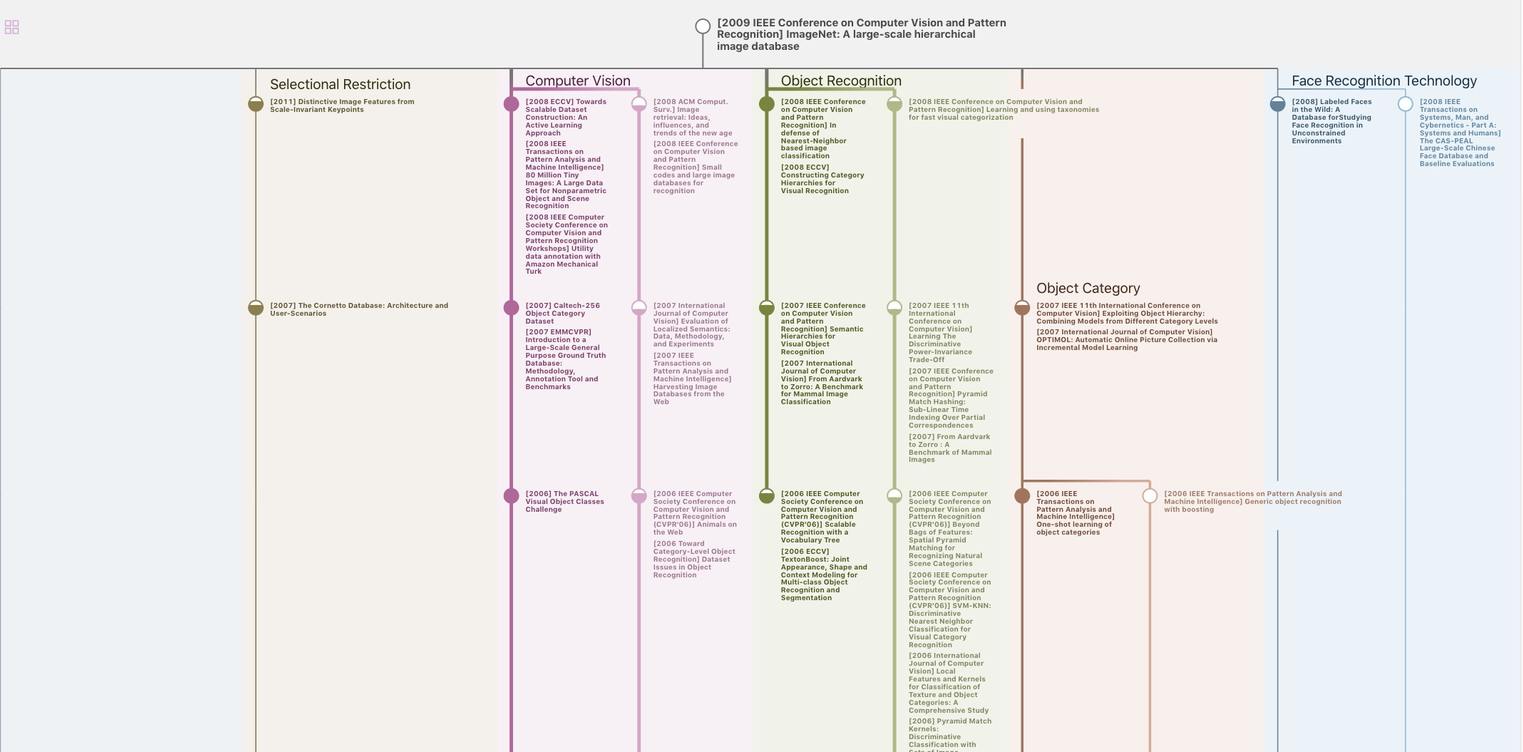
生成溯源树,研究论文发展脉络
Chat Paper
正在生成论文摘要