Molecular Fingerprint And Machine Learning To Accelerate Design Of High-Performance Homochiral Metal-Organic Frameworks
AICHE JOURNAL(2021)
摘要
Computational screening was employed to calculate the enantioseparation capabilities of 45 functionalized homochiral metal-organic frameworks (FHMOFs), and machine learning (ML) and molecular fingerprint (MF) techniques were used to find new FHMOFs with high performance. With increasing temperature, the enantioselectivities for (R,S)-1,3-dimethyl-1,2-propadiene are improved. The "glove effect" in the chiral pockets was proposed to explain the correlations between the steric effect of functional groups and performance of FHMOFs. Moreover, the neighborhood component analysis and RDKit/MACCS MFs show the highest predictive effect on enantioselectivities among the four ML classification algorithms with nine MFs that were tested. Based on the importance of MF, 85 new FHMOFs were designed, and a newly designed FHMOF, NO2-NHOH-FHMOF, with high similarity to the optimal MFs achieved improved chiral separation performance, with enantioselectivities of 85%. The design principles and new chiral pockets obtained by ML and MFs could facilitate the development of new materials for chiral separation.
更多查看译文
关键词
enantioseparation, machine learning, metal-organic framework, molecular fingerprint, molecular simulation
AI 理解论文
溯源树
样例
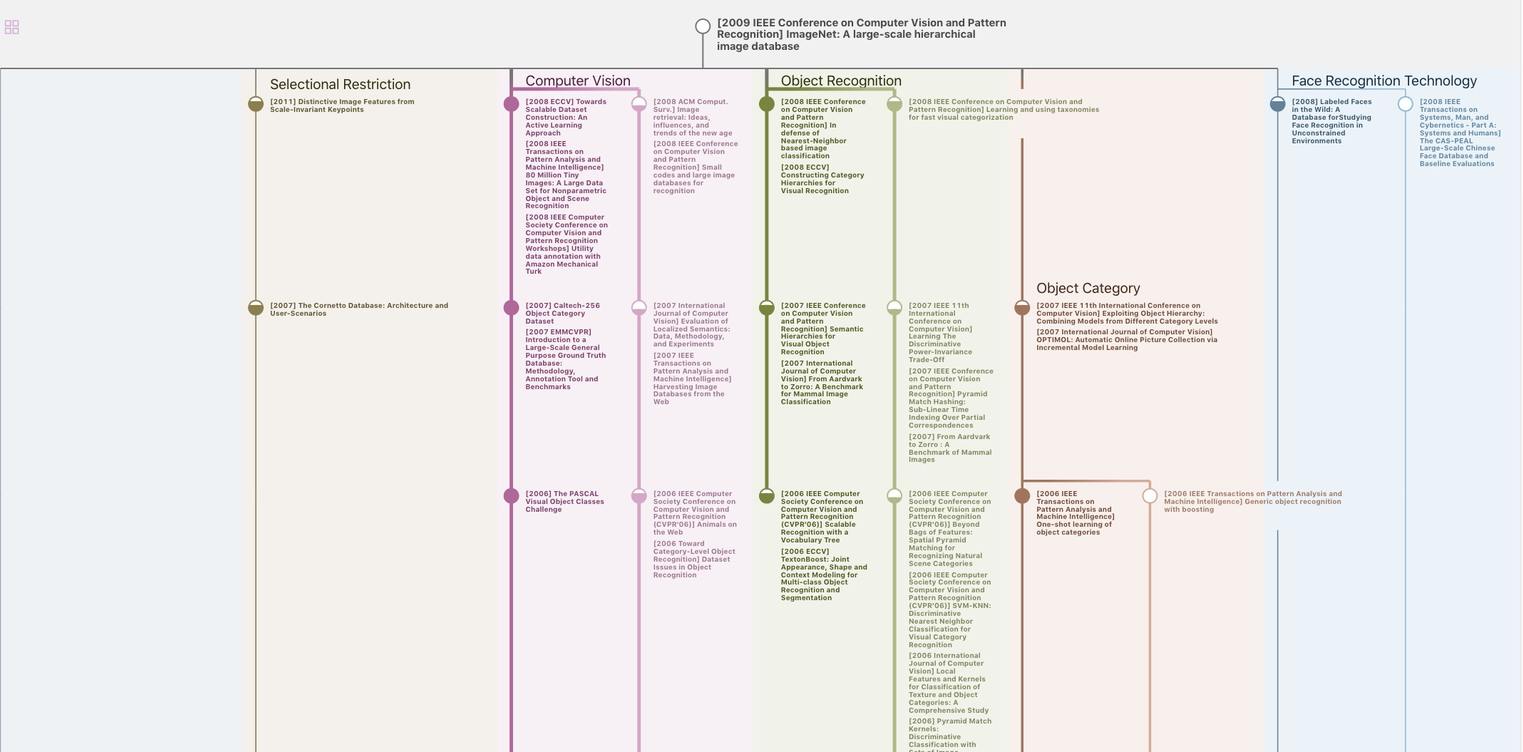
生成溯源树,研究论文发展脉络
Chat Paper
正在生成论文摘要