Interpretable entity meta-alignment in knowledge graphs using penalized regression: a case study in the biomedical domain
PROGRESS IN ARTIFICIAL INTELLIGENCE(2021)
摘要
In recent times, the use of knowledge graphs has been massively adopted so that many of these graphs can even be found publicly on the Web. This makes that solutions for solving interoperability problems among them might be in high demand. The reason is that unifying these knowledge graphs could impact a wide range of industrial and academic disciplines that can benefit from aspects such as the ability to configure queries that were not possible until now, for example, in the biomedical domain where there are significant problems of semantic interoperability. To date, several effective methods have been put forward to solve the heterogeneity problems in this knowledge ecosystem. However, it is not possible to assess their superiority in each different scenario they are facing. Therefore, we explore several penalized regression techniques that can mitigate the risk of incurring severe errors in real settings and preserve properties related to the interpretability of the solution. As a result, we have obtained a proposal for entity meta-alignment that yields promising results in the biomedical domain.
更多查看译文
关键词
Knowledge graphs, Knowledge engineering, Knowledge-based technology
AI 理解论文
溯源树
样例
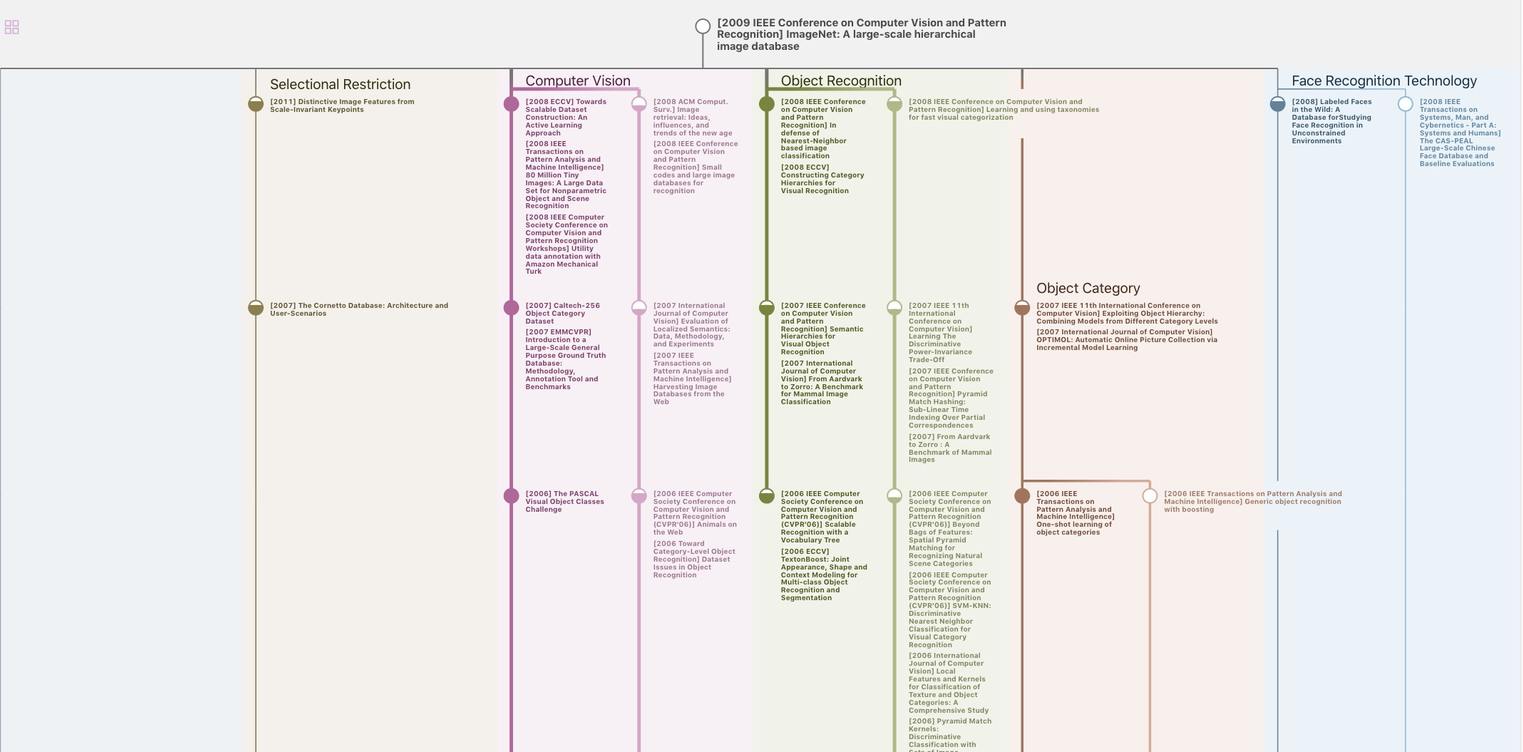
生成溯源树,研究论文发展脉络
Chat Paper
正在生成论文摘要