A Deep Learning-Based Fine Crack Segmentation Network On Full-Scale Steel Bridge Images With Complicated Backgrounds
IEEE ACCESS(2021)
摘要
Automatic defect detection of steel infrastructures in structural health monitoring (SHM) is still challenging because of complicated background, non-uniform illumination, irregular shapes and interference in images. Conventional defects detection mainly relies on manual inspection which is time-consuming and error-prone. In this study, a deep learning-based fine crack segmentation network, termed as FCS-Net was proposed in light of ResNet-50 and fully convolutional network (FCN). Structural modifications including Batch Normalization (BN) and Atrous Spatial Pyramid Pooling (ASPP) were made. In full-scale steel girder images with complicated background and fine foreground, the proposed FCS-Net achieves a MIoU of 0.7408, outperforming benchmark algorithms such as LinkNet, DeepLab V3, and CrackSegNet. Moreover, the ablation experiments were performed that justified the contribution and necessity of each modification.
更多查看译文
关键词
Feature extraction, Image segmentation, Convolution, Steel, Deep learning, Convolutional neural networks, Training, Deep learning, fine crack, complicated background, semantic segmentation
AI 理解论文
溯源树
样例
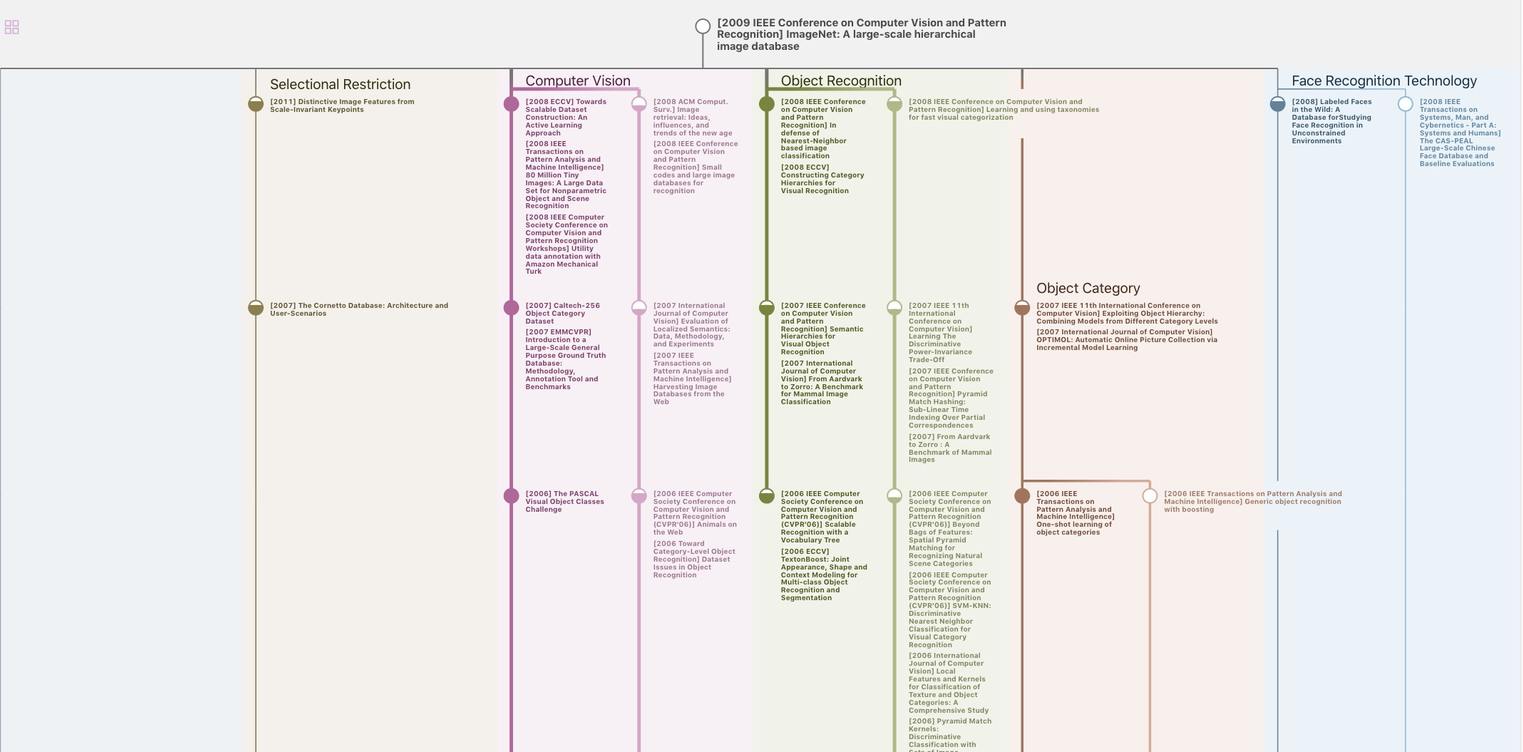
生成溯源树,研究论文发展脉络
Chat Paper
正在生成论文摘要