Improving Wetland Cover Classification Using Artificial Neural Networks With Ensemble Techniques
GISCIENCE & REMOTE SENSING(2021)
摘要
Wetland cover classification grows out of the need for management and protection for wetland sources to depict wetland landscapes. Exploring improved classification methods is important to derive good-quality wetland mapping products. This study investigates and applies two artificial neural network (ANN) based ensemble methods, namely, the MultiBoost artificial neural network (MBANN) and the rotation artificial neural network (RANN), for wetland cover classification, taking the Zoige wetland sited in the Qinghai-Tibet Plateau, China as the case area. The RANN trains and combines diverse ANNs by constructing a series of sparse rotation matrices, whereas the MBANN is developed from the sequential iteration in combination with the parallel sampling technique. Sixteen features related to wetland covers were extracted based on the digital elevation model data and Landsat 8 OLI images. The deep visual geometry group (VGG11) and random forests (RF) were implemented for comparison with our methods. The classification capability evaluation shows that our ensemble methods significantly improve the single ANN and outperform the VGG11 and RF. The RANN yields the highest overall accuracy (0.961), followed by the MBANN (0.942), VGG11 (0.934), RF (0.931), and ANN (0.916). We further concern and evaluate the classifier's robustness because it reflects the uniformity of classification capability. The RANN and the MBANN are insensitive to the reduction in data size, resistant to feature variability, and not influenced by data noise. Overall, the use of ensemble techniques can refine single ANN in classification capability and stability. The results from this study attest the important role of ensemble learning, which provides a promising scheme for wetland cover classification.
更多查看译文
关键词
Wetlands, classification, ensemble learning, artificial neural network, remote sensing
AI 理解论文
溯源树
样例
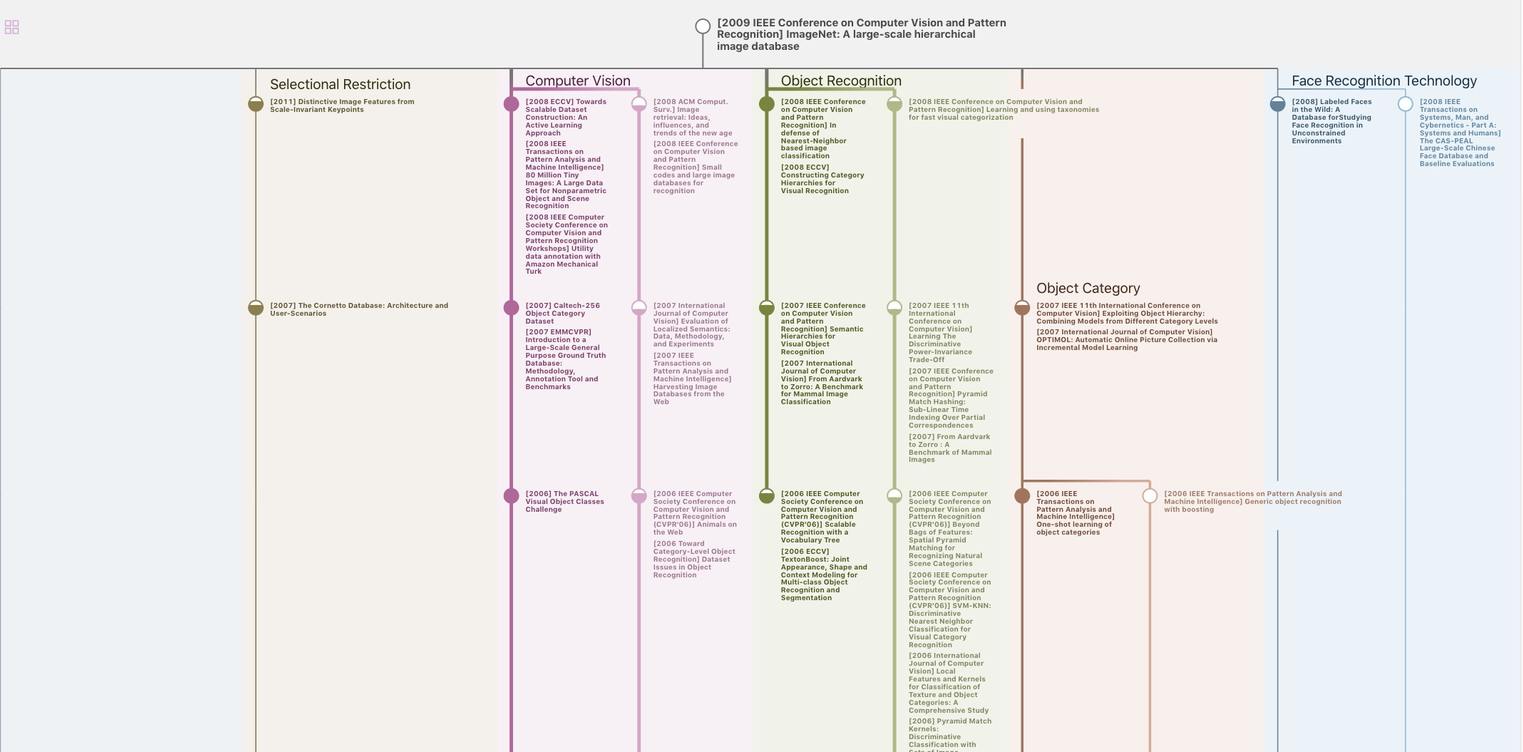
生成溯源树,研究论文发展脉络
Chat Paper
正在生成论文摘要