An Empirical Analysis Of Deep Learning Architectures For Vehicle Make And Model Recognition
IEEE ACCESS(2021)
摘要
Vehicle make and model recognition is an important component of the Intelligent Transport System (ITS), which plays an essential role in the vehicle surveillance and traffic monitoring. In this paper, we evaluated the performance of the recent deep neural networks for vehicle recognition to identify 196 different types of vehicles based on their make, model, and year using Stanford Cars data. Transfer learning has been employed to reduce the training time and also added a dropout of 0.5 at the last dense layer before the output layer for improved generalization performance on an applied dataset. Different augmentation methods such as random erasing, horizontal flip, random crop, resize, rotate, shear, and sharpen were applied to the training dataset to increase the number of images. Besides, optimization methods namely mixup and cyclical learning rate were also investigated to train these deep neural networks to avoid overfitting. Finally, the proposed ensemble learning framework using k-fold cross-validation has resulted in the improved accuracy of image classification for the vehicle make and model recognition. The final achieved accuracy is 93.96% through ensembling homogeneous models of DenseNet201, which is higher than previous state-of-the-art approaches for the vehicle make and model recognition. Simultaneously, it has also improved the performance of other models where MobileNetV2 has shown the highest improvement with an increase of 2.8% in the overall accuracy using the proposed ensemble learning technique.
更多查看译文
关键词
Neural networks, Computer architecture, Deep learning, Image recognition, Training, Degradation, Analytical models, Deep neural networks, vehicle make and model recognition, ensemble learning, k-fold cross-validation
AI 理解论文
溯源树
样例
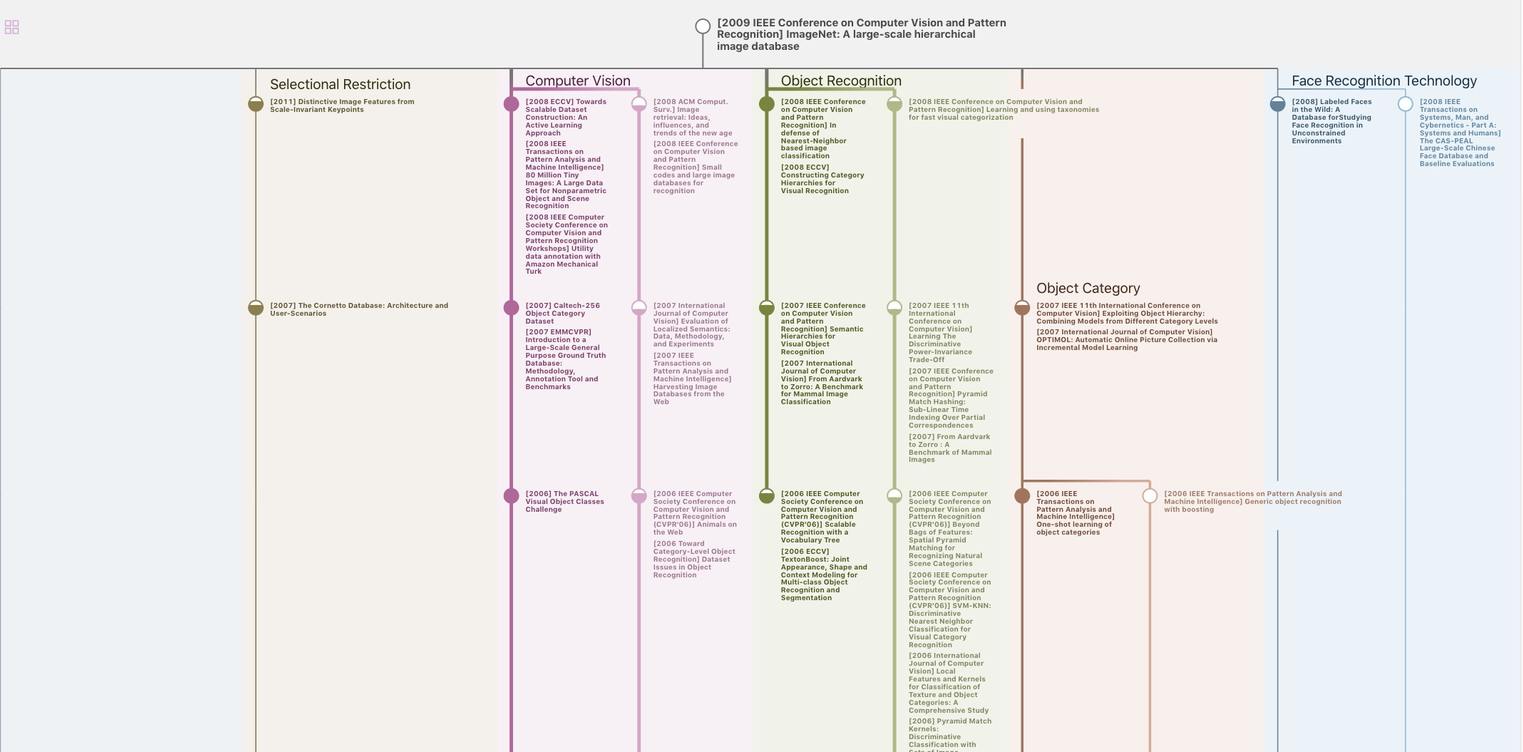
生成溯源树,研究论文发展脉络
Chat Paper
正在生成论文摘要