Multimodal Navigation-Affordance Matching For Slam
IEEE ROBOTICS AND AUTOMATION LETTERS(2021)
摘要
In robotics and mapping, prior knowledge of an environment can be included as virtual assets to a simultaneous localization and mapping (SLAM) solution. Borrowing the concept of affordances from robotic manipulation (i.e. virtual/interactive object models/primitives), this work addresses the fundamental duality in discrepancies between virtual and physical structures for localization and mapping. We propose a multimodal/non-Gaussian solution as a fundamental mechanism to leverage navigation-affordance assets during the localization and mapping process while simultaneously identifying any mismatches from the physical object. This allows the localization and mapping state-estimate more robust access to non-conventional and imperfect prior information about the environment, while computationally identifying assumed model discrepancies from imperfect sensor data. We use non-Gaussian factor graphs as modeling language to incorporate navigation-affordances with multi-sensor data similar to SLAM methods. We illustrate the approach with synthesized and real-world data from the construction industry where digital assets (such as drawings or models) are good proxies for how navigation-affordances can be generated and used in general.
更多查看译文
关键词
Autonomous vehicle navigation, SLAM, sensor fusion, probability and statistical methods, robotics and automation in construction
AI 理解论文
溯源树
样例
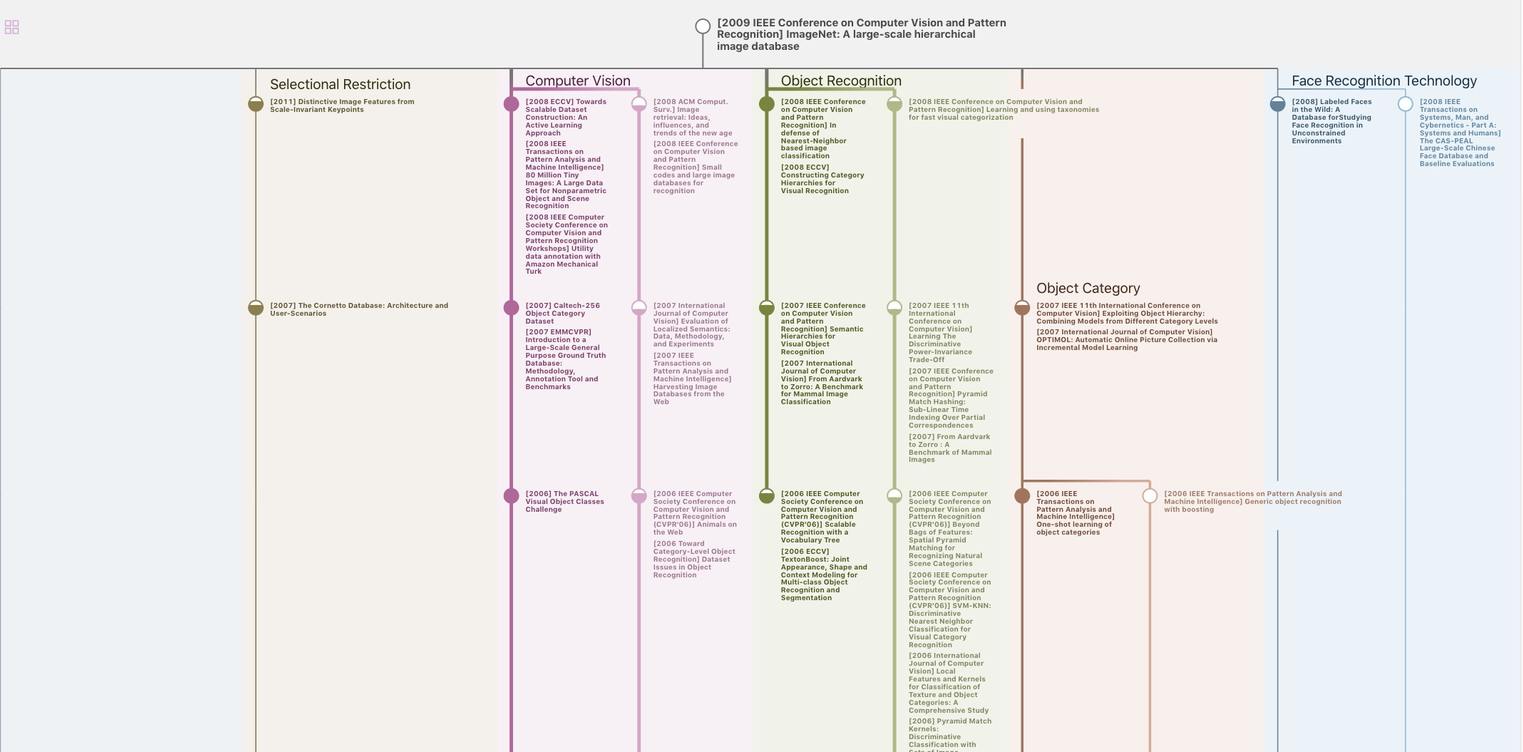
生成溯源树,研究论文发展脉络
Chat Paper
正在生成论文摘要