Higher-Order Conditional Random Field Established With Cnns For Video Object
KSII TRANSACTIONS ON INTERNET AND INFORMATION SYSTEMS(2021)
摘要
We perform the task of video object segmentation by incorporating a conditional random field (CRF) and convolutional neural networks (CNNs). Most methods employ a CRF to refine a coarse output from fully convolutional networks. Others treat the inference process of the CRF as a recurrent neural network and then combine CNNs and the CRF into an end-to-end model for video object segmentation. In contrast to these methods, we propose a novel higher-order CRF model to solve the problem of video object segmentation. Specifically, we use CNNs to establish a higher-order dependence among pixels, and this dependence can provide critical global information for a segmentation model to enhance the global consistency of segmentation. In general, the optimization of the higher-order energy is extremely difficult. To make the problem tractable, we decompose the higher-order energy into two parts by utilizing auxiliary variables and then solve it by using an iterative process. We conduct quantitative and qualitative analyses on multiple datasets, and the proposed method achieves competitive results.
更多查看译文
关键词
Video object segmentation, Conditional random field, Convolution Neural Networks, Higher-order potential
AI 理解论文
溯源树
样例
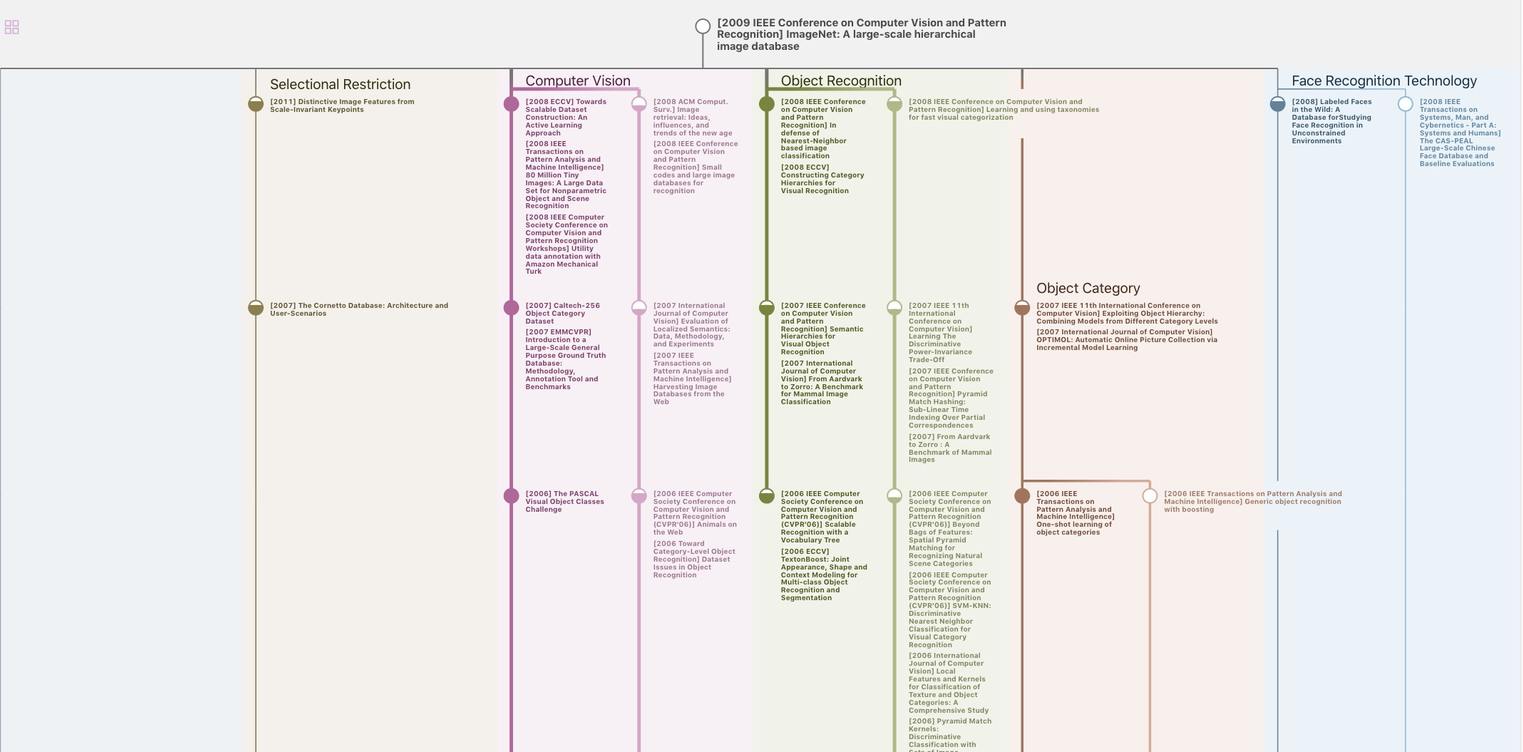
生成溯源树,研究论文发展脉络
Chat Paper
正在生成论文摘要